Baseline Photos and Confident Annotation Improve Automated Detection of Cutaneous Graft-Versus-Host Disease
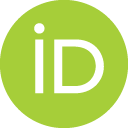
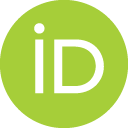
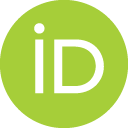
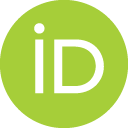
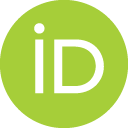
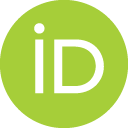
- DOI
- 10.2991/chi.k.210704.001How to use a DOI?
- Keywords
- Cutaneous erythema; chronic graft-versus-host disease; change detection; longitudinal photos; baseline photos; computer vision; convolutional neural networks
- Abstract
Cutaneous erythema is used in diagnosis and response assessment of cutaneous chronic graft-versus-host disease (cGVHD). The development of objective erythema evaluation methods remains a challenge. We used a pre-trained neural network to segment cGVHD erythema by detecting changes relative to a patient’s registered baseline photo. We fixed this change detection algorithm on human annotations from a single photo pair, by using either a traditional approach or by marking definitely affected (“Do Not Miss”, DNM) and definitely unaffected skin (“Do Not Include”, DNI). The fixed algorithm was applied to each of the remaining 47 test photo pairs from six follow-up sessions of one patient. We used both the Dice index and the opinion of two board-certified dermatologists to evaluate the algorithm performance. The change detection algorithm correctly assigned 80% of the pixels, regardless of whether it was fixed on traditional (median accuracy: 0.77, interquartile range 0.62–0.87) or DNM/DNI segmentations (0.81, 0.65–0.89). When the algorithm was fixed on markings by different annotators, the DNM/DNI achieved more consistent outputs (median Dice indices: 0.94–0.96) than the traditional method (0.73–0.81). Compared to viewing only rash photos, the addition of baseline photos improved the reliability of dermatologists’ scoring. The inter-rater intraclass correlation coefficient increased from 0.19 (95% confidence interval lower bound: 0.06) to 0.51 (lower bound: 0.35). In conclusion, a change detection algorithm accurately assigned erythema in longitudinal photos of cGVHD. The reliability was significantly improved by exclusively using confident human segmentations to fix the algorithm. Baseline photos improved the agreement among two dermatologists in assessing algorithm performance.
- Copyright
- © 2021 International Academy for Clinical Hematology. Publishing services by Atlantis Press International B.V.
- Open Access
- This is an open access article distributed under the CC BY-NC 4.0 license (http://creativecommons.org/licenses/by-nc/4.0/).
Download article (PDF)
View full text (HTML)
Cite this article
TY - JOUR AU - Xiaoqi Liu AU - Kelsey Parks AU - Inga Saknite AU - Tahsin Reasat AU - Austin D. Cronin AU - Lee E. Wheless AU - Benoit M. Dawant AU - Eric R. Tkaczyk PY - 2021 DA - 2021/07/15 TI - Baseline Photos and Confident Annotation Improve Automated Detection of Cutaneous Graft-Versus-Host Disease JO - Clinical Hematology International SP - 108 EP - 115 VL - 3 IS - 3 SN - 2590-0048 UR - https://doi.org/10.2991/chi.k.210704.001 DO - 10.2991/chi.k.210704.001 ID - Liu2021 ER -