Regional Integration and Growth Spillovers: Is Africa an Economic Space?
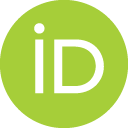
- DOI
- 10.2991/jat.k.200724.001How to use a DOI?
- Keywords
- Regional integration; trade; growth spillovers; Africa; spatial dynamic panel
- Abstract
Market integration is often associated with economic growth spillovers, to the extent that strengthened trade linkages would make economic growth in one country an opportunity for growth to its partners. This paper uses a spatial dynamic panel data approach to analyze the extent to which Africa and its major regional groupings have been able to generate shared growth. The results indicate that the continent as a whole is indeed a spatially integrated economic zone. Although of a weak proportion compared to advanced economies, the contemporaneous spatial growth effect is associated with strong growth convergence and a large rate of return to physical capital. The results also suggest that space has more to do with bilateral trade than geographical distance, and point to a great deal of heterogeneity: the positive growth spillover effect in regional groupings such as South African Development Authority, Common Market for Eastern and Southern African, and Economic Community of West African States, which happen to register larger intraregional trade shares, contrast with the absence of such an effect in Economic Community of Central African States and Arab Maghreb Union, two blocks which have achieved little in terms of economic integration. These results should be a welcome addition to the debate over the opportunity of the proposed continent-wide free trade area and its ability to foster inclusive and sustainable economic growth and development for African economies.
- Copyright
- © 2020 African Export-Import Bank. Publishing services by Atlantis Press International B.V.
- Open Access
- This is an open access article distributed under the CC BY-NC 4.0 license (http://creativecommons.org/licenses/by-nc/4.0/).
1. INTRODUCTION
The quest for regional integration has always been a key component for economic growth and development strategies in Africa. The expansion of goods markets as a result of the removal of trade barriers has the potential to overcome the many constraints associated with small national markets and provide opportunities for technology transfers, increased productivity, income generation, as well as job creation.
The literature on the trade-growth nexus with a focus on the African context has shown that increased trade tends to positively impact economic growth (see, e.g., Fosu, 1990; Musila and Yiheyis, 2015; Zahonogo, 2016). To the extent that trade openness is a result of discriminatory trade policies, the literature also tends to lend support to the view that greater trade ties through regional integration lead to economic growth. For instance, Vamvakidis (1998) has indicated that regional integration, a form of discriminatory openness, matters for economic growth, as “countries with open, large, and more developed neighboring economies grow faster than those with closed, smaller, and less developed neighboring economies” (p. 1). Additional evidence on the potentially positive impact of regional trade on growth from both theoretical and empirical perspectives includes the work of Dion (2004), who shows that a reduction in transaction costs as a result of the phasing out of trade barriers can lead to faster growth and convergence in member countries, and Bong and Premaratne (2018), who find that regional integration has a significant effect on economic growth for Southeast Asian countries, as does te Velde (2011) for a larger sample of countries.
These various empirical assessments of the growth impact of regional integration tend to fall short of capturing the regional spillover effects often associated with stronger economic ties. Trade flows can indeed act as a channel through which economic growth in one country benefits growth in its trading partners. The latter will have the opportunity to meet an increasing demand for their domestic goods, hence an expansion in domestic output and exports. On the import side, growth in neighboring countries often spells a greater availability of imported goods for the domestic economy, either for final consumption or domestic production. The same can apply to the case of Gross Domestic Product (GDP) contraction in one country that may also impair growth in the trade-dependent neighbors, in terms of reduced export markets and production as well as decreased imports. Trade in the end tends to tie the overall economic dynamics of trading partners.
Trade can also tie nations’ growth trajectories. It serves as a vehicle for knowledge flows and technology transfer. Importing knowledge- or technology-embodied inputs (mostly machinery or equipment) has been shown to benefit domestic growth. For instance, Coe and Helpman (1995) and Keller (2004) provide evidence, in the case of developed economies, that domestic productivity is positively related to imports from partners that spend more on Research and Development (R&D). Seck (2012) provides similar evidence of growth-enhancing technology diffusion through trade between developed and developing economies (more so than foreign direct investment).
Taking account of these growth spillover effects is more in line with the underlying motive that governs regional integration. Through economic integration, member countries aim to foster convergent economic trajectories that ensure shared prosperity. The expected mutual benefits are often underestimated by a typical regression analysis that relates growth to trade.
There is an emerging empirical literature that strives to capture these spillover effects, borrowing from spatial economics. The spillover effects mean that, through trade linkages, growth in one country will benefit growth in its trading partners. Empirical evidence tends to provide ample evidence for growth spillovers through trade. For instance, Ho et al. (2013) have shown that in the context of Organisation for Economic Co-operation and Development (OECD) countries, the growth spillover effect is significant as a result of bilateral trade, although it turns out not to be strongly persistent. Ignoring this effect has been shown to underestimate the growth convergence among these countries. Additional evidence of spatial dependence of national economic growth includes that provided by Ho et al. (2018) for OECD countries, Seif et al. (2017) for the Middle East and North Africa area, Yu (2012) for the US states, Tian et al. (2010) for Chinese provinces, and Ertur and Koch (2007) for a large set of countries, to name a few.
This research adds to this burgeoning empirical literature on trade-related spatial growth spillover by focusing on the African context, which offers a true picture of a “spaghetti bowl.”1 In effect, the continent is made up of eight major Regional Economic Communities (RECs), and each one of the 54 countries is a member of at least one REC.2 These regional groupings tend to differ in many respects, for instance in terms of economic or population size, age, and mostly, main objectives and actual level of implementation. All of these tend to translate into various levels of trade and economic proximity among member countries. More specifically, most RECs aim to achieve full economic union [such as the Economic Community of West African States (ECOWAS), or the Southern African Development Community (SADC)], whereas others focus more on the realization of a common market [such as the Common Market for Eastern and Southern African (COMESA)]. These various objectives, coupled with different and slow implementation processes, are key reasons to expect differential growth spillovers. Taking stock of the heterogeneous regional integration experiences, in terms of why some may have generated significant economic growth externalities, whereas others have failed to do so, could inform the design of an effective continent-wide integration scheme that would promote inclusive and sustainable development, which so far has been elusive for a host of African countries.
This study aims to assess the regional growth potential of economic integration in Africa. More specifically, it aims to (1) determine the extent of economic growth spillover through regional trade across the continent, and (2) analyze how various specificities of the regional integration processes explain the likely heterogeneity in the ability of the regional communities to generate growth spillovers.
The paper develops a spatial dynamic panel data model to capture any spatial dependence of national growth trajectories through trade linkages. The spatial weight matrix is constructed using bilateral trade flows (and, alternatively, the more traditional geographical distance). The spatial dimension is then added to a standard Solow growth framework that accounts for additional insights from the new growth theory. The model specification includes both a spatial autoregressive term and a spatial time lag term.
The results suggest strong evidence of a positive growth spillover effect across the continent as a whole, and trade turns out to be more conducive to these spillover gains than geographic proximity. However, the spatial dependence appears to be less strong than the one found in developed countries. Moreover, accounting for the spatial dependence leads to a greater economic convergence. In addition, regional groupings such as SADC, ECOWAS, and COMESA appear to be driving the results, as opposed to the Arab Maghreb Union (AMU) and Economic Community of Central African States (ECCAS), owing to the larger share of intraregional trade, as well as their significant effort to promote productive integration trough the development of regional value chains, financial and macroeconomic integration that ensures easier convertibility of national currencies, regional infrastructure, as well as free movement of people. All of these differential aspects could serve as key policy directions to further integrate the whole continent and ensure inclusive economic growth and development.
The rest of the paper unfolds as follows. Section 2 discusses the literature. Section 3 introduces the methodology and the data. Section 4 reports and discusses the results. Section 5 concludes.
2. LITERATURE REVIEW
Regional integration has been shown to potentially generate significant economic gains. But until relatively recently, the literature had focused on how a country’s trade expansion can benefit its own economic growth trajectory. Such an expansion can be a result of falling tariff and nontariff barriers in the wake of free trade arrangements. Most of the trade theories suggest indeed that international trade can lead to increased efficiency, economic growth, and income generation by allowing greater capital accumulation, industrial structure upgrading, technological progress, and institutional advancement.
More specifically, a large segment of the empirical literature has indicated that trade has a significant growth potential. Early contributions have shown that outward-oriented developing countries tend to enjoy rapid economic growth than their inward-oriented counterparts (Balassa, 1986; Dollar, 1992), in addition to alleviating poverty and reducing inequalities (Dollar and Kraay, 2004). In fact, international trade provides a country with increased supply of capital and intermediate inputs, which are not available in the domestic markets. As a result, productivity of the manufacturing sector can rise (Lee, 1995).
International trade is also a channel through which foreign technology and knowledge diffuse to the domestic economy, which then translates into substantial aggregate productivity gains and economic growth. By importing technology-embodied goods, such as machinery and equipment, the domestic economy acquires advanced technology to the extent that it develops its absorption capabilities (Coe and Helpman, 1995; Keller, 2004; Seck, 2012).
On the export side, a greater participation in foreign markets, where domestic firms face international competition, can also lead to an increase in productivity (Wagner, 2007). Exports are also associated with a learning-by-doing effect through which firms can copy from more advanced foreign competitors and subsequently gain in terms of increased efficiency (Kraay, 1999).
There is also ample evidence that casts some doubt on this positive growth effect of trade and openness, as a result for instance of reduced tariffs. As far as the post-war period is concerned, authors such as Vamvakidis (2002),Yanikkaya (2003), and DeJong and Ripoll (2006) have found a positive correlation between average tariff level (reduced trade) and long-term economic growth.3 This is the case when government’s tariff targets industries subject to externalities, as suggested by Krugman’s (1987) model of learning-by-doing and Grossman and Helpman’s (1993) endogenous growth model with R&D externalities.4
These unsettled results on whether more trade leads to economic growth have extended to the recent empirical literature mostly focused on Africa. For instance, Musila and Yiheyis (2015) have indicated in the case of Kenya that more trade does not, at best, lead to significant growth gains. Moreover, they have found trade-policy-induced openness to have negatively and significantly affected investment and the rate of economic growth. By contrast, Zahonogo (2016) has shown that trade has a beneficial impact on growth up to a threshold level of openness, above which the effect then declines. To the extent that African countries tend to trade less with each other and with the rest of the world (below the critical thresholds), increasing trade can therefore be good for economic growth.
The growth spillover associated with increased trade, however, tends to be ignored in this literature, thereby underestimating the economic growth effect of increased trade ties, as a result of, for instance, regional integration. A relatively recent literature sought to directly capture the spillover effect by borrowing from geography economics. It builds on earlier attempts that relate a country’s growth to the average growth of its trading partners. Such an approach tends to be not satisfactory, as it does not account for the heterogeneity of the trade ties across partners. Instead, spatial approaches relate a country’s growth to individual growth of its neighbors. The links are obtained through a weighting matrix constructed by using bilateral trade flows.
This approach is better able to reveal the trade potential of regional integration. The spatial nature of the economic relationships within the regional grouping is synonymous with some synchronization of the growth trajectories.
Important contributions to this literature include those presented by Ho et al. (2013). They developed an extended framework of the Solow growth model by considering the spillover effect due to bilateral trade in the context of OECD countries. They showed that trade is indeed associated with significant growth spillovers, although the effect was not persistence. Accounting for this growth spillover effect also meant that the rate convergence was higher than otherwise. Furthermore, Ho et al. (2018) provided similar evidence in the context of advanced countries. Additional contributions with a focus on developing countries include those provided by Tian et al. (2010) for China and Seif et al. (2017) for Arab countries in the Middle East and North Africa.
This paper brings to this literature the unique context of the African continent, which is a place where regional integration has always been a significant part of the economic processes. This has resulted into a dense web of economic integration arrangements. Despite this strong appetite for economic integration, Africa is also a place that trades less with itself. The comparative approach that looks at the regional specifics will be able to shed light on the various mechanisms that underlie the process of spatially generated growth spillovers associated with regional integration.
3. METHODOLOGY AND DATA
The starting point is the Solow growth model with a Cobb–Douglas production
To relax the assumption of closed economy and allow for countries to interact through trade, we consider the following equation, augmented with spatial terms:
As a first approximation, we follow the standard literature that uses spatial econometrics to address various economic issues and construct W using geographical distance. This allows us to see whether spatial interdependence across countries occurs “naturally,” as it may relate to an exogenous proximity. The elements are then obtained from wij = exp(−dij), with dij the distance between countries i and j, or more precisely their capital cities (see Keller, 2004; Ertur and Koch, 2007; and Ho et al., 2013, for applications in the literature).
We next consider bilateral flows trij, and the elements of the spatial weight matrix are simply wij = trij, averaged over the period (time-invariant). This trade-related matrix could provide room for altering the economic proximity through more or less trade. Ho et al. (2013) also considered both definitions of proximity, and showed that both are associated with spatial correlation, with a greater spillover effect through the trade channel.
The estimation strategy, based on a quasi-maximum likelihood estimate approach, consists of first running the regressions for all African countries, and then separately for the major regional blocs, namely, COMESA, AMU, ECCAS, ECOWAS, and SADC.8 These regional communities span the whole continent. The remaining regional communities tend to either overlap [e.g., West African Economic and Monetary Union with respect to ECOWAS, and Intergovernmental Authority on Development and the East African Community (EAC) to COMESA—with the exception of Somalia, South Sudan, and Tanzania], or too large and heterogeneous [Community of Sahel–Saharan States with 24 member countries]. The pooling of all the countries will tell how, on average, one country’s growth is beneficial to the neighboring countries. The separate regressions will help reveal the specificities that make a given integration process more prone to generate growth spillover than the rest.
Table 1 offers some descriptive statistics of the data. It appears that the continent is trading little with itself, despite the dense web of free trade agreements. Nevertheless, the data clearly indicate positive trends for all regional groupings. In effect, they are improving their trade ties, as the share of intraregional trade is rising. SADC and COMESA have seen the largest increase, with 8.4% and 5.9% points, respectively. On the opposite end, regions with the smallest increase (AMU and ECCAS) turn out to be those where intraregional trade is the lowest.
Africa | COMESA | AMU | ECCAS | ECOWAS | SADC | |
---|---|---|---|---|---|---|
GDP p.c. 2016 (constant 2010$) | 2693.6 | 2818.8 | 3383.9 | 3402.5 | 1093.6 | 3864.3 |
GDP p.c. growth, 2000–2016 (%) | 2.1 | 1.5 | 1.7 | 2.2 | 1.6 | 2.4 |
Intraregional trade, 2016 (%) | 12.6 | 12.4 | 3.4 | 4.2 | 10.8 | 19.2 |
Intraregional trade, change 2000–2016 | 1.4 | 5.9 | 0.8 | 1.1 | 1.9 | 8.4 |
Area (million sq. km) | 30.8 | 12.0 | 5.8 | 6.5 | 5.1 | 10.0 |
# of member countries | 54 | 19 | 5 | 11 | 15 | 15 |
Area/member | 0.57 | 0.63 | 1.16 | 0.59 | 0.34 | 0.67 |
Population growth, 2000–2016 (%) | 2.5 | 2.3 | 1.9 | 3.0 | 2.7 | 2.2 |
Investment rate, 2000–2016 (% GDP) | 22.5 | 18.7 | 27.0 | 22.9 | 20.6 | 22.3 |
Inflation (GDP deflator %, 2016, and change from 2000) | 10.1 (−79) | 5.0 (176) | 1.9 (−7) | 4.1 (−320) | 3.8 (−8) | 8.7 (−236) |
Regulation (2016, and change) | 3.43 (−0.22) | 3.31 (−0.08) | 3.04 (−0.79) | 3.07 (0.25) | 3.76 (−0.37) | 4.09 (−0.25) |
The scores for the regulation quality, initially ranging from −2.5 (weak) to +2.5 (strong), have been rescaled to 0–10.
Source: Authors’ calculations, from World Bank, World Development Indicators (WDI) and World Governance Indicators (WGI), United Nations Commodity Trade (COMTRADE) Database and UNECA.
Arab Maghreb Union, in addition to recording the smallest share and slowest pace of intraregional trade, also turns out to be relatively larger in terms of geographical size as it relates to the number of member countries. The figure of 1.16 million square miles (sq.m.) is an indication of the relatively long distance a good produced in a typical member country has to travel in order to get to the national border and be traded with a member partner. By contrast, the group that trades the most with itself (SADC) is also the place where geographical size is relatively smaller (0.67 million sq.m., versus 0.34 million sq.m. for ECOWAS). This is a suggestion that size (or distance) might be a potential predictor of cross-regional trade.
A far as economic growth is concerned, the association with intraregional trade seems less obvious. In fact, the region with the largest share of and increase in intraregional trade (SADC) is also the one that has posted the largest GDP per capita growth rate over the 16-year period (2.4%), but the second largest region, intra-trade-wise (COMESA), has experienced the slowest growth rate of 1.5%. Furthermore, ECCAS, which is the second less trade-integrated region, has posted the second largest growth rate of 2.2%.
These figures do not reveal a definite, clear trend as to the extent to which domestic growth could benefit from neighboring countries’ growth, either through trade or through geographical distance, as with the association between growth and inflation on one hand, and growth and governance quality, on the other hand. A more robust analysis should come up with a better representation of spatial dependency through a spatial weight matrix, but also design an alternative way through which growth spillover effects could materialize. The framework would then allow growth in one country to benefit growth in neighboring countries as a result of greater trade (or geographical) proximity, while actually controlling for factors relevant to growth and technological progress at the steady state equilibrium.
4. RESULTS AND DISCUSSION
Table 2 shows the results for various model specifications for the continent as a whole. Going from a standard dynamic panel specification (column 1) to the augmented one with a spatial autoregressive term (columns 2 and 3), the associated coefficient (λ) appears to be significant. This suggests that results from the first standard model that ignores this relevant spatial dependence are likely to be biased, on one hand, and that Africa as whole is indeed an effectively integrated economic space to the extent that it allows economic growth externalities across countries, on the other hand. Growth in one country benefits growth in partner countries, as it is the case for OECD countries (Ho et al., 2013), for OECD and developing countries (Ertur and Koch, 2011 and 2007), and for US states (Yu and Lee, 2012).
Variables - coefficients | (1) | (2) | (3) | (4) | (5) |
---|---|---|---|---|---|
ln(yit−1)—(γ) | 0.893*** (0.08) | 0.898* (0.49) | 0.854* (0.48) | 0.882*** (0.08) | 0.873*** (0.08) |
ln(nit + 0.05)—(β1) | −0.178* (0.13) | −0.182** (0.08) | −0.276** (0.14) | −0.263*** (0.04) | −0.259* (0.14) |
ln(sit)—(β2) | 0.078 (0.08) | 0.192** (0.09) | 0.296*** (0.08) | 0.223** (0.09) | 0.079 (0.09) |
ln(1 + Infit)—(β3) | −0.043** (0.02) | −0.039* (0.02) | −0.038* (0.02) | −0.046** (0.02) | −0.047** (0.02) |
ln(Instit)—(β4) | 0.068 (0.08) | −0.059 (0.08) | −0.062 (0.09) | 0.035 (0.07) | −0.029 (0.08) |
λ | − (−) | 0.015* (0.08) | 0.237* (0.14) | 0.153* (0.09) | 0.286** (0.11) |
ρ | − (−) | − (−) | − (−) | −0.068 (0.16) | 0.142 (0.09) |
N | 46 | 46 | 46 | 46 | 46 |
T | 8 | 8 | 8 | 8 | 8 |
Obs. | 368 | 368 | 368 | 368 | 368 |
Implied α | 0.421 | 0.653 | 0.669 | 0.653 | 0.384 |
Convergence rate | 0.057 | 0.054 | 0.079 | 0.063 | 0.068 |
Spatial weights | No | Geog. dist. | Trade | Geog. dist. | Trade |
Country FE | Yes | Yes | Yes | Yes | Yes |
Year FE | Yes | Yes | Yes | Yes | Yes |
The dependent variable is log of real GDP per capita. The common convergence rates (c) are obtained from γ = exp(−ct), with t = 2 (the time interval), and the elasticity of output with respect to capital (α) from
Estimation results for the whole African continenta
Our results also suggest that both trade and physical distance are conducive to growth spillovers across Africa. Comparing between these two channels, the spatial dependence appears to occur more through trade than through geographical distance, suggesting that neighborhood or proximity is more relevant as an economic concept than a physical concept, in the African context. In effect, the coefficient estimate on the spatial time lag term is higher when the spatial weight matrix is constructed by using bilateral trade than physical distance. Moreover, the common convergent rate of 7.9% is higher when trade-related spatial weight is considered than weight constructed through physical distance (5.4%), and even when ignoring spatial dependence (5.7%). Furthermore, the estimated elasticity of output with respect to capital (α) is also higher at 66.9% for the trade-weighted spatial model.
These results appear to be generally consistent with the literature although with some noticeable differences. The higher convergence rate associated with the inclusion of spatial dependence in standard models is also found by Ho et al. (2013) for OECD countries, although our estimated convergence rate appears to be much larger (7.9% against 5.2% in the case of OECD countries, for the trade-weighted spatial model). Like Ho et al. (2013), the notion of neighborhood is more associated with trade than pure geographical distance, as far as growth spillovers are concerned. However, their estimated spatial autoregressive coefficient is more than twice as much as our estimate (0.589 against 0.237). This is an indication that the African continent is less of an integrated economic space than the OECD group when it comes to trade-related growth externalities. This is because these advanced economies trade more with one another (65% within the EU and 50% between US, Mexico, and Canada in 2015), and the production base (GDP) is much larger and allows for more exports and imports, compared to African economies.9
Furthermore, the general premise that macroeconomic stability is good for economic growth is supported by the results, as reduced inflation is associated with increased real GDP per capita. Institutional quality, captured by the quality of government regulation, however, does not seem to be significantly correlated with economic growth. This could be an indication of effective coping mechanisms in the face of an unfriendly (business) environment, or a lack of improvement in public governance (in fact, Table 1 indicates a slightly deteriorating institutional quality during the time period).
When the spatial time lag is introduced (columns 4 and 5), the associated coefficient (ρ) turns out to be insignificant, regardless of how the spatial weight matrix is constructed. Although the inclusion of an irrelevant spatial time lag term tends not to be associated with an obvious efficiency loss, as suggested by Yu (2012), we prefer dropping that variable. In the end, growth spillovers happen contemporaneously: domestic growth in a given year would benefit from growth happening the same year in neighboring countries, not from growth in the past (2 years). We therefore favor model specification (3), Table 2—contemporaneous spatial autoregressive term, with trade-related spatial weight matrix—to see whether this broad continental picture of spatially integrated economies may be hiding some heterogeneity among the component regional blocs.
As one would have expected, Table 3 shows that regional groupings that happen to be trading more within themselves (COMESA, ECOWAS, and SADC) appear to be driving the results in Table 2, as their combined member countries make up most of the continent (87%). In effect, only for these three groupings is the trade-related spatial dependence significantly positive.10 The combination of (1) relatively high economic growth, (2) stronger trade ties which have increased more than anywhere else on the continent, and (3) a relatively moderate size of geographical space, which suggests that traded goods have to travel relatively smaller distances to reach national borders, could explain why these entities are more integrated economic spaces, insofar as growth spillovers through trade are concerned.
Variables - coefficients | COMESA | AMU | ECCAS | ECOWAS | SADC |
---|---|---|---|---|---|
ln(yit−1)—(γ) | 0.687*** (0.04) | 0.785 (0.90) | 0.892*** (0.24) | 0.862*** (0.25) | 0.754*** (0.15) |
ln(nit + 0.05)—(β1) | 0.193* (0.11) | −0.283 (0.56) | −0.031 (0.09) | −0.248** (0.11) | −0.252*** (0.09) |
ln(sit)—(β2) | 0.040 (0.03) | 0.397 (0.20) | 0.104** (0.04) | 0.294* (0.17) | 0.293*** (0.07) |
ln(1 + Infit)—(β3) | −0.018 (0.02) | 0.006 (0.18) | −0.023** (0.01) | −0.039* (0.02) | −0.036* (0.02) |
ln(Instit)—(β4) | 0.042 (0.03) | −0.24 (0.85) | 0.068** (0.03) | 0.035 (0.06) | 0.052 (0.12) |
λ | 0.216* (0.12) | 0.193 (0.43) | −0.038 (0.18) | 0.284*** (0.10) | 0.263*** (0.04) |
N | 14 | 5 | 9 | 15 | 12 |
T | 8 | 8 | 8 | 8 | 8 |
Obs. | 112 | 40 | 72 | 120 | 96 |
Implied α | 0.113 | 0.580 | 0.491 | 0.681 | 0.544 |
Convergence rate c | 0.188 | 0.121 | 0.057 | 0.074 | 0.141 |
Spatial weights W | Trade | Trade | Trade | Trade | Trade |
Country FE | Yes | Yes | Yes | Yes | Yes |
Year FE | Yes | Yes | Yes | Yes | Yes |
The dependent variable is log of real GDP per capita. In all regressions, the elements of spatial weight matrix are total bilateral import and export flows. The regressions also include both country and time fixed effects. Standard errors are between parentheses, and significance at 1%, 5%, and 10% is indicated by ***, **, and *, respectively.
Estimation results for regional groupingsa
According to the African Union Commission–African Development Bank–United Nations Economic Commission for Africa (AU–AfDB–UNECA) measurements of regional integration across Africa, bilateral trade has been the dimension along which Africa as a whole has made more effort, with a score of 0.54, as of 2016.11 This is a result of reduced customs duties as well as increased intraregional trade shares. In the opposite, areas where economic integration has been lagging across the continent is financial and macroeconomic integration. The average score of 0.38 suggests that, as far as trade and growth spillovers are concerned, regional convertibility of national currency is still an issue, for it worsens uncertainty and adds to the already high trade transaction costs, thereby reducing trade flows.
Whereas the regional groupings have been making almost similar progress on regional infrastructure (such as intraregional flights and roaming costs) and production integration (such as trade on intermediate inputs and trade complementarity), they tend to fare differently on the fronts of free movement of people and financial and macroeconomic integration, with ECOWAS faring better on both dimensions.
The relationship between growth and institutions on one hand, and with inflation on the other, within the subgroups tend to generally reflect the overall findings for the continent as a whole, shown in Table 2. In effect, higher inflation tends to be associated with, at best, no significant change in economic growth, whereas institutional quality change does not significantly affect growth, except for ECCAS member countries where the impact is more in line with the general finding in the relevant literature on a positive impact of governance quality improvement. This could be because only this region has seen an improvement in the average score over the time period.
Overall, ECOWAS and SADC have registered the highest average scores, mostly when it comes to free movement of people, financial and macroeconomic integration, and regional infrastructure. COMESA has fared relatively well on the dimension of trade and productive integration. The results showing these three regional blocs being more conducive to growth spillover through trade are another translation of their relatively greater effort to integrate member economies. The results are also an indication that, in addition to increased trade flows and regional value chains, free movement of people, financial and macroeconomic integration, and regional infrastructure are key to generating a more integrated economic space that would allow economic growth in any domestic economy to benefit economic growth in its trading partners.
5. SUMMARY AND CONCLUSION
This paper was concerned with the ability of regional integration processes across Africa to generate growth spillover through trade, especially in the prospect of the African Continental Free Trade Area (AfCFTA). Using a spatial dynamic panel data model, the paper showed that Africa is indeed a place where national economic growth benefits from growth in neighboring countries, more so through trade linkages than through geographical proximity. However, the generated spillover gains appeared to be of a small magnitude when compared to economic spaces of more advanced countries, such as OECD or US states. The results also indicated that this overall picture hides a great deal of heterogeneity across RECs, with SADC, ECOWAS, and COMESA registering significant, positive spillover effect, whereas groupings such as ECCAS and AMU show no significant spatial correlation, owing mainly to the differentiated length at which they have gone to improve bilateral trade flows.
For AfCFTA agreements to produce inclusive and sustainable economic growth and development, the spatial dependence of economic entities needs to be further strengthened, so that national growth trajectories could be reinforcing one another, in line with the solidarity spirit that governs this collective initiative. To that end, trade ties have to be further strengthened, for instance, by continuously reducing trade impediments (particularly nontariff barriers that tend to be left out of major arrangements). Harmonized industrial policies should also contribute to the development of regional value chains, on the one hand, whereas financial and macroeconomic policies should ensure less burdensome convertibility among the too many national currencies on the other hand, with the goal of further reducing the high cross-border transaction costs and increasing the growth-spillover conduciveness of trade across the continent.
CONFLICTS OF INTEREST
The authors declare they have no conflicts of interest.
AUTHORS’ CONTRIBUTION
Abdoulaye Seck is the principal author, with research assistance by Founty Fall and Khadidiatou Aidara.
ANNEXES
A. Data Sources
Bilateral trade data are obtained from COMTRADE. Geographical distances are provided by CEPII. Macroeconomic variables (real GDP per capita, and investment rate) and population growth rate come from the World Bank’s World Development Indicators, and data on regulation quality are from the World Bank’s World Governance Indicators. Area size and other relevant information regarding the continent and the regional groupings are from UNECA ( https://www.uneca.org/oria/pages/regional-economic-communities).
B. Regional Groupings and Country Lists
COMESA (Common Market for Eastern and Southern Africa): Burundi, Comoros, Democratic Republic of Congo, Egypt, Ethiopia, Kenya, Libya, Madagascar, Malawi, Mauritius, Rwanda, Uganda, Zambia, and Zimbabwe (exclusion of Djibouti, Eritrea, Seychelles, Sudan and Swaziland).
AMU (Arab Maghreb Union): Algeria, Libya, Mauritania, Morocco, and Tunisia.
ECCAS (Economic Community of Central African States): Angola, Burundi, Cameroon, Central African Republic, Congo, Democratic Republic of the Congo, Equatorial Guinea, Gabon, and Rwanda (exclusion of Chad and Sao Tome and Principe).
ECOWAS (Economic Community of West African States): Benin, Burkina Faso, Cabo Verde, Cote d’Ivoire, Gambia, Ghana, Guinea, Guinea Bissau, Liberia, Mali, Niger, Nigeria, Senegal, Sierra Leone, and Togo.
SADC (Southern African Development Community): Angola, Botswana, Democratic Republic of Congo, Madagascar, Malawi, Mauritius, Mozambique, Namibia, South Africa, Tanzania, Zambia, and Zimbabwe (exclusion of Lesotho, Seychelles, and Swaziland).
Footnotes
Term used by Bhagwati (1995) to refer to the proliferation of preferential trade agreements (PTAs).
In fact, only 11 countries are members of only one REC, whereas seven are members of three RECs (Source: UNECA, https://www.uneca.org/oria/pages/highlights-%E2%80%93-africa-regional-integration-index-report-2016, accessed on March 1, 2019).
On the opposite side of the literature on tariff and long-term growth, Edwards (1992, 1998), Clemens and Williamson (2004), and Nunn and Trefler (2010) provide evidence of a negative correlation.
See Nunn and Trefler (2010) for a detailed discussion.
Source: World Bank (http://info.worldbank.org/governance/wgi/#home, accessed on March 4, 2019).
A more common choice in the literature is a 5-year interval. But given our time span (2000–2016) and the size of our regional groupings (which go to as low as five members for AMU), the closest we can get in satisfying the assumption that the number of individuals N (member states) is always greater than the time dimension T in our panel on one hand, while having sufficiently large number of total observations on the other hand, is by choosing a 2-year window.
For many country pairs, however, the period is shorter than 2000–2016, because of trade data unavailability.
For a detailed discussion of the quasi-maximum likelihood estimate approach and its application using Stata, see Belotti et al. (2016).
Moreover, our implied return to capital, proxied by the elasticity of output with respect to capital, is much higher (66.9% against 51.7%), which could be indicative of the relatively scarce capital stock throughout the continent as opposed to the more advanced economies that make up the OECD.
The results for AMU seem very imprecise and inefficient, as suggested by the larger standard errors, owing certainly to the smaller sample size as well as to the imbalance between the two dimensions of the panel structure (T exceeds N). We also argue that differences in the results are a translation of differentiated integration processes.
The overall index relies on five dimensions: trade integration, regional infrastructure, productive integration, free movement of people, and financial and macroeconomic integration. The score ranges from 0 (lowest) to 1 (highest). The 2016 report can be found here: http://www.tralac.org/images/docs/9384/africa-regional-integration-index-report-2016.pdf (accessed on March 1, 2019).
REFERENCES
Cite this article
TY - JOUR AU - Abdoulaye Seck AU - Founty Alassane Fall AU - Khadidiatou Aidara PY - 2020 DA - 2020/07/29 TI - Regional Integration and Growth Spillovers: Is Africa an Economic Space? JO - Journal of African Trade SP - 37 EP - 44 VL - 7 IS - 1-2 SN - 2214-8523 UR - https://doi.org/10.2991/jat.k.200724.001 DO - 10.2991/jat.k.200724.001 ID - Seck2020 ER -