Detecting Objects from No-Object Regions: A Context-Based Data Augmentation for Object Detection
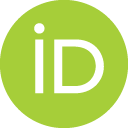
- DOI
- 10.2991/ijcis.d.210622.003How to use a DOI?
- Keywords
- Data augmentation; Object detection; Convolutional neural network; Deep learning
- Abstract
Data augmentation is an important technique to improve the performance of deep learning models in many vision tasks such as object detection. Recently, some works proposed the copy-paste method, which augments training dataset by copying foreground objects and pasting them on background images. By designing a learning-based context model to predict realistic placement regions, these approaches have been proved to be more effective than traditional data augmentation methods. However, the performance of the existing context model was limited by three problems: (1) The definitions of positive and negative samples generate too much label noise. (2) The examples with masked regions lose a lot of context information. (3) The sizes (i.e., scale and aspect ratios) of predicted regions are sampled from a prior shape distribution, which leads to a coarse estimation. In this work, we first explore the placement rules that generate realism and effective training examples for detectors. And then, we propose a trainable context model in order to find proper placement regions by classifying and refining dense prior default boxes. We also design a corresponding reasonable generation for training examples by annotating ground truth on free space according to the placement rules. The experimental results on PASCAL VOC show that our approach outperforms the state-of-the-art- related work.
- Copyright
- © 2021 The Authors. Published by Atlantis Press B.V.
- Open Access
- This is an open access article distributed under the CC BY-NC 4.0 license (http://creativecommons.org/licenses/by-nc/4.0/).
Download article (PDF)
View full text (HTML)
Cite this article
TY - JOUR AU - Jun Zhang AU - Feiteng Han AU - Yutong Chun AU - Kangwei Liu AU - Wang Chen PY - 2021 DA - 2021/06/28 TI - Detecting Objects from No-Object Regions: A Context-Based Data Augmentation for Object Detection JO - International Journal of Computational Intelligence Systems SP - 1871 EP - 1879 VL - 14 IS - 1 SN - 1875-6883 UR - https://doi.org/10.2991/ijcis.d.210622.003 DO - 10.2991/ijcis.d.210622.003 ID - Zhang2021 ER -