Wood Species Recognition with Small Data: A Deep Learning Approach
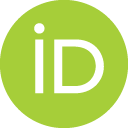
- DOI
- 10.2991/ijcis.d.210423.001How to use a DOI?
- Keywords
- Wood recognition; Transfer learning; Generalization performance; Feature extraction; ResNet50; Linear discriminant analysis; KNN
- Abstract
Wood species recognition is an important work in the wood trade and wood commercial activities. Although many recognition methods were presented in recent years, the existing wood species recognition methods mainly use shallow recognition models with low accuracy and are still unsatisfying for many real-world applications. Besides, their generalization ability is not strong. In this paper, a novel deep-learning-based wood species recognition method was proposed, which improved the accuracy and generalization greatly. The method uses 20X amplifying glass to acquire wood images, extracts the image features with ResNet50 neural network, refines the features with linear discriminant analysis (LDA), and recognizes the wood species with a KNN classifier. Our data was small, but we adopted transfer learning to improve our method. About 3000 wood images were used in our wood species recognition experiments and our method was executed in 25 rare wood species and the results showed our method had better generalization performance and accuracy. Compared with traditional deep learning our results were obtained from a small amount of data, which just confirmed the effectiveness of our method.
- Copyright
- © 2021 The Authors. Published by Atlantis Press B.V.
- Open Access
- This is an open access article distributed under the CC BY-NC 4.0 license (http://creativecommons.org/licenses/by-nc/4.0/).
Download article (PDF)
View full text (HTML)
Cite this article
TY - JOUR AU - Yongke Sun AU - Qizhao Lin AU - Xin He AU - Youjie Zhao AU - Fei Dai AU - Jian Qiu AU - Yong Cao PY - 2021 DA - 2021/04/30 TI - Wood Species Recognition with Small Data: A Deep Learning Approach JO - International Journal of Computational Intelligence Systems SP - 1451 EP - 1460 VL - 14 IS - 1 SN - 1875-6883 UR - https://doi.org/10.2991/ijcis.d.210423.001 DO - 10.2991/ijcis.d.210423.001 ID - Sun2021 ER -