Strategic Management of Organizational Knowledge and Employee's Awareness About Artificial Intelligence With Mediating Effect of Learning Climate
- DOI
- 10.2991/ijcis.d.191025.002How to use a DOI?
- Keywords
- Strategic knowledge management; Artificial intelligence; Learning climate; Confirmatory Factor Analysis; Structural Equation Modeling
- Abstract
This study has aimed to examine the empirically relationship between strategic management of organizational knowledge and awareness about artificial intelligence (AI) through mediating effect of learning climate in service sector of Saudi Arabia. For better understanding, a structural questionnaire was developed, and both questionnaire and interview techniques were applied to collect the data from targeted respondents. Statistical methods like confirmatory factor analysis, structural equation modeling and mediation technique were applied to check the direct and indirect effects with overall valid responses of 390. Findings under factor analysis specify that all the indicators of strategic management of knowledge, learning climate and awareness about AI have demonstrated good factor loadings. Additionally, results under structural equation model for strategic management of knowledge and awareness about AI indicates that knowledge acquisition, knowledge dissemination and knowledge responsiveness have their significant and positive relationship with AI awareness. While mediating effect of learning climate (LC), shows that relationship between knowledge acquisition and AI, between knowledge dissemination and AI is significantly mediated by LC under full sample. While mediating effect of LC between knowledge responsiveness and AI is not significant. In addition, practical implications of this study reveals the fact that management in all three layers of the business firms should consider these findings and positive role by learning climate to promote awareness about AI. However, this study has observed several limitations. At first overall impact of strategic management of knowledge is yet to be explored as this study has examined its impact through knowledge acquisition, dissemination, and responsiveness factors under sperate models. At second, study has a regional limitations and specific organizational implications only for the service sector is observed. At third, earlier studies have raised serious concern over data collection through questionnaire as it covers only one dimensions of employee's attitude. Other instruments like open ended non-structural interview should be reconsidered in more significant manner. Future studies could be reimplemented on similar topic while addressing these limitations.
- Copyright
- © 2019 The Authors. Published by Atlantis Press SARL.
- Open Access
- This is an open access article distributed under the CC BY-NC 4.0 license (http://creativecommons.org/licenses/by-nc/4.0/).
1. INTRODUCTION AND BACKGROUND
The factor of globalization has placed the business firms in a situation where market competition and effective attitude in terms of innovation is very much required. In recent years, business firms are working to effectively manage their organizational knowledge while looking for some new ways to survive in the marketplace [1–4]. To effectively manage the organizational knowledge, decision making process seems to be riskier as business firms are moving from domestic market to international arena [5–7]. It is a common notion that business firms who can effectively manage the knowledge assets can create values, identify new opportunities and evolve through better financial outcomes [8–11].
The success factor in the business enterprise depends upon many elements where some are under the control and some are beyond the grip of the management. These factors are the ability of the business to acquire desired level of organizational knowledge [12], distribution or dissemination of organizational knowledge [13,14], and finally the response towards the organizational knowledge [15–26]. However, various issues have been recognized in the earlier research studies as associated to KM in the business. One of the most significant problems is the organization and further distribution of business knowledge [27]. As per the findings of [6,28], it is observed that organizational knowledge has nine basic steps for its management either the explicit, tacit or subjective dimensions. These are transforming the information into knowledge, identification and verification of knowledge, capturing and securing the knowledge, organizing and combining the knowledge, creation and learning of knowledge and finally the distribution and selling of knowledge. Additionally, another significant problem in the knowledge management (KM) is its presentation which refers the way organization will display its knowledge in learning culture [28–35]. However, the problem with the presentation of organizational knowledge is that processing of knowledge is different in front of every employee [1,36,37].
For the growth of KM, various organizations are working for the practice of how a business can improve its value. In this way, the factor of artificial intelligence (AI) and KM are closely associated to each other. The field of AI and KM for the organization, reincarnating and presentation of the knowledge is examined in series of studies. Intelligence is defined as the ability to perceive and process specific level of data and transforming it into meaningful information towards specific goal or objective. In this regard, effective adaption of AI is very important with the usage of some advance technology which can integrate the human thought with some specific outcomes. Additionally, the feature of learning climate defines the overall organizational trends towards the adoption of new ideas and thoughts in various layers of management. Learning climate also indicates the organizational capability to adopt the changing environment to get market success and long-term success. However, the mediating effect of learning climate is an important discussion in the field of strategic management while discussing the relationship between KM and awareness about AI. This study has primarily examined this gap in the literature and develops a conceptual relationship between strategic management of organizational knowledge and its impact on awareness about AI, while mediating their relationship through learning climate. The rest of the paper is as follows. Section 2 describes the literature review of the study. Section 3 discuss the methods of the study while Section 4 covers the results and discussion. Section 5 discusses the conclusion of the study.
2. LITERATURE REVIEW
Relationship between KM and AI is widely discussed in the literature of business and management. Li, Bonn and Ye [38] have examined the awareness of AI and its impact on employee's turnover intention. Their study is conducted in empirical context with the sample of 468 employees from hotel industry. Findings through implications of structural model indicate that relationship between employee's turnover and their awareness about AI is significantly moderated by the organizational support. Meanwhile, study is significantly suggested to various employees in the field of hotel industry and similar other business. Paschen, Kietzmann and Kietzmann [39] have examined the phenomenon of AI and its relationship with the KM in Business to Business (B2B) marketing. To examine this association, their paper has developed a conceptual framework for in-depth understanding of the relationship between exogenous and indigenous variables of the study. As per findings, authors have suggested that AI is fundamental phenomenon through input-output process and further defined by six building blocks. While practical implications of the study indicates that in B2B business, there is a significant need to implement AI concept for the better management of knowledge. Additionally, their study is observed a reasonable contribution in the field of big data and KM for AI. In their study [6], have considered the decision support through KM with the role of AI in computer bases system. Authors explain that both decision support and KM are interdependent to each other in various business organizations. Meanwhile, their study has provided a good understanding of AI towards the KM. Nemati, Steiger, Iyer and Herschel [40] have observed the factor of knowledge warehouse and integration of KM through decision support and AI. Their paper has significantly proposed the idea that there are different directions of decision support system for organizational management of knowledge under the title of AI. Whereas, some study has integrated the concept of organizational learning for various mechanisms in the business-like transformational leadership. Veng Seng, Zannes and Wayne Pace [41] has examined the relationship between KM and its contribution towards workplace learning. Their work argues that learning at workplace is significant associated to the level of KM, where organizational culture and support is increasing and encourage the knowledge sharing. However, to create a learning climate, employees' perception towards learning is a significant indicator. For this purpose, [42] have empirically examine the learning climate in both public and private organizations in the region of South Africa. Their study was based on the survey and comparative analysis of the various departments while observing their learning climate. The findings of the study suggest that management support, opportunity to develop, time and organizational responsibility with the access to information are the core factors towards the learning climate across the business firms. In addition, it is revealed that learning climate could be created in different type of organizations with individual drivers. Furthermore, their article makes a reasonable contribution for learning environment and talent development in the business organizations of South Africa. Additionally, the trend of literature in the field of learning climate is also observed under range of studies, both in developed and emerging economies while taking the sample from both public and private sector organizations [43–45]. Additionally, research work conducted by authors like [46–50] have significantly focus on the economy of Saudi Arabia to examine the factor of KM and its industrial implication.
However, the literature trend is significantly providing a gap for the mediating role of learning climate between KM and awareness about AI in service firms of Saudi Arabia. Based on the above literature, following hypotheses are developed and empirically tested:
H1: More the acquisition of strategic knowledge in the business, more the awareness about AI in all three layers of management.
H2: More the dissemination of strategic knowledge in the business, more the awareness about AI in all three layers of management.
H3: More the responsiveness of strategic knowledge in the business, more the awareness about AI in all three layers of management.
H4: Learning climate mediates the relationship between strategic acquisition of knowledge (SAK) and AI awareness.
H5: Learning climate mediates the relationship between strategic dissemination of knowledge (SDK) and AI awareness.
H6: Learning climate mediates the relationship between strategic responsiveness of knowledge and AI awareness.
3. RESEARCH METHODOLOGY
The present study was conducted in the west region of Saudi Arabia, while targeting the service industry and its key employees in all three management layers. The key reason to select the service industry was that they are primarily working for organizational KM to create the awareness in their business. For the purpose of data collection, a structural questionnaire is developed, and data is collected from first, second and third layer of the management. A total sample of 390 respondents is finally collected from overall population in targeted region. The research design of current study is defined under the title of empirical research where two-dimensional hypotheses are defined. Research hypotheses from H1 to H3 are measuring the direct impact of KM and its relationship with awareness about AI. Whereas, hypotheses from H4 to H6 are indicating the mediating effect of learning climate. Additionally, this research has adopted the deductive research approach. For the development of questionnaire, five items for each of the latent variable of strategic management of organizational knowledge through acquisition, dissemination and responsiveness are added. For learning climate as key mediator, three items and for awareness about AI, five items are added in the model. All the stated items are measured on 5-point Likert scale. Figure 1 presents the structural model of the study, where SAK, SDK are added as key latent variables and exogenous factors of the study.
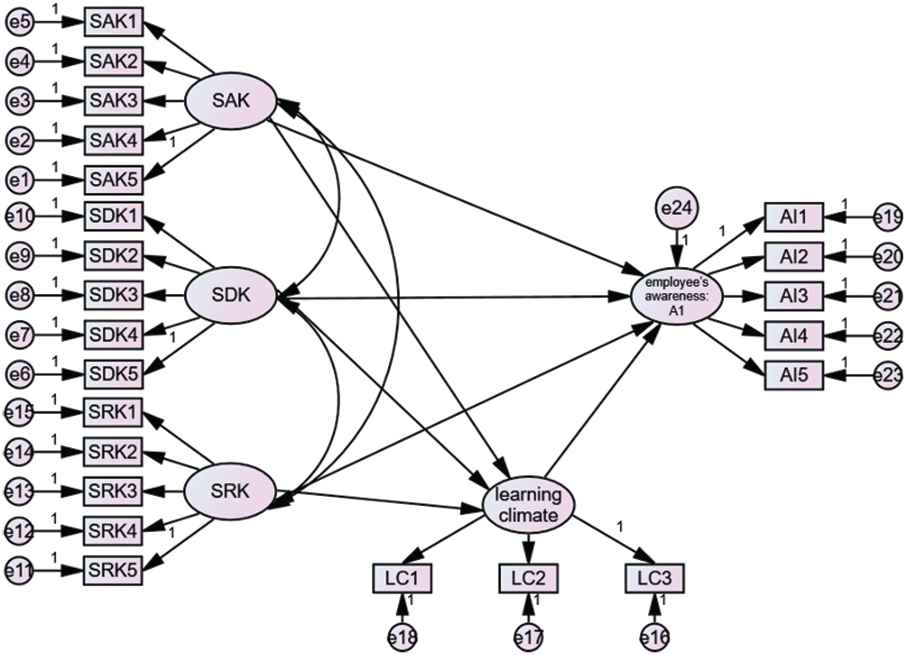
Structural model of the study.
4. RESULTS AND DISCUSSIONS
4.1. Demographic Distribution and Reliability Analysis
To conduct empirical analysis in current study, a final sample of 390 respondents is valid, out of 465, showing a percentage of 75 percent for response rate. For the managerial implications, the respondents for present study were selected from those who have managerial posts in all three layers (first level, second and bottom level as well). These employees in the business firms are directly or indirectly dealing with organizational strategic KM as acquiring, sharing and response towards organizational knowledge is controlled by all three layers of management. As per the classification of gender both male and female respondents were observed in the data collection, where 68 percent belongs to male category and 32 percent were the female's managers. It shows that there is a significant gap of male and female gender as 68 percent of the respondents are males, showing their majority position in organizational decision making and strategic management of knowledge. As per the distribution of age, 43 percent belongs to the age range of 20–28 years, 25 belongs to 29–35 years, 16 percent in range of 36–45 years and rest of the 16 percent belongs to above 45 years of age.
After the demographic distribution of respondents, next step is to examine the reliability analysis through the value of Cronbach alpha. Table 1 provides the findings for the alpha score of each of the latent variable of the study, where strategic acquisition, strategic dissemination and strategic responsiveness of the knowledge along with awareness of AI are measured through five items of each. Whereas learning climate is measured through three items. The score of alphas for each of the variable is in range of .72 to .931 respectively, where lowest score is observed for learning climate and highest for strategic responsiveness of the knowledge. As per the earlier literature, value of alpha above .70 is considered as good and above .90 is known as excellent.
Description of Latent Variables | Total Items | Value of Alpha |
---|---|---|
Strategic acquisition of knowledge | 5 | .836 |
Strategic dissemination of knowledge | 5 | .893 |
Strategic responsiveness of knowledge | 5 | .931 |
Awareness about AI | 5 | .884 |
Learning climate | 3 | .729 |
AI, artificial intelligence.
Reliability analysis.
For the factor analysis, confirmatory factor analysis (CFA) is performed with the model fit findings and factor loadings as presented in Tables 2 and 3. For model fit, Chi-square is 116.890, significant at 5 percent, showing that overall model is fit for the factor loadings of stated items as presented under Table 4. For Goodness Fit Index (GFI), value of .961, for Adjusted Goodness Fit Index (AGFI) .945, for Tucker Lewis Index (TLI) .951, for Comparative Fit Index (CFI) .966, for Parsimony Comparative Fit Index (PCFI) .809 and for Root Mean Square Error of Approximation (RMSEA) is .030. All these model fit indices have shown significant values above their specific threshold points for accepting the values of factor loadings and overall CFA technique. Based on the stated findings as presented under Table 2, all model fit values are above their cut points, providing the fact that there is no biasness in the value of factor loadings.
Description of Fit Measurement | Value Achieved | Accepted/Not Accepted |
---|---|---|
Chi-square | 116.890 | Accepted |
Probability value | .000 | Accepted |
GFI | .961 | Accepted |
AGFI | .945 | Accepted |
TLI | .959 | Accepted |
CFI | .966 | Accepted |
PCFI | .809 | Accepted |
RMSEA | .030 | Accepted |
Model fit indices.
Items | Direction | Variables | Estimate |
---|---|---|---|
SAK5 | <— | SAK | 0.822 |
SAK4 | <— | SAK | 0.836 |
SAK3 | <— | SAK | 0.914 |
SAK2 | <— | SAK | 0.936 |
SAK1 | <— | SAK | 0.883 |
SDK5 | <— | SDK | 0.917 |
SDK4 | <— | SDK | 0.883 |
SDK3 | <— | SDK | 0.946 |
SDK2 | <— | SDK | 0.782 |
SDK1 | <— | SDK | 0.861 |
SRK5 | <— | SRK | 0.842 |
SRK4 | <— | SRK | 0.856 |
SRK3 | <— | SRK | 0.913 |
SRK2 | <— | SRK | 0.926 |
SRK1 | <— | SRK | 0.847 |
AI5 | <— | AI | 0.836 |
AI4 | <— | AI | 0.914 |
AI3 | <— | AI | 0.957 |
AI2 | <— | AI | 0.785 |
AI1 | <— | AI | 0.813 |
LC3 | <— | LC | 0.917 |
LC2 | <— | LC | 0.827 |
LC1 | <— | LC | 0.880 |
AI, artificial intelligence; CFA, confirmatory factor analysis; SAK, strategic acquisition of knowledge; SDK, strategic dissemination of knowledge; SRK, Strategic Responsiveness of knowledge.
Factors loadings for each items of latent variables under CFA.
Variables | Directions | Variables | Estimate | SE | CR | P |
---|---|---|---|---|---|---|
SAK | <—> | SRK | .106 | .024 | 4.463 | *** |
SAK | <—> | SDK | .142 | .028 | 5.126 | *** |
SDK | <—> | SRK | .179 | .031 | 5.806 | *** |
SAK, strategic acquisition of knowledge; SDK, strategic dissemination of knowledge; SE, standard error; CR, Composite Reliability.
Covariances: (Group number 1—Default model).
Table 3 have provided the findings for the factor loadings of three latent variables of strategic management of knowledge, covered under the title of knowledge acquisition, knowledge dissemination and knowledge responsiveness. For awareness about AI, five indicators are added in the model. For learning climate three indicators under the title of LC1, LC2 and LC3 are observed. As per findings, all factors have shown a good value of loadings through CFA and highest value is observed for AI3, SDK3 and followed by SRK2 respectively. It means that all variables have their significant contribution in developing and testing the structural model of the study.
Table 4 presents the covariance between the variables, it is observed that all three measures of strategic management of organizational knowledge have their significant estimates of covariance with 95 percent confidence level.
Table 5 presents the outcome for direct relationship between strategic management of organizational knowledge and managers awareness about AI. For SAK, effect on AI is .300 with the standard error of .091. It means that more the acquisition of strategic knowledge in the business, more the awareness in all three layers of the business about AI. This impact accepted the research hypotheses that more the acquisition of strategic knowledge in the business, more the awareness about AI in all three layers of management. For H2, effect of SDK on AI is observed. This relationship has demonstrated a coefficient of .533 with the standard error of .235. It means that there is a positive relationship between SDK and AI under full sample of the study. This effect has a critical ratio of 2.265, significant at 5 percent level of significance. Based on significant value of the coefficient, this study has also accepted H2 that “more the dissemination of strategic knowledge in the business, more the awareness about AI in all three layers of management.” Through third latent variable under the title of strategic responsiveness of organizational knowledge, effect on AI is 1.243 with the standard error of .169. It means that there is a direct effect of SDK on AI awareness in overall organizational structure and all three management layers. This effect is also significant as CR is 7.366, at 95 percent confidence level.
Items | Directions | Variables | Estimate | SE | CR | P |
---|---|---|---|---|---|---|
A1 | <— | SAK | .300 | .091 | 3.29 | 0.000*** |
A1 | <— | SDK | .533 | .235 | 2.265 | 0.023** |
A1 | <— | SRK | 1.243 | .169 | 7.366 | 0.000*** |
SAK5 | <— | SAK | 1.000 | |||
SAK4 | <— | SAK | .974 | .187 | 5.213 | *** |
SAK3 | <— | SAK | 1.035 | .181 | 5.716 | *** |
SAK2 | <— | SAK | 1.271 | .222 | 5.732 | *** |
SAK1 | <— | SAK | 1.246 | .217 | 5.737 | *** |
SDK5 | <— | SDK | 1.000 | |||
SDK4 | <— | SDK | 1.188 | .150 | 7.917 | *** |
SDK3 | <— | SDK | 1.211 | .149 | 8.107 | *** |
SDK2 | <— | SDK | 1.014 | .135 | 7.512 | *** |
SDK1 | <— | SDK | 1.002 | .135 | 7.414 | *** |
SRK5 | <— | SRK | 1.000 | |||
SRK4 | <— | SRK | .813 | .081 | 10.052 | *** |
SRK3 | <— | SRK | .647 | .091 | 7.122 | *** |
SRK2 | <— | SRK | .670 | .088 | 7.586 | *** |
SRK1 | <— | SRK | .673 | .086 | 7.807 | *** |
AI1 | <— | A1 | 1.000 | |||
AI2 | <— | A1 | .957 | .091 | 10.503 | *** |
AI3 | <— | A1 | .832 | .089 | 9.383 | *** |
AI4 | <— | A1 | .650 | .086 | 7.543 | *** |
AI5 | <— | A1 | .744 | .082 | 9.103 | *** |
AI, artificial intelligence; SAK, strategic acquisition of knowledge; SDK, strategic dissemination of knowledge; SE, standard error; SMK, Strategic Management of knowledge.
Regression weights: Direct effect of SMK on awareness about AI.
4.2. Mediating Effect of LC Between SAK and Awareness About AI
After the analysis of direction relationship between strategic management of knowledge and awareness about AI, next step is to examine the effect of each of the three factors through mediation process while taking the LC as main mediator for AI. Figure 2 provides a structural relationship between SAK, LC and AI for the whole sample of the study. The factor of learning climate LC has three items named as LC1, LC2 and LC3. Findings are presented under Table 6. The effect of SAK on AI is positively significant with the coefficient of .446 and standard error of 3.269. It means that SAK has a direct and significant effect on AI. While the coefficient of SAK on LC is positively significant and through LC to AI is also significant. It means that there is a partial mediation between SAK and AI through LC.

Mediating effect of LC between SAK and AI. AI, artificial intelligence; SAK, strategic acquisition of knowledge.
Variables/Items | Directions | Variables | Estimate | SE | CR | P |
---|---|---|---|---|---|---|
LC | <— | SAK | .182 | .088 | 2.06 | .021** |
AI | <— | SAK | .446 | .137 | 3.269 | .001** |
AI | <— | LC | −.311 | .140 | −2.215 | .027** |
SAK5 | <— | SAK | 1.000 | |||
SAK4 | <— | SAK | 1.067 | .218 | 4.893 | *** |
SAK3 | <— | SAK | 1.163 | .221 | 5.253 | *** |
SAK2 | <— | SAK | 1.190 | .240 | 4.966 | *** |
SAK1 | <— | SAK | 1.202 | .239 | 5.029 | *** |
AI1 | <— | AI | 1.000 | |||
AI2 | <— | AI | .904 | .107 | 8.438 | *** |
AI3 | <— | AI | .966 | .110 | 8.775 | *** |
AI4 | <— | AI | .685 | .100 | 6.867 | *** |
AI5 | <— | AI | .867 | .101 | 8.616 | *** |
LC3 | <— | LC | 1.000 | |||
LC2 | <— | LC | 1.238 | .277 | 4.468 | *** |
LC1 | <— | LC | 1.408 | .328 | 4.294 | *** |
R-Square | 0.6214 | |||||
Adjusted R-Square | 0.6087 | |||||
F-Value | 58.391 | |||||
P-value | 0.000*** |
AI, artificial intelligence; SAK, strategic acquisition of knowledge; SE, standard error.
Regression weights: Mediation effect of LC between SAK and AI.
For second latent variable of strategic management of organizational knowledge (SDK), mediation effect through LC between SDK and AI is empirically tested. Figure 3 presents the structure of this relationship where SDK has five dimensions, AI has five dimensions and LC has three dimensions. Findings for the mediating effect of LC between SDK and AI are presented under Table 7.
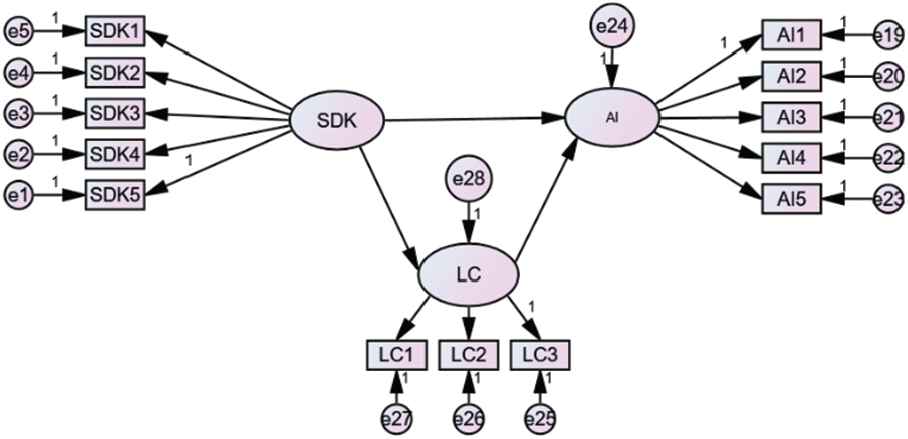
Mediating effect of LC between SDK and AI. AI, artificial intelligence; SDK, strategic dissemination of knowledge.
Variables/Items | Directions | Variables | Estimate | SE | CR | P |
---|---|---|---|---|---|---|
LC | <— | SDK | .381 | .064 | 5.953 | 0.000*** |
AI | <— | SDK | .472 | .103 | 4.597 | 0.000*** |
AI | <— | LC | −.331 | .142 | −2.337 | 0.019** |
SDK5 | <— | SDK | 1.000 | |||
SDK4 | <— | SDK | 1.125 | .158 | 7.130 | *** |
SDK3 | <— | SDK | 1.312 | .171 | 7.687 | *** |
SDK2 | <— | SDK | 1.075 | .149 | 7.202 | *** |
SDK1 | <— | SDK | 1.003 | .145 | 6.904 | *** |
AI1 | <— | AI | 1.000 | |||
AI2 | <— | AI | .901 | .104 | 8.703 | *** |
AI3 | <— | AI | .936 | .105 | 8.906 | *** |
AI4 | <— | AI | .656 | .096 | 6.827 | *** |
AI5 | <— | AI | .830 | .096 | 8.667 | *** |
LC3 | <— | LC | 1.000 | |||
LC2 | <— | LC | 1.257 | .282 | 4.461 | *** |
LC1 | <— | LC | 1.401 | .325 | 4.306 | *** |
R-Square | 0.491 | |||||
Adjusted R-Square | 0.486 | |||||
F-Value | 68.391 | |||||
P-Value | 0.000*** |
AI, artificial intelligence; SDK, strategic dissemination of knowledge.
Regression weights: Mediation effect of LC between SDK and AI.
The effect of SDK on AI is .472 with the standard error of .103 and critical ratio of 4.597, which indicates that there is significant direct effect of SDK on AI for full sample of the study. For SDK on LC, coefficient is .381 and for LC to AI, it is −.331, significant at 5 percent. It means that there is a direct as well as indirect effect of LC as key mediator on AI This effect presents the fact that again the outcomes are in favor for the partial mediation.
Figure 4 presents the structural model for mediation effect of LC between SRK and AI under full sample consideration. The effect of SRK on AI is.856 with the standard error of .088. It means that there is a significant and positive influence of SRK on AI in all management layers. For mediation effect, when SRK approaches to LC, its coefficient is −.139, significant at 5 percent. However, its path through LC to AI is found to be insignificant at CR is .770, providing the evidence that there is no significant mediation between SRK and AI through LC. This effect implies the fact LC as a core mediator provides its significant role while dealing with the SAK and AI, and SDK and AI. However, for the third latent indicator of strategic management of organizational knowledge, no mediation is observed (Table 8).
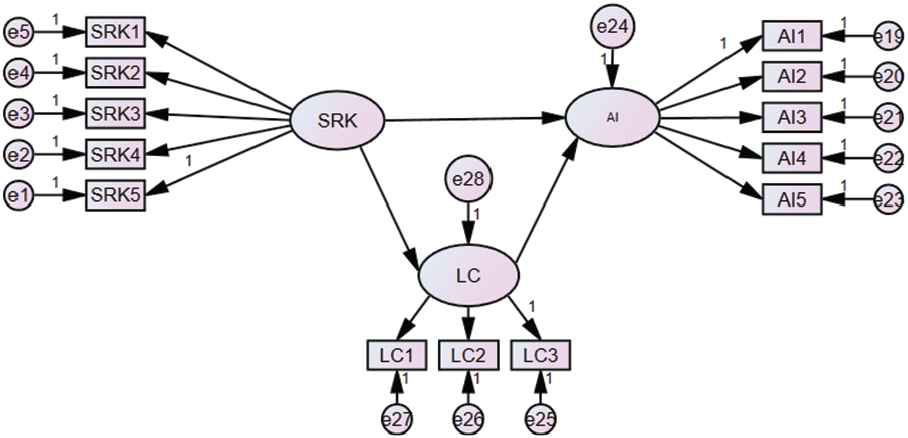
Mediating effect of LC between SRK and AI. AI, artificial intelligence.
Items | Directions | Variables | Estimate | SE | CR | P |
---|---|---|---|---|---|---|
LC | <— | SRK | −.139 | .050 | −2.762 | .006*** |
AI | <— | SRK | .856 | .088 | 9.763 | *** |
AI | <— | LC | .034 | .115 | .292 | 0.770 |
SRK5 | <— | SRK | 1.000 | |||
SRK4 | <— | SRK | .755 | .072 | 10.489 | *** |
SRK3 | <— | SRK | .528 | .082 | 6.437 | *** |
SRK2 | <— | SRK | .536 | .080 | 6.743 | *** |
SRK1 | <— | SRK | .504 | .077 | 6.523 | *** |
AI1 | <— | AI | 1.000 | |||
AI2 | <— | AI | .964 | .091 | 10.601 | *** |
AI3 | <— | AI | .826 | .088 | 9.356 | *** |
AI4 | <— | AI | .648 | .086 | 7.531 | *** |
AI5 | <— | AI | .747 | .082 | 9.161 | *** |
LC3 | <— | LC | 1.000 | |||
LC2 | <— | LC | 1.212 | .270 | 4.485 | *** |
LC1 | <— | LC | 1.326 | .302 | 4.387 | *** |
R-Square | 0.437 | |||||
Adjusted R-Square | 0.405 | |||||
F-Value | 65.371 | |||||
P-value | 0.000*** |
AI, artificial intelligence.
Regression weights: Mediation effect of LC between SRK and AI.
5. CONCLUSION AND RECOMMENDATIONS
The current study has focused on developing the conceptual and empirical understanding through relationship between strategic management of organizational knowledge, learning climate and awareness about AI. Based on the structural equation modeling, and mediating effect of learning climate on the relationship between strategic management of organizational knowledge and awareness about AI, this research has contributed significantly in the present literature. In the first step, data is collected through questionnaire and interview techniques. In the next step, factor analysis were applied, showing a good loading for all the items of each of the latent variable of the study. After CFA, SEM model is developed to examine the relationship between strategic management of organizational knowledge as measured through knowledge acquisition, knowledge dissemination and knowledge responsiveness under five items for each. For AI five items are also added in the model. Findings through SEM approaches shows that SAK, SDK and SRK have their significant and positive relationship with AI under full sample of the study. For mediation, three sperate mediation models are developed, showing the relationship between SAK, LC and AI, between SDK, LC and AI, and between SRK, LC and AI of the study. It is found that between SAK and IA and between SDK and IA, partial and significant medication is presented due to LC. However, between SRK and IA, no significant mediation is found between SRK and IA through LC. As per theoretical and managerial implications, this study has significantly contributed while providing a new addition in the field of strategic management of knowledge, learning climate as key mediator and awareness about AI. In addition, practical implications of this study reveals the fact that management in all three layers of the business firms should consider these findings and positive role by learning climate to promote awareness about AI. However, this study has observed several limitations. At first overall impact of strategic management of knowledge is yet to be explored as this study has examined its impact through knowledge acquisition, dissemination and responsiveness factors under sperate models. At second, study has a regional limitations and specific organizational implications only for the service sector is observed. Future studies could be reimplemented on similar topic while addressing these limitations.
CONFLICT OF INTEREST
There is no conflict of interest between authors and with other stake holders.
AUTHORS' CONTRIBUTIONS
All the authors have equally contributed in this research.
ACKNOWLEDGMENTS
We thanks to Business school, Hunan University, Changsha, P.R. China and Ministry of Education, Riyadh, Kingdom of Saudi Arabia for the support of this research.
REFERENCES
Cite this article
TY - JOUR AU - Mohammed Majdy M. Baslom AU - Shu Tong PY - 2019 DA - 2019/11/12 TI - Strategic Management of Organizational Knowledge and Employee's Awareness About Artificial Intelligence With Mediating Effect of Learning Climate JO - International Journal of Computational Intelligence Systems SP - 1585 EP - 1591 VL - 12 IS - 2 SN - 1875-6883 UR - https://doi.org/10.2991/ijcis.d.191025.002 DO - 10.2991/ijcis.d.191025.002 ID - Baslom2019 ER -