Volume 13, Issue 1, 2020, Pages 576 - 590
Multi-view Genetic Programming Learning to Obtain Interpretable Rule-Based Classifiers for Semi-supervised Contexts. Lessons Learnt
Authors
Carlos García-Martínez*,
, Sebastián Ventura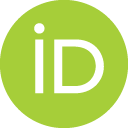
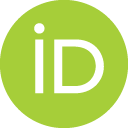
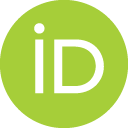
Computing and Numerical Analysis Department, University of Córdoba, Córdoba, 14071, Spain
*Corresponding author. Email: cgarcia@uco.es
Corresponding Author
Carlos García-Martínez
Received 29 January 2019, Accepted 9 April 2020, Available Online 25 May 2020.
- DOI
- 10.2991/ijcis.d.200511.002How to use a DOI?
- Keywords
- Multi-view learning; Rule-based classification; Comprehensibility; Semi-supervised learning; Co-training; Grammar-based genetic programming
- Abstract
Multi-view learning analyzes the information from several perspectives and has largely been applied on semi-supervised contexts. It has not been extensively analyzed for inducing interpretable rule-based classifiers. We present a multi-view and grammar-based genetic programming model for inducing rules for semi-supervised contexts. It evolves several populations and views, and promotes both accuracy and agreement among the views. This work details how and why common practices may not produce the expected results when inducing rule-based classifiers under this methodology.
- Copyright
- © 2020 The Authors. Published by Atlantis Press SARL.
- Open Access
- This is an open access article distributed under the CC BY-NC 4.0 license (http://creativecommons.org/licenses/by-nc/4.0/).
Download article (PDF)
View full text (HTML)
Cite this article
TY - JOUR AU - Carlos García-Martínez AU - Sebastián Ventura PY - 2020 DA - 2020/05/25 TI - Multi-view Genetic Programming Learning to Obtain Interpretable Rule-Based Classifiers for Semi-supervised Contexts. Lessons Learnt JO - International Journal of Computational Intelligence Systems SP - 576 EP - 590 VL - 13 IS - 1 SN - 1875-6883 UR - https://doi.org/10.2991/ijcis.d.200511.002 DO - 10.2991/ijcis.d.200511.002 ID - García-Martínez2020 ER -