Exploratory Analysis of Demographic Factors and the Temporal Evolution of COVID-19 in India
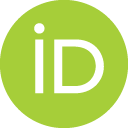
- DOI
- 10.2991/jegh.k.200921.001How to use a DOI?
- Keywords
- COVID-19; population and urbanization; tobacco and epidemics; smoking and smokeless tobacco products (SLT); tobacco harm reduction
- Copyright
- © 2020 The Authors. Published by Atlantis Press International B.V.
- Open Access
- This is an open access article distributed under the CC BY-NC 4.0 license (http://creativecommons.org/licenses/by-nc/4.0/).
1. INTRODUCTION
With more than 7 million cases and approaching half a million deaths, the global impact of 2019-nCoV popularly known as COVID-19 remains uncertain. Low and middle income countries including India have witnessed exponential urbanization and migration with complex patterns across urban–rural landscapes. These population shifts bring along behavioral and substance use challenges during pandemics. The associated migration patterns are dynamically changing at the time of writing this paper depending on advice from local authorities and emerging scientific evidence. For example, COVID-19 is now suspected to be airborne as contested by a group of scientists and the World Health Organization (WHO). Therefore, it is expected that urbanized areas with high population densities would lead to a much faster spread of the virus.
Several countries including India, South Africa and Botswana had banned sale of alcohol and/or tobacco during imposed lockdowns to curb social gatherings and public contact, while supplementary addressing domestic violence and abuse. Although alcohol in limited quantity is not expected to adversely affect infection or recovery from COVID-19 which attacks the respiratory system, the toxicity of inhaled carcinogens from smoking or other forms of tobacco may affect immune response presenting an impending health crisis that could intensify respiratory infection. Even before COVID-19, tobacco use was directly correlated as the number one cause of mortality from oral cancer for which India is among the worst affected globally. The authors did not find any peer-reviewed studies that have evaluated the risk of SARS-CoV-2 infection among smokers. However, there is epidemiological evidence [1] that smoking increases the risk of viral lung and throat infections. The WHO has recently published a review by public health experts concluding that smokers are more likely to develop severe disease with COVID-19, compared with non-smokers [2]. The increased risk stems from the fact that smoking suppresses immune function and inflames the lungs and throat. Additionally, substance use is characterized by inhalation patterns and repetitive hand-to-mouth movements which are strongly advised against to reduce viral contamination.
Several demographic and geographic factors in addition to prognostic factors including underlying health conditions and age, environmental factors including pollution, temperature and humidity can assist modeling studies in providing vital insights on COVID-19. These factors can be used in future mathematical models [3,4] for predicting the reproductive number (R) and case fatality rate for different geographies. The current exploratory analysis studied the dynamic relation between population density, urbanization and tobacco prevalence on the spread and recovery rate associated with COVID-19 for Indian States.
2. MATERIALS AND METHODS
The authors obtained data for total COVID-19 cases and number of patients recovered for all Indian States from the Government of India portal as reported for the pandemic until 5 June and until 18 July [5]. Population and areas (km2) for States were obtained from the central government database of India [6] which were used to calculate population densities. The data on number of tobacco users in India including smoking and smokeless tobacco were obtained from the Global Adult Tobacco Survey published by the WHO [7]. Urbanization for all States were obtained from the data on population census provided by the Government of India [8]. The descriptive statistics for cumulative data at either timepoints is illustrated in Table 1. The authors performed linear regression and correlation analysis using the statistical package R integrated in the Rstudio environment (Version 1.0.153 – © 2009–2017 RStudio, Inc., Boston, Massachusetts). The Spearman’s rank correlation coefficient was used for statistical analysis since the data did not represent a normal distribution as shown with margin plots. The statistical parameters including the p-value, Confidence Intervals (CI) and rho (ρ) are reported in the figure captions with confidence level and level of significance set at 95% and 5%, respectively. Additionally, an Exploratory Factor Analysis (EFA) was not pursued since the number of variables and items per variable were insufficient. Further, the KMO-Criteria (Kaiser-Meyer-Olkin Test of Sampling Adequacy) for datasets from either time points were obtained as 0.48 and 0.56 indicating that the data is not adequate for further factoring, and additional variables and/or sample points are needed before undertaking any further EFA analysis. The assumptions for the analysis are the dynamically changing influence of several factors including lockdown strategies and social distancing guidelines by local and central governments. Abbreviations used for all States can be found from the Government of India source: https://slusi.dacnet.nic.in/watershedatlas/list_of_state_abbreviation.htm.
min | max | mean | sd | median | skew | kurtosis | se | |
---|---|---|---|---|---|---|---|---|
1a) 5 June | ||||||||
Recovery rate (%) | 0.00 | 86.64 | 44.05 | 21.94 | 44.72 | –0.41 | –0.40 | 4.07 |
Cases per 1000 | 0.001 | 0.74 | 0.11 | 0.17 | 0.05 | 2.44 | 5.40 | 0.03 |
GATS2 Tobacco use | 9.70 | 64.50 | 31.57 | 14.61 | 26.50 | 0.52 | –0.80 | 2.71 |
Population density | 18.75 | 1325.36 | 406.69 | 326.42 | 330.76 | 1.23 | 0.77 | 60.61 |
Urbanization | 10.03 | 62.17 | 30.81 | 12.45 | 28.86 | 0.52 | –0.27 | 2.31 |
1b) 18 July | ||||||||
Recovery rate (%) | 16.38 | 75.48 | 58.43 | 15.13 | 61.98 | –1.04 | 0.25 | 2.81 |
Cases per 1000 | 0.12 | 2.38 | 0.63 | 0.60 | 0.39 | 1.75 | 2.11 | 0.11 |
Descriptive statistics for recovery rate and spread of COVID-19 in India at two timepoints, (a) 5 June 2020 and (b) 18 July 2020 shown with tobacco use, population density and urbanization for Indian states (n = 29)
3. RESULTS AND DISCUSSION
This study included analysis of data obtained from official Government of India sources on population, urbanization, prevalence of smoking and smokeless tobacco, number of COVID-19 cases, and the number of patients recovered from COVID-19 for all 29 States in India. The descriptive statistics including min, max, mean, Standard Deviation (SD), median, kurtosis, skewness and Standard Error (SE) is reported in Table 1 for all variables at both timepoints. The kurtosis and skewness indicate non-normality in the data distribution for both timepoints justifying the use of Spearman correlation. The data on number of COVID-19 cases per 1000 (spread) and percentage of patients recovered from COVID-19 were calculated based on the original data which is appended with this publication. A significant negative correlation was obtained between tobacco prevalence and recovery rate from COVID-19 at the regional level as illustrated in Figure 1. Population density significantly correlated with spread as well as the recovery rate for available cumulative data until 5 June. However, the recovery rate did not correlate with either tobacco prevalence, urbanization, or population density for the latest cumulative data until 18 July illustrated by Figure 2. Additionally, only urbanization correlated with the spread of Covid-19 for the latest data until 18 July.
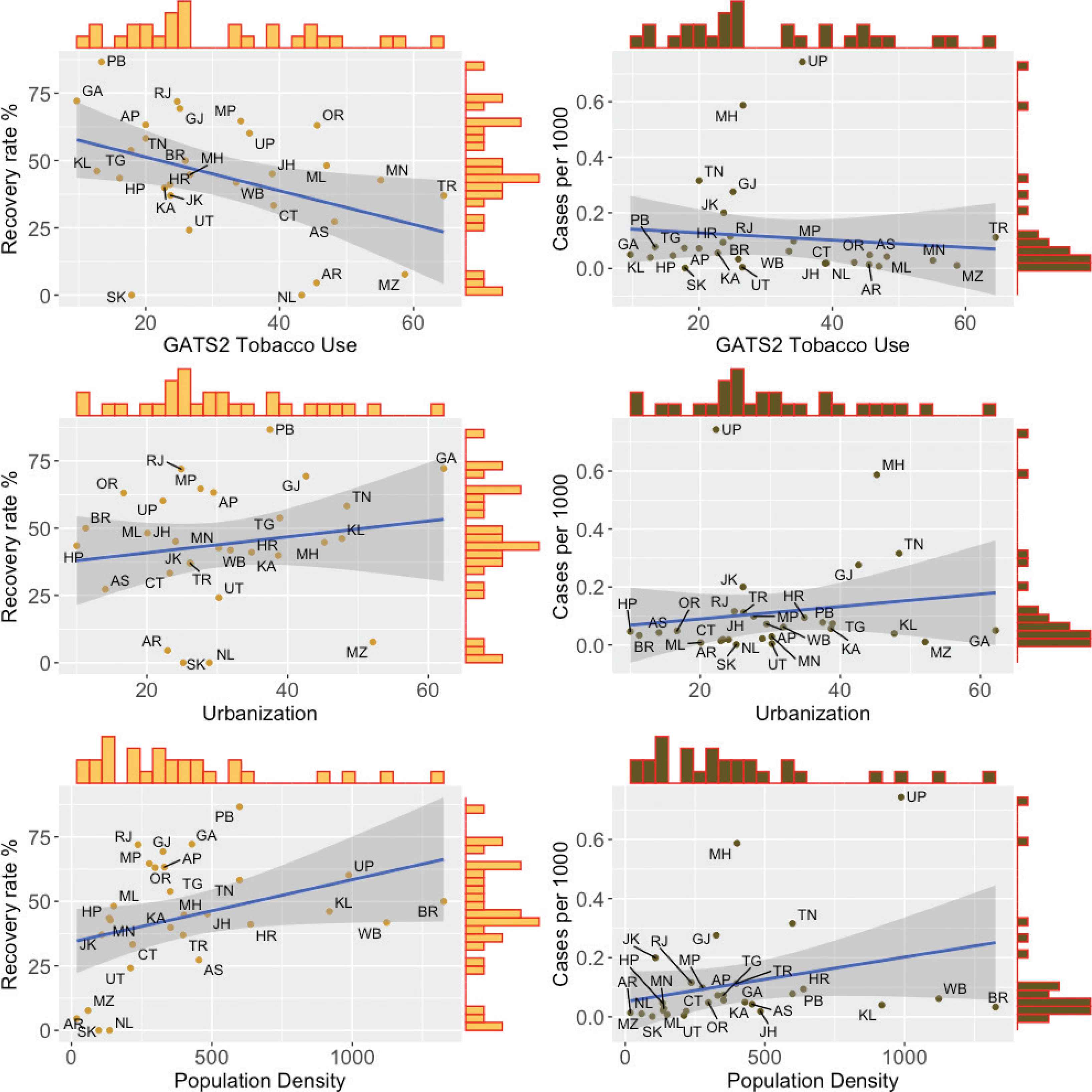
Correlation between tobacco use, urbanization (%) and population density, and the number of COVID-19 cases per 1000 people for India shown on the right. Whereas correlations between the same factors and the recovery rate from COVID-19 are shown on the left. These data represent cumulative COVID-19 data until 5 June 2020. Tobacco use (p = 0.026; R = −0.41; CI = −0.76, −0.06) and population density (p = 0.015; R = 0.44; CI = 0.09, 0.78) showed a significant correlation with the recovery rate, whereas population density (p = 0.017; R = 0.44; CI = 0.07, 0.80) also correlated to the number of cases per 1000.
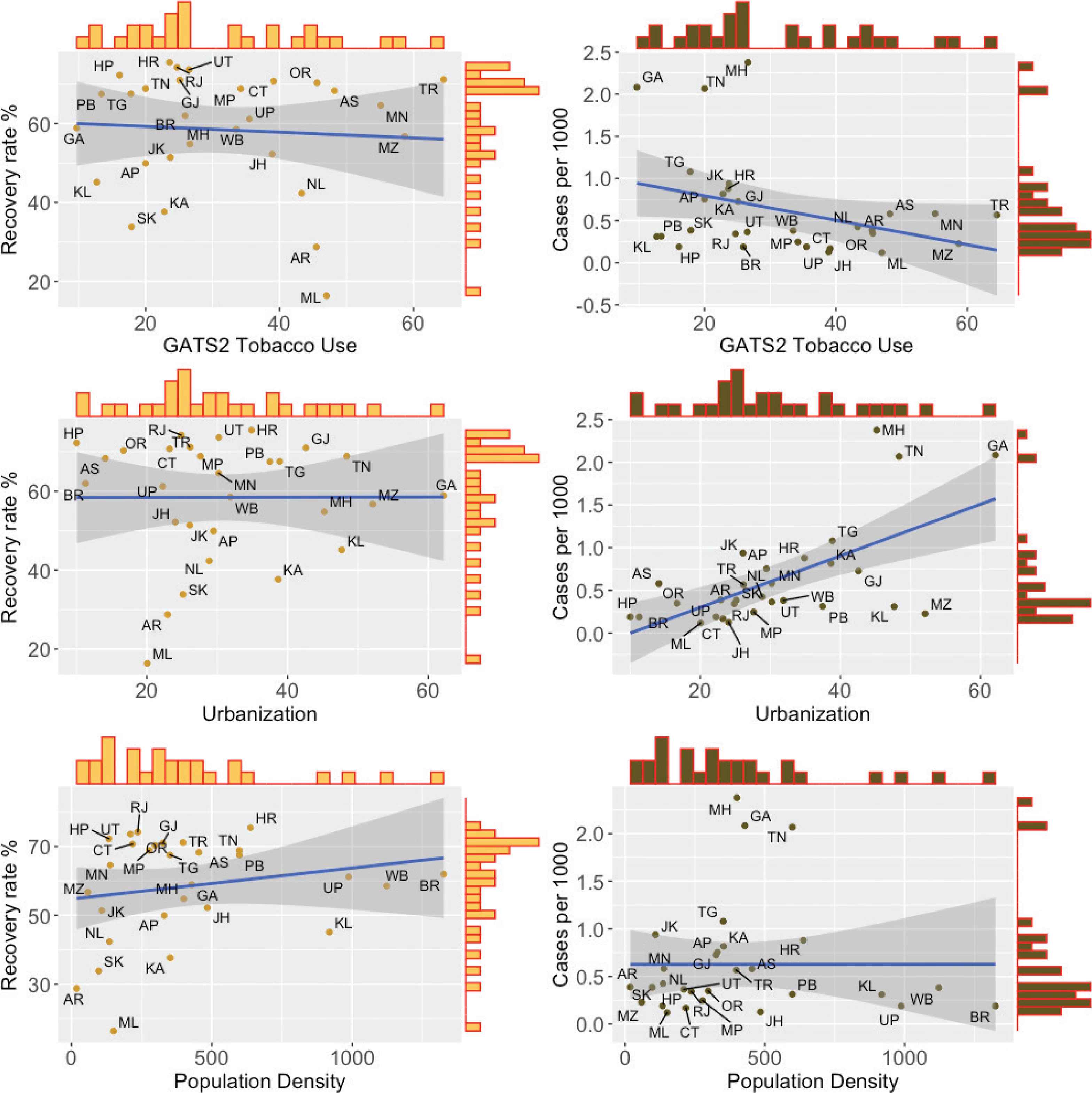
The same plots as shown in Figure 1 for cumulative COVID-19 data until 18 July 2020 are illustrated above. Urbanization showed a statistically significant correlation with number of cases per 1000 (p = 0.0017, R = 0.56, CI = 0.23, 0.89), whereas none of the correlations from Figure 1 were significant for the latest cumulative data.
The combination of substance use including smoking, vaping and opioid with the ongoing COVID-19 presents a direct increase in risk due to the respiratory nature of the infection as presented in a recent article by Volkow [9]. The upregulation associated with smoking of two different virus receptors observed with two different coronaviruses suggests that smoking contributes to the higher number of viral receptors and may support the findings of the recent case series observations by the WHO. DPP4 mRNA and protein expressions are significantly higher in smokers compared with never smokers without airflow limitation and are inversely correlated with lung function [10]. It has recently been reported that ACE2 gene expression is higher in ever smokers (both current and former) compared with never smokers in normal lung tissue in a sample of patients with lung adenocarcinoma, after adjustment for age, gender, and ethnicity. ACE2 gene expression was also higher in small and large airway epithelia of healthy ever smokers compared with never smokers: current smokers had the highest expression, never smokers had the lowest expression; recent former smokers (≤15 years) had higher ACE2 gene expression than non-smokers but not long-term former smokers (>15 years) [11]. Further, the meta-analysis by Emami et al. [12] analysed data from observational studies for 2986 patients and found a pooled prevalence of smoking of 7.6% (3.8–12.4%) while the recent systematic review [13] by Nikitara identified five studies and concluded that “smoking is most likely associated with negative progression and adverse outcomes of COVID-19.” These findings support the need for studies on substance use demographics both at the patient level and at the population prevalence levels.
Limitations of the current study include urbanization data from the 2011 census of population in India with the next upcoming census scheduled for 2021. Tobacco prevalence data used in this study is from a 2016 WHO report whereas population data is from 2019. India has witnessed high urbanization in the last two decades and the urban-rural landscape is witnessing complex population shifts influenced by several factors including availability of jobs and urban transportation infrastructure in addition to language and cultural factors. Further, COVID-19 numbers reported in different states in India might seem inaccurate due to inefficient centralized healthcare records and inadequate internet infrastructure especially in rural areas. Cohort studies are urgently needed at the patient level to study associated morbidity and mortality risks from substance use including alcohol and tobacco prevalence as more data becomes available for India and rest of the World. The authors will continue to pursue further exploratory analysis at regular intervals tracking the spread and recovery rates of COVID-19 in India and studying its relation with several underlying demographic and geographic factors. These findings can assist in healthcare strategy and planning for the current COVID-19 pandemic as well as future health crises.
In conclusion population density, urbanization and behavioral characteristics including substance use can be vital during lockdowns and for deploying additional healthcare facilities. Our findings suggest that highly urbanized states including Maharashtra, Gujarat and Tamil Nadu are vulnerable during the current pandemic with incoming data from India further showing that hyper-urbanized cities like Mumbai and Delhi are hit hardest, requiring additional health infrastructure sustainability amid challenges in the social environment. The ongoing pandemic has resulted in a dynamic evolution of these factors as a response to government regulations leading to complex migration patterns which bring together unique behavioral and socio-economic challenges.
CONFLICTS OF INTEREST
The authors declare they have no conflicts of interest.
AUTHORS’ CONTRIBUTION
SV analyzed the data and drafted the manuscript. VP assisted in drafting sections of the introduction and discussion. NJ assisted in editing and reviewing the manuscript.
FUNDING
No financial support was provided.
ACKNOWLEDGMENTS
The authors would like to thank Prof. Ayumi Shintani (Osaka-City University, Japan) for advice on the statistical methods used in this study. The authors would also like to thank Shweta Wani, Shilpa Gupta, Ramu Venkatesan, Reena Jhamthani, and Francie Patel for their continued support during this study.
REFERENCES
Cite this article
TY - JOUR AU - Samir Vinchurkar AU - Nilesh Jain AU - Vikas Punamiya PY - 2020 DA - 2020/09/26 TI - Exploratory Analysis of Demographic Factors and the Temporal Evolution of COVID-19 in India JO - Journal of Epidemiology and Global Health SP - 10 EP - 14 VL - 11 IS - 1 SN - 2210-6014 UR - https://doi.org/10.2991/jegh.k.200921.001 DO - 10.2991/jegh.k.200921.001 ID - Vinchurkar2020 ER -