Machine Learning Techniques for the Detection of Inappropriate Erotic Content in Text
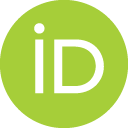
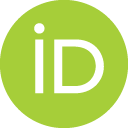
- DOI
- 10.2991/ijcis.d.200519.003How to use a DOI?
- Keywords
- Inappropriate content; Machine learning; Text classification; Natural language processing; Text encoders
- Abstract
Nowadays, children have access to Internet on a regular basis. Just like the real world, the Internet has many unsafe locations where kids may be exposed to inappropriate content in the form of obscene, aggressive, erotic or rude comments. In this work, we address the problem of detecting erotic/sexual content on text documents using Natural Language Processing (NLP) techniques. Following an approach based on Machine Learning techniques, we have assessed twelve models resulting from the combination of three text encoders (Bag of Words, Term Frequency-Inverse Document Frequency and Word2vec) together with four classifiers (Support Vector Machines (SVMs), Logistic Regression, k-Nearest Neighbors and Random Forests). We evaluated these alternatives on a new created dataset extracted from public data on the Reddit Website. The best performance result was achieved by the combination of the text encoder TF-IDF and the SVM classifier with linear kernel with an accuracy of 0.97 and F-score 0.96 (precision 0.96/recall 0.95). This study demonstrates that it is possible to detect erotic content on text documents and therefore, develop filters for minors or according to user's preferences.
- Copyright
- © 2020 The Authors. Published by Atlantis Press SARL.
- Open Access
- This is an open access article distributed under the CC BY-NC 4.0 license (http://creativecommons.org/licenses/by-nc/4.0/).
Download article (PDF)
View full text (HTML)
Cite this article
TY - JOUR AU - Gonzalo Molpeceres Barrientos AU - Rocío Alaiz-Rodríguez AU - Víctor González-Castro AU - Andrew C. Parnell PY - 2020 DA - 2020/06/11 TI - Machine Learning Techniques for the Detection of Inappropriate Erotic Content in Text JO - International Journal of Computational Intelligence Systems SP - 591 EP - 603 VL - 13 IS - 1 SN - 1875-6883 UR - https://doi.org/10.2991/ijcis.d.200519.003 DO - 10.2991/ijcis.d.200519.003 ID - Barrientos2020 ER -