Agricultural Imports, Agriculture Productivity and Economic Growth in Sub-Saharan Africa
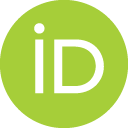
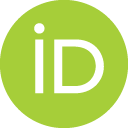
- DOI
- 10.2991/jat.k.200902.001How to use a DOI?
- Keywords
- Agricultural import; agriculture productivity; economic growth; Granger causality; Sub-Saharan Africa
- Abstract
This study investigates the causal links among agricultural imports, agriculture productivity, and economic growth in 40 sub-Saharan African countries over the period 1990–2015. Granger causality tests are applied to infer direction of causality, and the generalized two-stage least squares instrumental variable technique is employed to estimate the effects while controlling for endogeneity. The bootstrapping procedure is used to deal with cross-sectional dependence. The results reveal bidirectional causality between agricultural imports and agriculture productivity in the full sample, and in middle- and low-income non-oil-exporting countries. The relationship between agricultural imported inputs and agriculture productivity is positive and significant. In addition, unidirectional causality from agricultural imports to economic growth is observed in the full sample and middle-income non-oil exporters. The growth elasticity of agricultural imports is about 1.0 in the full sample and 1.3 in the middle-income group. These results then cast new light on the importance of agricultural imports for growth and development in the African region.
- Copyright
- © 2020 African Export-Import Bank. Publishing services by Atlantis Press International B.V.
- Open Access
- This is an open access article distributed under the CC BY-NC 4.0 license (http://creativecommons.org/licenses/by-nc/4.0/).
1. INTRODUCTION
Debate on agricultural trade can be traced back from the negotiations on agricultural trade under the General Agreement on Tariffs and Trade, which came into force in 1948. Later, significant progress toward agricultural trade reforms were covered and continues to be negotiated upon by the World Trade Organization Agreement on Agriculture, which came into force in 1995 as a result of the 1986–1994 Uruguay Round of world trade negotiations supported by the General Agreement on Tariffs and Trade (WTO, 2016). Since 1994, agricultural trade has been liberalized, which has led to increased globalization and integration. World Trade Organization members commit themselves to general agricultural trade rules pertaining to market access, domestic support, and export competition within well-defined timelines. The ultimate goal of these negotiations is to improve the livelihoods of farmers around the world through implementing sound agricultural trade regulations and domestic policies that promote competition and reduce distortions in agricultural trade (WTO, 2016). The research on agricultural trade and economic growth has therefore been gaining attention in the recent past both in developed and developing countries.
Most countries in sub-Saharan Africa (SSA) are agriculture-based, and agriculture accounts for about 32% of their Gross Domestic Product (GDP) on average (World Bank, 2008). In addition, more than 65% of the total population in the region is employed in the agriculture sector, although Africa in general has recently been experiencing rapid agricultural exits particularly in oil-producing countries (Headey et al., 2010). Nevertheless, Africa’s agriculture has the potential to reduce rural and urban poverty, which explains why boosting agriculture productivity remains a critical priority (Mozumdar, 2012). In SSA, the sector’s production remains relatively low compared with other regions in the world. Agriculture total factor productivity in SSA has been generally low and highly volatile, with an annual growth rate ranging between −0.05 and +0.05 for both low- and middle-income countries for the period 1962–2015 (USDA 2019). Stagnation in agriculture productivity in the region has resulted in low agricultural output and consequently low global share of Africa’s agricultural exports. Nevertheless, consumption demand has continued to grow because of the increase in population and income growth (Parker, 2011; Badiane et al., 2018). According to the Food and Agriculture Organization (2017), the value of food consumption exceeded the value of food production in SSA for the period 2000–2017.
The demand deficits are met by imports, which are becoming more challenging to finance because of the increasing international food commodity prices and poor performance of exports in the recent past. For example, over the period 2000–2010, Africa accounted for only about 2.8% of the world’s exports (UNCTAD, 2013). Between 1998 and 2013, the value of Africa’s agricultural imports grew fivefold whereas the value of agricultural exports tripled, leading to increased trade deficits (Badiane et al., 2018). According to Blein et al. (2013), over the period 2007–2011, 37 African countries were net importers of food, whereas 22 countries were net importers of agricultural raw materials. Furthermore, for the period 1960–2007, Africa in general was a net importer of food and other agricultural products despite her agricultural production potential (FAO, 2011). In SSA, the value of agricultural exports exceeded the value of imports for the period 1961–2005, indicating a favorable balance of agricultural trade. However, for the period 2005–2017, the region was a net importer of agricultural products.
The agricultural market in SSA is not homogeneous in the sense that each country presents some degree of heterogeneity in terms of imports demand and exports. A few countries are able to meet their import bills, whereas many still have difficulties (Manitra et al., 2012). Low yields and agriculture productivity coupled with increasing rates of population and economic growth among SSA and developing countries have been cited in the economic literature as the main factors behind the increasing demand for agricultural imports (Ikema, 1969; Gormely, 1991; Hilderink, et al., 2012; FAO, 2011; Parker, 2011; Manitra et al., 2012; Badiane et al., 2018).
The link between trade openness and growth has been widely studied, and the central debate is whether trade openness leads to economic growth or it is economic growth that drives trade openness. Most of the related studies have analyzed the causality between exports and economic growth, whereas a few focus on the causality between agricultural exports and economic growth. The relationship between imports and economic growth has not gained much attention despite the economic intuition that import supports growth. Theoretically, it has been argued that imports play a critical role in promoting economic growth through technological transfer and spillover effects, which in turn increase efficiency and productivity. Imports expose domestic firms to foreign competition, thus triggering their response to technology advancement (Haddad et al. 1996; Lawrence and Weinstein, 1999). Consequently, consumption gains resulting from a wide variety of commodities made available at competitive prices lead to welfare improvement. Furthermore, several studies (Lawrence and Weinstein, 1999; Awokuse, 2007; Awokuse, 2008) have tested and supported the import-led growth hypothesis that imports support economic growth. In SSA, Fosu (2001) reports that import instability affects economic growth negatively; thus, stability of imports flow is crucial for growth in the region.
The present study seeks to investigate whether any causality exists between agricultural imports and GDP per capita growth, and between agricultural imports and agriculture productivity, of different country groups in SSA, using a framework design that accounts for cross-section dependence and slope heterogeneity. We focus on the agriculture sector because of its significance in the region. The research is further prompted by the increasing imports of food and agricultural products in SSA. The analysis is based on World Bank’s nonoverlapping classification of countries, that is, oil exporters, middle-income non-oil exporters and low-income non-oil exporters, and applies bootstrapped Granger causality tests in heterogeneous panels. In order to gain more insight on the effect of variables of interest, the analysis is extended by applying the Instrumental Variable (IV) estimation with Generalized Two-stage Least Squares (G2SLS) for panel-data models to control for endogeneity.
The results reveal a bidirectional causality between agriculture productivity and agricultural imports in the full sample, and in middle- and low-income non-oil-exporting countries. However, in oil-exporting countries no causality is observed between agriculture productivity and agricultural imports. The relationship between agriculture productivity and agricultural imports is positive and significant. There is no evidence of unidirectional causality running from GDP per capita growth to agricultural imports in the whole sample or in the subsamples. However, evidence of unidirectional causality running from agricultural imports to GDP per capita growth is recorded in the full sample and in middle-income non-oil-exporting countries. The growth elasticity of agricultural imports is approximately 1.0 in the full sample and 1.3 in middle-income non-oil-exporting countries. The results imply that agricultural import-led growth hypothesis is supported in SSA. The findings of this study, therefore, contribute to existing international trade literature; they counter the notion that imports reduce economic growth via import leakages and crowding out domestic production, by showing that agricultural imports positively and significantly affect agriculture productivity and GDP per capita growth in SSA. Imports are either consumed or invested; hence import of agricultural consumer goods and capital goods play an important role in influencing technology absorption and innovation in the domestic market in order to keep pace with international competition. Technology transfer increases production efficiency, output, and consequently growth of the economy.
The rest of this paper is composed of the following sections. Section 2 reviews the relevant literature and highlights some empirical evidence on the relationship between trade openness, productivity, and economic growth. Description of the data used in the analysis is provided in Section 3, whereas Section 4 explains the process of empirical analysis. Section 5 presents the empirical results, discussion of the results, and robustness check. Finally, the conclusions of the study are provided in Section 6.
2. LITERATURE REVIEW: TRADE OPENNESS, PRODUCTIVITY, AND ECONOMIC GROWTH NEXUS
In the international trade literature, several studies have attempted to identify the relationship and the direction of causality between trade openness (measured by the ratio of imports plus exports to GDP, ratio of imports to GDP or ratio of export to GDP) and economic growth. Some of these studies have applied the conventional regression analysis, which only captures the effect of trade openness on economic growth. Most of the studies find that trade openness significantly affects economic growth positively (Sala-i-Martin, 1997; Frankel and Romer, 1999; Dollar and Kraay, 2002; Yanikkaya, 2003; Nicita et al., 2014; Were, 2015; Zahonogo, 2016; Abreha, 2019). Other studies use Granger causality or error correction models to identify the direction of causality between trade openness and economic growth variables. These studies provide mixed results. For example, Ahmad and Kwan (1991) apply Granger causality in 47 African countries and find no causality between exports and growth. Bbaale and Mutenyo (2011) analyze exports and economic growth in 35 SSA countries and find that agricultural exports and capital goods imports significantly affect per capita income growth. Fatma and Ayse (2013) apply the Granger causality test to investigate causality between trade openness and growth in G7 countries over the period 1970–2011. They find positive bidirectional causality. Thomas and Margarete (2012) analyze the relationship between GDP per capita and trade openness for 158 countries over the period 1970–2009 using error correction models. They find positive significant bidirectional causality.
On imports and productivity, Lee (1995) finds that imported capital goods inputs increased efficiency and long-run growth in developing countries. Sangho et al. (2007) use Korean data for the period 1980–2003 to investigate the link between imports and productivity growth. They find positive and significant effects of imports. Uslu (2016) studies the causal link between imports and growth in Turkey for the period 1998–2014 and finds positive causality running from imports to GDP growth. Guntukula (2018) reports a bidirectional causality between imports and economic growth in India. Abreha (2019) investigated the causal relationship between imports and firm productivity in Ethiopia and recorded productivity gains from imports. Aluko and Adeyeye (2020) use Granger causality to test causality between imports and economic growth in Africa. They report unidirectional causality running from imports to economic growth in some countries, whereas in other countries they find unidirectional causality running from economic growth to imports. In most of the countries under study, they conclude that there is no causality between imports and economic growth either in the short or long run.
Few studies have investigated the effect of agricultural trade on economic growth. Sanjuán‐López and Dawson (2010) use Granger causality tests and report a long-run positive relationship between agricultural exports and economic growth in developing countries. In Africa, Ouma et al. (2016) study the relationship between agricultural trade and economic growth in the East Africa community using vector autoregressive and vector error correction models. They find bidirectional causality between agricultural exports and economic growth in Kenya, unidirectional causality in Rwanda, and no causality in the case of Tanzania, Uganda, and Burundi. Nahanga and Bečvářová (2016) use Granger causality and impulse response in their analysis and report that agricultural exports support economic growth in Nigeria. Bakari and Mohamed (2018) investigate the effect of agricultural trade on economic growth of North Africa. They report that agricultural exports have a positive effect on GDP, but agricultural imports have no effect on economic growth. Most of these studies investigate the contribution of agricultural exports to economic growth, thereby overlooking the effect of agricultural imports.
3. DATA
This study uses panel data for 40 SSA countries for the period 1990–2015. Countries and time span are selected based on data availability. The list of countries in the sample is presented in Appendix Table A1. Data are obtained mainly from the Food and Agriculture Organization Corporate Statistical Database, World Bank, and International Monetary Fund. Agricultural imports are measured by the total value of aggregated agricultural products in US$. Economic growth is measured using GDP per capita growth, whereas agricultural productivity is measured by the value of agricultural production per hectare of agricultural land following Frisvold and Ingram (1995). Table 1 presents a summary of the variables description and data sources, and Appendix Table A2 presents the descriptive statistics of these variables.
Variables | Code | Definition | Data source |
---|---|---|---|
Agricultural imports | agricM | Total value of agricultural imports in US$ | FAOSTAT |
Agriculture output | agricQ | Total value in international $constant (2004–2006) | FAOSTAT |
Agricultural land | agricL | Rain fed, irrigated cropland and permanent pasture in hectares | FAOSTAT |
Agriculture productivity | agricvperha | Total agricultural production value per hectare of agricultural land | Computed using FAOSTAT data |
Economic growth | GDPpc growth | Annual percentage growth rate of GDP per capita based on constant 2010 US$ | World Bank |
Investment | grosskform | Gross capital formation as % of GDP | World Bank and OECD |
Human capital | schenroll | Secondary School enrollment as % of gross enrollment | UNESCO |
Population growth | popgrowth | Annual population growth rate in % | World Bank |
Inflation | inflation | Annual % changes in the consumer price index | International Monetary Fund |
Imported inputs | importedI | Total value of imported agricultural inputs in US$ | Calculated from Eora26 IO tables |
Agricultural labor | agricLbr | Number of economically active adults in agriculture | FAOSTAT |
Agricultural capital | agricK | The total stock of farm machinery | FAOSTAT |
Fertilizer | Fert | Metric tons of N, P2O5, K2O fertilizer consumption | International Fertilizer Association and FAO |
Food imports | foodM | Food imports (% of merchandise imports) | World Bank |
Notes: FAO, Food and Agricultural Organisation; FAOSTAT, Food and Agriculture Organization Corporate Statistical Database; GDP, gross domestic product; OECD, Organisation for Economic Co-operation and Development; UNESCO, United Nations Educational, Scientific and Cultural Organization; IO, input-output.
Variable description and data sources
Other variables included in the growth model are population growth, inflation, and investment. Secondary school enrollment captures the level of human capital. Data on imported agricultural inputs was computed from Eurostat manual of supply, use and input–output tables, specifically Eora26. Eora26 is a model in which all countries have been aggregated to a 26-common sector classification and the supply–use tables from the full Eora MRIO are converted to symmetric industry-by-industry input–output tables using the industry technology assumption (Lenzen et al., 2012, 2013). In order to gain more insights, the sample is divided into three nonoverlapping subsamples based on the World Bank country classification—that is, oil exporters, middle-income non-oil exporters, and low-income non-oil exporters. These groups of countries are assumed to exhibit different degrees of heterogeneity in agricultural trade.
4. METHODOLOGY
4.1. Preliminary Analysis: Cross-sectional Dependency, Unit Root, and Panel Cointegration
Hashem Pesaran (2006) emphasizes the importance of accounting for cross-sectional dependence in panel data in order to avoid biased results. Testing cross-sectional correlation of errors in panel data is advisable because the shock emanating from one country is likely to be transmitted to other countries as a result of the high degree of cross-border trade and/or financial integration accelerated by globalization (Kar et al., 2011; Menyah et al., 2014; Chang et al., 2014). In addition, countries in the sample differ on the degree of imports dependency and levels of growth; hence the assumption of slope homogeneity in the analysis may not hold. Therefore, the analysis starts by testing data for cross-sectional dependence and slope homogeneity. Cross-sectional dependence is tested using Hashem Pesaran (2004) cross-sectional dependency test (CD) and Breusch–Pagan Lagrange multiplier (LM) test of independence. Hashem Pesaran (2004) shows that the test is robust for panels, where time (T) tends to infinity, and then where the number of observations of the cross-section (N) tends to infinity, or both. The CD test statistic is computed as follows:
The Granger causality test applied in this study assumes that the variables are stationary. In line with this, the cross-sectional augmented Im, Pesaran and Shin (CIPS) test proposed by Hashem Pesaran (2007) is applied. The CIPS test assumes cross-sectional dependency and is valid for series with different lags and unbalanced panels. The null hypothesis—that is, all panels contain unit root—is tested against the alternative hypothesis—that is, some panels are stationary.
The widely used panel cointegration tests in the economic literature proposed by Pedroni (1999, 2004) and the Westerlund (2007) Error Correction Based (Westerlund ECB, hereafter) were applied to test cointegration. Both tests allow for a large degree of heterogeneity and account for cross-sectional dependence within and across the cross-sectional units. In both tests, the null hypothesis of no cointegration is tested against the alternative hypothesis that all panels are cointegrated.
4.2. Panel Granger Causality Test
In order to determine the direction of causality, this study adopts Dumitrescu and Hurlin’s (2012) procedure for testing for Granger causality in heterogeneous panel data, and uses Stata user-written command developed by Lopez and Weber (2017). This is the most recent test developed in the economic literature. It requires a balanced panel and allows for the selection of the optimal number of lags. The procedure involves the estimation of the following equation:
The alternative hypothesis that there can be causality in some panels but not necessarily all is defined as:
F-test of the P linear hypothesis λi1 = ⋯ = λiP = 0 is conducted obtaining the Wald statistic Wi for each individual panel. The average Wald test statistic
The decision to reject or not to reject the null hypothesis is based on the values of the test statistics: Z bar (
In the case of a panel dataset where, T > 5 + 3P, the standardized Z statistic, that is,
Furthermore, the study estimates regressions for each individual country to obtain more insights on the coefficients of the regressions in which the tests are based. In the case of cross-section dependence, Dumitrescu and Hurlin (2012) propose a procedure to compute the bootstrapped critical values of
In testing for Granger causality, the null hypothesis is therefore rejected if
5. RESULTS AND DISCUSSION
As previously stated, the Granger causality test procedure developed by Dumitrescu and Hurlin (2012) requires balanced panel data and assumes the variables are stationary. In addition, cross-section dependence is a problem that requires consideration particularly in panel data with long time series (Baltagi, 2008). Preliminary test results for cross-sectional dependence and slope homogeneity presented in Table 2 show that the p-values in both tests are zero; hence, the null hypothesis of cross-sectional independence and slope homogeneity are rejected. These results, therefore, justified the application of the bootstrapping procedure.
Test | Statistic | p-value |
---|---|---|
Cross-sectional dependency H0: cross-section independence | ||
Hashem Pesaran (2004) CD | 29.403 | 0.000 |
Breusch–Pagan LM | 7328.705 | 0.000 |
Slope homogeneity H0: slope homogeneity | ||
Delta | 24.488 | 0.000 |
Delta adj. | 26.621 | 0.000 |
The data used for these tests cover the full sample period 1990 to 2015.
Cross-sectional dependency and homogeneity tests resultsa
The results for unit root test proposed by Hashem Pesaran (2007) that assumes cross-section dependence are presented in Table 3. They show that, for all variables, some panels are stationary at level, and at first difference both without trend and with trend. Hence, the data were appropriate for testing causality.
Variables | Lags | Without trend | With trend | ||
---|---|---|---|---|---|
Z-bar statistic | p-value | Z-bar statistic | p-value | ||
agricM | 0 | −11.055 | 0.000 | −9.737 | 0.000 |
1 | −5.565 | 0.000 | −3.905 | 0.000 | |
agricvperha | 0 | −6.223 | 0.000 | −5.045 | 0.000 |
1 | −2.164 | 0.015 | −2.224 | 0.013 | |
GDPpcgrowth | 0 | −18.528 | 0.000 | −16.306 | 0.003 |
1 | −10.151 | 0.000 | −7.790 | 0.000 |
The data used for these tests cover the full sample period from 1990 to 2015. H0: All panels contain unit root. H1: Some panels are stationary.
CIPS panel unit root tests resultsa
Table 4 presents the results for both Pedroni (1999, 2004) and Westerlund ECB panel cointegration tests. Three models are used to run the tests where, in each case, one variable is treated as the dependent variable and others as independent variables. For example, in the first model, agricultural imports are treated as the dependent variable whereas GDP per capita growth and agriculture productivity (agricvperha) are treated as independent variables. The results of the Pedroni test provide strong evidence for rejecting the null hypothesis of no cointegration in the three models, particularly in model 2, while Westerlund ECB test results show strong evidence for rejecting the null hypothesis of no cointegration in the second model but not in the first or third models. Thus, in the case of the second model where GDP per capita growth is treated as a function of agricultural imports and agriculture productivity, the null hypothesis of no cointegration is strongly rejected by both tests. These results indicate that the variables have long-run relationships particularly with per capita GDP growth and, hence, the study proceeds on estimating the causal relationships.
Pedroni panel cointegration test | Westerlund ECB panel cointegration test | |||||
---|---|---|---|---|---|---|
Panel statistics | Group-mean statistics | Value | Z-value | Robust p-value | ||
Model 1 (dependent variable –agricM) | ||||||
v | −2.768*** | Gt | −0.776 | 8.644 | 1.000 | |
Rho | 0.4313 | 0.3946 | Ga | −2.374 | 6.807 | 1.000 |
t | −1.948*** | −3.196*** | Pt | −5.682 | 4.990 | 0.790 |
adf | −0.4065 | −0.2176 | Pa | −1.885 | 4.502 | 0.860 |
Model 2 (dependent variable –GDPpc growth) | ||||||
v | 3.463*** | Gt | −2.897 | −5.919 | 0.000 | |
Rho | −12.45*** | −10.55*** | Ga | −9.560 | −0.439 | 0.000 |
t | −20.73*** | −26.26*** | Pt | −15.899 | −4.905 | 0.000 |
adf | −14.03*** | −13.72*** | Pa | −8.904 | −3.438 | 0.000 |
Model 3 (dependent variable –agricvperha) | ||||||
v | 0.9496 | Gt | −2.353 | 1.322 | 0.340 | |
Rho | −3.246*** | −1.853** | Ga | −6.697 | 5.983 | 0.570 |
t | −6.114*** | −6.857*** | Pt | −7.362 | 7.877 | 0.980 |
adf | −3.274*** | −2.684*** | Pa | −3.583 | 6.460 | 0.930 |
The data used for these tests cover the full sample period from 1990 to 2015. The null hypothesis in both tests is no cointegration. For the Pedroni test,
p < 0.1,
p < 0.05, and
p < 0.01 (Pedroni 1999, 2004). For Westerlund ECB test, Lag 1 and lead 1 are selected based on AIC (Westerlund 2007), the test is done with constant and no trend, Bartlett kernel window length formula, selected based on 4(T/100)2/9 ≈ 3 (Wooldridge 2013). p-values are calculated by repeating the bootstrap 100 times.
Gt, group t-statistic; Ga, group ADF; adf, augmented Dickey–Fuller test statistic; Pt, panel t-statistic; Pa, panel ADF.
Panel cointegration test resultsa
In testing causality on panel data, the Dumitrescu and Hurlin (2012) procedure allows for the selection of the number of lags to be included. Lag 1 is selected automatically in this study based on the minimum Akaike, Bayesian, or the Hannan–Quinn information criterion. This eliminates the bias associated with selection of too few lags or too many lags (Menyah et al., 2014). Table 5 presents the results of panel causality analysis between agriculture productivity and agricultural imports, and between economic growth (measured by GDP per capita growth) and agricultural imports.
|
|
95% critical value | |
---|---|---|---|
H0: Agriculture productivity do not Granger-cause agricultural imports | |||
Full sample | 3.8433 | 10.326** | 6.376 |
Oil exporters | 3.794 | 5.589 | 6.287 |
Middle-income non-oil exporters | 3.845 | 6.969** | 6.379 |
Low-income non-oil exporters | 3.862 | 9.050** | 5.271 |
H0: Agricultural imports do not Granger-cause agriculture productivity | |||
Full sample | 3.631 | 9.526*** | 6.734 |
Oil exporters | 1.071 | 0.141 | 5.789 |
Middle-income non-oil exporters | 4.584 | 8.778*** | 6.257 |
Low-income non-oil exporters | 4.083 | 9.950*** | 6.065 |
H0: GDP per capita growth do not Granger-cause agricultural imports | |||
Full sample | 1.017 | −0.312 | 2.321 |
Oil exporters | 0.731 | −0.538 | 2.884 |
Middle-income non-oil exporters | 2.022 | 2.504 | 3.455 |
Low-income non-oil exporters | 0.537 | −1.463 | 2.669 |
H0: Agricultural imports do not Granger-cause GDP per capita growth | |||
Full sample | 1.773 | 3.532** | 3.418 |
Oil exporters | 0.712 | −0.576 | 3.926 |
Middle-income non-oil exporters | 3.697 | 6.605*** | 3.850 |
Low-income non-oil exporters | 1.165 | 0.521 | 3.333 |
The data used cover the full sample period 1990–2015.
p < 0.1,
p < 0.05, and
p < 0.01. Bootstrap procedure is repeated 1000 times. In the whole sample the test statistic favored is
Results summary of panel Granger causality testa
Table 5 shows strong evidence of bidirectional causality between agriculture productivity and agricultural imports in the whole sample. The
Causality analysis between economic growth and agricultural imports shows no evidence of causality running from GDP per capita growth to agricultural imports in any of the samples. The result implies that economic growth does not significantly cause agricultural imports in SSA. These results contrast with some claims in the existing economic literature that increased imports of agricultural products in the region would result from increased economic growth (Parker, 2011; Hilderink, et al., 2012; Badiane et al., 2018). There is strong evidence of unidirectional causality running from agricultural imports to GDP per capita growth in the full sample and in the middle-income non-oil exporter group. These results support the agricultural import-led growth hypothesis which suggests that imports growth has a positive and significant effect on economic growth. The results are in line with the findings of Uslu (2016) and Aluko and Adeyeye (2020) that imports cause GDP growth in certain countries. Nonetheless, as also shown in Table 5, agricultural imports do not Granger-cause GDP per capita growth in oil exporter or low-income non-oil exporter subsamples.
5.1. Robustness Check on Causality: Country Evidence
For robustness check, the causality analysis is repeated by country level for all countries under study (see Appendix Tables A3 and A4). The results indicate unidirectional causality from agriculture productivity to agricultural imports in the case of Angola, Kenya, Malawi, Nigeria, Rwanda, and Somali. A unidirectional causality running from agricultural imports to agriculture productivity is evident in the case of Burundi, Mozambique, Senegal, Central Africa, Mauritius, and Gambia. In addition, a strong evidence of bidirectional causality between agriculture productivity and agricultural imports is evident in the case of Zambia. Causality analysis between GDP per capita growth and agricultural imports reveals a weak bidirectional causality between GDP per capita growth and agricultural imports in the case of only two countries—Cote d’Ivoire and Ghana—out of the 40 countries in the sample. Unidirectional causality running from agricultural imports to GDP per capita growth is found in the case of five countries: Cote d’Ivoire, Ghana, Kenya, Sao Tome, and Cape Verde, which are all classified as middle-income non-oil exporter countries. There is no evidence of unidirectional causality running from GDP per capita growth to agricultural imports in any of the countries under study.2 These findings support the main results of the subsamples presented in Table 5.
5.2. Control for Endogeneity: Effect of Agricultural Imports on Economic Growth
Having established that a feedback effect exists between agriculture productivity and agricultural imports, and that agricultural imports cause economic growth in some countries, we next examine the effect of agricultural imports on economic growth while controlling for endogeneity. In this section, the IV technique is used to address endogeneity. The following growth model is estimated:
Variables | (1) | (2) | (3) | (4) | (5) |
---|---|---|---|---|---|
lnGDPpc growth | Full panel | Full panel | Oil exporters | Middle income | Low income |
lnagricM | −0.0395 (0.0676) | 0.977** (0.573) | −0.123 (0.0876) | 1.300** (0.601) | −0.000717 (0.149) |
lngrosskform | 0.457*** (0.121) | 0.149* (0.231) | −0.160 (0.266) | 0.0887* (0.477) | 0.444* (0.227) |
lnschenroll | −0.146 (0.108) | −1.495* (0.710) | −0.297 (0.198) | −1.651** (0.800) | −0.249 (0.173) |
lnpopgrowth | −0.273*** (0.0930) | −0.215 (0.170) | −0.220 (0.316) | −0.184 (0.232) | −0.415** (0.203) |
inflation | 0.000312 (0.000219) | 0.0003*** (0.0001) | 0.000327 (0.000215) | 0.0112* (0.006) | −0.00517 (0.00801) |
Constant | 0.646 (0.701) | −6.556 (4.383) | 4.278*** (1.367) | −9.758** (4.56) | 0.635 (1.300) |
Hausman test (χ2) | 18.41** | 0.27 | 18.48*** | 1.64 | |
Over identification test (Sargan–Hansen statistic) | 0.000 | 0.000 | 0.000 | 0.000 | 0.000 |
Under identification test (Anderson canon. corr. LM statistic) | 31.589*** | 33.196*** | |||
Weak identification test (Cragg–Donald Wald F-statistic) | 33.041*** | 38.42*** | 38.808*** | 20.92*** | |
Anderson–Rubin (AR) Wald test | 6.52** | 1.03 | 17.53*** | 0.91 | |
Observations | 819 | 819 | 168 | 231 | 420 |
Number of country | 39 | 39 | 8 | 11 | 20 |
Notes:
Standard errors are in parentheses.
p < 0.1;
p < 0.05;
p < 0.01.
Column 1: RE model results, columns 2–5: FE model results.
Regression results for agricultural imports on economic growth (2SLS-IV estimation)a
As presented in Table 6, the Sargan–Hansen test statistic is equal to zero, which implies that the model is exactly identified. The Anderson canon corr. LM statistic is significant at the 1% level, implying that the null hypothesis that the model is underidentified is rejected. Furthermore, the F-statistic for Anderson–Rubin (AR) Wald joint test for relevance and exogeneity developed by Anderson and Rubin (1949, 1950) is significant at the 5% level in the full sample and in the middle-income group. This suggests that the instrument used is valid—that is, it is significantly correlated with agricultural imports and is uncorrelated with the error term; hence it does not affect the dependent variable through other channels other than the regressor of interest. The AR joint test is robust to weak instruments. In addition, weakly identified instruments could lead to biased results. Hence, the Cragg–Donald (1993) Wald F-test is applied to test for weak identification. The results satisfy the rule of thumb (F-statistic is greater than 10) in all subsamples; hence the instrument used is not weak.
Column 1 in Table 6 shows the 2SLS-IV estimation results of the RE for the full panel. The coefficient of agricultural imports is negative though not significant. However, on applying 2SLS-IV FE with robust standard errors (the preferred model given the Hausman test), the coefficient becomes positive and significant at the 0.05 level (column 2 in Table 6). This suggests that agricultural imports positively and significantly influence economic growth. The growth elasticity of agricultural imports is approximately 1.0. This relationship is replicated in the middle-income non-oil-exporting countries, where the coefficient of the logarithm of agricultural imports is positive and significant at the 0.05 level (column 4 in Table 6). The growth elasticity of agricultural imports in this subsample is 1.3. However, the relationship between agricultural imports and GDP per capita growth in the oil exporters and low-income non-oil exporters subsamples is negative though not significant (columns 3 and 5 in Table 6). Hence, agricultural imports do not significantly affect economic growth in these subsamples. In conclusion, these empirical results are consistent with the results of the Granger causality presented in Table 5.
5.3. Effect of Imported Agricultural Inputs on Agriculture Productivity
As a result of increasing globalization, agricultural inputs are either imported or produced domestically. We examine the effect of imported agricultural inputs on agriculture productivity as a potential channel through which agricultural imports promote economic growth. Table 7 presents the empirical results of the effect of imported agricultural inputs on agriculture output. The results reveal that for all the samples, except the low-income group, the coefficient of imported inputs is positive and significant at the 0.01 level. The output elasticity of imported agricultural inputs is approximately 0.1. The coefficients of the control variables, which include agricultural land, agricultural labor, agricultural capital, and fertilizer, are positive as expected and are statistically significant. Furthermore, when food imports were added to the model, the significance and magnitude of the coefficients of imported agricultural inputs and the control variables remained almost the same for the full sample (column 2 in Table 7). Nevertheless, food imports have a negative and significant impact on agriculture output in the full sample. This negative coefficient is also reported in the oil exporter and low-income non-oil exporter groups, though only that for the latter group is significant. In the middle-income non-oil exporter group, the coefficient is positive, though insignificant.
Variables | (1) | (2) | (3) | (4) | (5) |
---|---|---|---|---|---|
lnagricQ | Full panel | Full panel | Oil exporters | Middle income | Low income |
ImportedI | 0.105*** (0.0128) | 0.102*** (0.0131) | 0.111*** (0.0192) | 0.103*** (0.0233) | 0.0316 (0.0258) |
lnagricL | 0.746*** (0.0372) | 0.745*** (0.0379) | 0.904*** (0.0724) | 0.382*** (0.0789) | 0.818*** (0.0551) |
lnagricLbr | 0.228*** (0.0485) | 0.243*** (0.0500) | 0.384*** (0.111) | 0.208** (0.0836) | 0.435*** (0.0974) |
lnagricK | 0.0644*** (0.0147) | 0.0677*** (0.0150) | 0.0165 (0.0172) | 0.161*** (0.0322) | 0.0468* (0.0258) |
lnfert | 0.0234*** (0.00764) | 0.0220*** (0.00786) | 0.0122 (0.0129) | 0.0783*** (0.0166) | 0.00737 (0.0112) |
foodM | −0.00215* (0.00115) | −0.000631 (0.00174) | 0.00524 (0.00357) | −0.00294** (0.00149) | |
Constant | 4.121*** (0.270) | 4.082*** (0.288) | 2.070*** (0.600) | 5.686*** (0.590) | 3.494*** (0.501) |
Observations | 819 | 819 | 168 | 231 | 420 |
Number of countries | 39 | 39 | 8 | 11 | 20 |
Notes: Based on the Hausman test, all models are estimated using RE.
Standard errors are in parentheses.
p < 0.1;
p < 0.05;
p < 0.01.
Regression results for imported agricultural inputs on agriculture productivity (2SLS-IV estimation)a
These results imply that a 1% increase in imported agricultural inputs increase agriculture output by approximately 0.1% in SSA. The results suggest that promoting imports of agricultural inputs and intermediate goods is an important channel through which agriculture productivity growth as well as economic growth in the region could be realized. The results are consistent with empirical findings in the economic literature (Lawrence and Weinstein, 1999; Gokcekus, 1997; Sangho et al., 2007; Abreha, 2019), that imports enhance productivity whereas protectionism hinders technological advancement and productivity growth.
The positive relationship between agricultural imports and economic growth in SSA could therefore be explained by the fact that most agriculture-based SSA countries import intermediate goods in the production process, which boosts agriculture productivity and consequently economic growth. Many of the modern inputs such as hybrid seeds, pesticides, fertilizers, and machinery are sourced from the world market. Sheahan and Barrett (2014) report that farmers in SSA have increasingly been using external inputs to boost crop yields. Agricultural foreign direct investment in SSA, which has been on the rise recently, may facilitate technology transfer and spillover effects, which can further promote agricultural production in the region. Inadvisable; the exact opposite is more likely, according to the results! Note that the coefficient is significantly negative in the full sample and in the low-income group, suggesting that food imports would likely reduce agricultural productivity; besides, the positive coefficient in the middle-income group is not even significant!
6. CONCLUSION
This study applies Granger causality tests in heterogeneous panels to analyze causal relationships among agricultural imports, agriculture productivity, and GDP per capita growth using panel data for 40 SSA countries over the period 1990–2015. The approach accounts for cross-sectional dependence and provides presumably reliable results even when both N and T are small. The analysis is then extended by using the IV technique with G2SLS for panel-data models to estimate the effects of the variables of interest while controlling for endogeneity. In order to gain more insight, the sample is further divided into three nonoverlapping sub-samples—oil exporters, middle-income non-oil exporters, and low-income non-oil exporters—based on World Bank classification.
The empirical results indicate strong evidence of a feedback relationship between agriculture productivity and agricultural imports in the full sample, middle income non-oil exporters, and low-income non-oil-exporting countries in SSA. In six countries, we find unidirectional causality running from agriculture productivity to agricultural imports, whereas unidirectional causality from agricultural imports to agriculture productivity is evident in six countries. Evidence of bidirectional causality between agriculture productivity and agricultural imports is evident in one country. There is no evidence of causality running from GDP per capita growth to agricultural imports in the full sample or in any of the samples. The results therefore do not support some existing claims in the economic literature that economic growth in SSA contributes to increased agricultural imports in the region. However, there is strong evidence of unidirectional causality running from agricultural imports to GDP per capita growth in the full sample and in middle-income non-oil-exporting countries in SSA. Thus, the agricultural import-led growth hypothesis is supported. Nonetheless, the country-specific causality tests indicate that the import-led hypothesis is strongly supportable in only five out of the 40 countries, with a feedback in two of the countries.
Two main findings are drawn from this study. First, a feedback relationship exists between agriculture productivity and agricultural imports in SSA except in the case of oil-exporting countries, where there is no evidence of causality. Second, economic growth does not significantly cause increased imports of agricultural products in SSA, but agricultural imports support economic growth. The estimated growth elasticity of agricultural imports is about 1.0 in the full sample and 1.3 in middle-income non-oil-exporting countries. Agricultural output elasticity of imported inputs in the region is about 0.1. These findings negate the notion that imports hinder economic growth through import leakages and crowding out of domestic production; instead they show that agricultural imports have a positive and significant impact on agriculture productivity and on economic growth in SSA. Agricultural import of raw materials, and intermediate and capital goods can promote competition, innovation, and absorption of advanced technology in the domestic market, which may enhance productivity and growth. Policy makers should therefore carefully consider their agricultural trade policies in order to promote agricultural trade openness for economic growth and development. In this regard, and as a general rule, policies that favor imports of agricultural inputs and intermediate commodities should be promoted over those geared toward the imports of end products and/or food.
CONFLICTS OF INTEREST
The authors declare they have no conflicts of interest.
AUTHORS’ CONTRIBUTION
Esther Mwangi is the principal author, with significant contributions by the two co-authors.
ACKNOWLEDGMENTS
The authors thank the journal chief editor, Augustin Fosu, and the anonymous referees for their valuable comments. This study was financially supported by the
APPENDIX
Oil exporters | Middle-income non-oil exporters | Low-income non-oil exporters | |
---|---|---|---|
Angola | Cote d’Ivoire | Burkina Faso | Zimbabwe |
Chad | South Africa | Burundi | Central Africa |
Cameroon | Ghana | Guinea | Madagascar |
Congo | Kenya | Malawi | Somalia |
Nigeria | Zambia | Mali | Comoros |
Sudan | Mauritania | Mozambique | Liberia |
Gabon | Mauritius | Niger | Senegal |
Seychelles | Namibia | Rwanda | Sierra Leone |
Lesotho | Gambia | ||
Botswana | Benin | ||
Sao Tome | Togo | ||
Cape Verde | Uganda |
List of countries
Variables | Mean | Std. dev. | Min | Max | Observations | |
---|---|---|---|---|---|---|
agricvperha | Overall | 701.995 | 452.529 | 89.085 | 3290.598 | N = 1040 |
Between | 438.779 | 164.485 | 2727.148 | n = 40 | ||
Within | 129.952 | 52.777 | 1919.444 | T = 26 | ||
GDPpcgrowth | Overall | 1.367 | 5.266 | −47.503 | 37.536 | N = 1040 |
Between | 1.5682 | −1.274 | 5.103 | n = 40 | ||
Within | 5.033 | −49.809 | 35.230 | T = 26 | ||
agricM | Overall | 497,724.5 | 905,867.3 | 6448 | 8,331,117 | N = 1040 |
Between | 672,395.2 | 21,545.23 | 3,114,184 | n = 40 | ||
Within | 615,921.2 | −2,055,419 | 5,714,657 | T = 26 | ||
grosskform | Overall | 21.876 | 9.769 | −2.424 | 61.469 | N = 1040 |
Between | 7.389 | 11.383 | 46.912 | n = 40 | ||
Within | 6.495 | 3.2596 | 57.661 | T = 26 | ||
popgrowth | Overall | 2.455 | 1.107 | −6.766 | 8.118 | N = 1040 |
Between | 0.685 | 0.705 | 3.632 | n = 40 | ||
Within | 0.876 | −6.027 | 8.857 | T = 26 | ||
inflation | Overall | 21.274 | 168.239 | −11.686 | 4145.106 | N = 1040 |
Between | 67.953 | 1.540 | 424.966 | n = 40 | ||
Within | 154.009 | −396.411 | 3741.414 | T = 26 | ||
schenroll | Overall | 35.542 | 22.601 | 5.221 | 115.957 | N = 1040 |
Between | 20.246 | 9.606 | 88.280 | n = 40 | ||
Within | 10.537 | −3.526 | 73.753 | T = 26 | ||
agricL | Overall | 4470.823 | 7297.637 | 1.690 | 52,982.57 | N = 1040 |
Between | 7278.981 | 3.510 | 42,282.22 | n = 40 | ||
Within | 1243.709 | −8461.043 | 15,171.17 | T = 26 |
Notes: For variable definitions and data sources, see Table 1.
Descriptive statistics of the variables
Country | H0: Agriculture productivity does not Granger-cause agricultural imports | H0: Agricultural imports does not Granger-cause agriculture productivity | ||||
---|---|---|---|---|---|---|
|
|
95% critical value |
|
|
95% critical value | |
Angola | 8.362 | 4.483** | 4.090 | 0.001 | −0.654 | 4.556 |
Benin | 1.166 | 0.039 | 2.906 | 0.152 | −0.564 | 3.200 |
Botswana | 0.002 | −0.653 | 3.351 | 0.179 | −0.548 | 3.249 |
Burkina Faso | 0.626 | −0.282 | 3.357 | 0.404 | −0.415 | 3.209 |
Burundi | 1.928 | 0.493 | 2.279 | 14.725 | 8.109** | 1.899 |
Chad | 0.523 | −0.343 | 2.137 | 0.253 | −0.504 | 2.221 |
Cameroon | 3.239 | 1.273 | 4.487 | 1.645 | 0.324 | 5.163 |
Congo | 5.441 | 2.583 | 6.184 | 0.000 | −0.655 | 4.039 |
Cote d’Ivoire | 0.650 | −0.268 | 3.604 | 3.705 | 1.551 | 3.984 |
Guinea | 0.965 | −0.081 | 3.715 | 0.121 | −0.583 | 3.768 |
Ghana | 0.610 | −0.292 | 4.422 | 8.749 | 4.552 | 4.771 |
Kenya | 11.253 | 6.042** | 3.949 | 0.001 | −0.699 | 4.302 |
Malawi | 8.720 | 4.535** | 3.423 | 1.345 | 0.146 | 3.270 |
Mali | 1.904 | 0.478 | 2.341 | 3.596 | 1.436 | 2.616 |
Mozambique | 4.894 | 2.258 | 2.748 | 11.800 | 6.368** | 2.802 |
Niger | 2.458 | 0.808 | 4.510 | 0.842 | −0.164 | 4.206 |
Nigeria | 8.304 | 4.287** | 3.968 | 1.275 | 0.104 | 4.795 |
Rwanda | 11.112 | 5.959** | 3.735 | 0.992 | −0.064 | 3.809 |
Senegal | 0.628 | −0.281 | 3.244 | 19.761 | 11.106** | 2.555 |
Sierra Leone | 6.158 | 3.010 | 3.320 | 4.989 | 2.315 | 3.744 |
South Africa | 2.462 | 0.810 | 4.495 | 5.870 | 2.839 | 5.301 |
Sudan | 0.610 | −0.292 | 2.761 | 0.588 | −0.305 | 3.407 |
Togo | 3.057 | 1.165 | 2.778 | 0.450 | −0.387 | 2.901 |
Uganda | 4.405 | 1.967 | 2.995 | 0.060 | −0.619 | 3.633 |
Zambia | 16.697 | 9.282** | 3.523 | 12.006 | 6.490** | 3.910 |
Zimbabwe | 1.772 | 0.400 | 2.585 | 0.644 | −0.217 | 2.727 |
Central Africa | 2.817 | 1.022 | 3.065 | 7.831 | 4.006* | 3.313 |
Madagascar | 8.960 | 4.678 | 5.269 | 1.654 | 0.330 | 4.444 |
Somalia | 11.741 | 6.333** | 2.780 | 0.192 | −0.540 | 3.203 |
Mauritania | 5.308 | 2.504 | 3.336 | 4.725 | 2.157 | 3.274 |
Mauritius | 0.206 | −0.533 | 3.407 | 15.335 | 8.472** | 4.019 |
Namibia | 0.334 | −0.456 | 2.530 | 0.141 | −0.571 | 2.620 |
Sao Tome | 3.469 | 1.410 | 3.781 | 0.896 | −.0121 | 3.873 |
Seychelles | 2.084 | 0.585 | 3.766 | 4.371 | 1.947 | 3.649 |
Cape Verde | 2.739 | 0.975 | 3.784 | 0.000 | −0.361 | 4.517 |
Comoros | 0.150 | −0.566 | 2.114 | 2.941 | 1.096 | 4.412 |
Gabon | 1.522 | 0.251 | 3.813 | 0.432 | −0.397 | 3.492 |
Gambia | 3.074 | 1.175 | 2.798 | 7.802 | 3.989** | 2.921 |
Lesotho | 2.409 | 0.779 | 2.041 | 3.387 | 1.361 | 1.834 |
Liberia | 0.700 | −0.238 | 2.243 | 1.379 | 0.166 | 3.073 |
The data used cover the full sample period from 1990 to 2016.
p < 0.1;
p < 0.05;
p < 0.01.
Causality between agriculture productivity and agricultural importsa
Country | H0: GDP per capita growth does not Granger-cause agricultural imports | H0: Agricultural imports does not Granger-cause GDP per capita growth | ||||
---|---|---|---|---|---|---|
|
|
95% critical value |
|
|
95% critical value | |
Angola | 0.491 | −0.363 | 2.140 | 0.191 | −0.541 | 2.526 |
Benin | 0.499 | −0.358 | 1.956 | 0.220 | −0.530 | 1.967 |
Botswana | 0.391 | −0.422 | 1.881 | 0.015 | −0.646 | 1.842 |
Burkina Faso | 0.040 | −0.631 | 2.012 | 0.046 | −0.627 | 1.806 |
Burundi | 0.159 | −0.560 | 2.796 | 0.041 | −0.630 | 2.200 |
Chad | 0.312 | −0.469 | 1.981 | 0.049 | −0.626 | 1.908 |
Cameroon | 1.596 | 0.295 | 3.088 | 0.166 | −0.556 | 3.351 |
Congo | 0.054 | −0.623 | 2.742 | 3.448 | 1.398 | 2.377 |
Cote d’Ivoire | 6.911 | 3.458* | 2.478 | 5.759 | 2.773** | 2.729 |
Guinea | 0.562 | −0.321 | 1.661 | 2.297 | 0.712 | 1.888 |
Ghana | 9.012 | 4.708* | 3.007 | 18.599 | 10.414*** | 2.765 |
Kenya | 1.990 | 0.530 | 2.404 | 4.837 | 2.250* | 2.224 |
Malawi | 0.312 | −0.469 | 1.978 | 0.938 | −0.096 | 1.920 |
Mali | 0.765 | −0.200 | 1.872 | 0.997 | −0.061 | 1.874 |
Mozambique | 0.316 | −0.467 | 2.314 | 0.000 | −0.655 | 1.690 |
Niger | 0.799 | −0.179 | 1.828 | 4.044 | 1.752 | 2.435 |
Nigeria | 0.797 | −0.181 | 2.790 | 0.567 | −0.318 | 2.335 |
Rwanda | 0.045 | −0.628 | 2.296 | 0.042 | −0.630 | 1.946 |
Senegal | 0.001 | −0.654 | 2.053 | 0.312 | −0.469 | 2.363 |
Sierra Leone | 0.063 | −0.617 | 2.164 | 0.079 | −0.608 | 1.997 |
South Africa | 1.603 | 0.299 | 2.662 | 0.562 | −0.320 | 2.983 |
Sudan | 1.655 | 0.330 | 2.029 | 0.585 | −0.307 | 1.694 |
Togo | 0.578 | −0.311 | 2.466 | 0.285 | −0.485 | 2.028 |
Uganda | 0.810 | −0.173 | 1.847 | 1.323 | 0.133 | 2.477 |
Zambia | 1.277 | 0.105 | 2.863 | 2.063 | 0.573 | 2.168 |
Zimbabwe | 0.000 | −0.655 | 2.378 | 1.950 | 0.506 | 2.369 |
Central Africa | 3.354 | 1.341 | 1.645 | 0.849 | −0.149 | 1.704 |
Madagascar | 1.056 | −0.026 | 1.925 | 0.212 | −0.529 | 1.934 |
Somalia | 0.162 | −0.558 | 1.664 | 0.363 | −0.438 | 1.804 |
Mauritania | 0.232 | −0.517 | 1.687 | 0.151 | −0.565 | 2.331 |
Mauritius | 0.010 | −0.649 | 1.589 | 0.136 | −0.574 | 1.982 |
Namibia | 0.528 | −0.341 | 2.328 | 0.975 | −0.074 | 2.078 |
Sao Tome | 1.426 | 0.194 | 2.128 | 4.927 | 2.367** | 2.277 |
Seychelles | 0.231 | −0.517 | 1.874 | 0.002 | −0.653 | 2.119 |
Cape Verde | 0.015 | −0.646 | 2.363 | 6.013 | 3.062** | 2.924 |
Comoros | 0.509 | −0.352 | 2.299 | 1.142 | 0.025 | 1.705 |
Gabon | 0.712 | 0.778 | 2.080 | 0.689 | −0.245 | 2.115 |
Gambia | 0.406 | −0.413 | 1.821 | 3.471 | 1.411 | 2.040 |
Lesotho | 0.872 | −0.136 | 2.009 | 0.320 | −0.464 | 2.071 |
Liberia | 0.140 | −0.571 | 1.779 | 2.246 | 0.682 | 2.287 |
The data used cover the full sample period from 1990 to 2016.
p < 0.1;
p < 0.05;
p < 0.01.
Causality between agricultural imports and economic growtha
Footnotes
See Dumitrescu and Hurlin (2012) for details on the step-by-step bootstrapping procedure.
The results for country-level causality analysis are presented in Appendix Tables A3 and A4.
REFERENCES
Cite this article
TY - JOUR AU - Esther N. Mwangi AU - Fuzhong Chen AU - Daniel M. Njoroge PY - 2020 DA - 2020/09/08 TI - Agricultural Imports, Agriculture Productivity and Economic Growth in Sub-Saharan Africa JO - Journal of African Trade SP - 15 EP - 28 VL - 7 IS - 1-2 SN - 2214-8523 UR - https://doi.org/10.2991/jat.k.200902.001 DO - 10.2991/jat.k.200902.001 ID - Mwangi2020 ER -