Rumor Detection by Propagation Embedding Based on Graph Convolutional Network
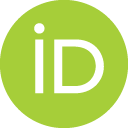
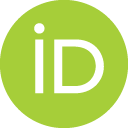
- DOI
- 10.2991/ijcis.d.210304.002How to use a DOI?
- Keywords
- Rumor detection; Propagation embedding; Graph convolutional network; Feature aggregation
- Abstract
Detecting rumors is an important task in preventing the dissemination of false knowledge within social networks. When a post is propagated in a social network, it typically contains four types of information: i) social interactions, ii) time of publishing, iii) content, and iv) propagation structure. Nonetheless, these information have not been exploited and combined efficiently to distinguish rumors in previous studies. In this research, we propose to detect a rumor post by identifying characteristics based on its propagation patterns and other kinds of information. For the propagation pattern, we suggest using a graph structure to model how a post propagates in social networks, allowing useful knowledge to be derived about a post's pattern of propagation. We then propose a propagation graph embedding method based on a graph convolutional network to learn an embedding vector, representing the propagation pattern and other features of posts in a propagation process. Finally, we classify the learned embedding vectors to different types of rumors by applying a fully connected neural network. Experimental results illustrate that our approach reduces the error of detection by approximately 10% compared with state-of-the-art models. This enhancement proves that the proposed model is efficient on extracting and integrating useful features for discriminating the propagation patterns.
- Copyright
- © 2021 The Authors. Published by Atlantis Press B.V.
- Open Access
- This is an open access article distributed under the CC BY-NC 4.0 license (http://creativecommons.org/licenses/by-nc/4.0/).
Download article (PDF)
View full text (HTML)
Cite this article
TY - JOUR AU - Dang Thinh Vu AU - Jason J. Jung PY - 2021 DA - 2021/03/16 TI - Rumor Detection by Propagation Embedding Based on Graph Convolutional Network JO - International Journal of Computational Intelligence Systems SP - 1053 EP - 1065 VL - 14 IS - 1 SN - 1875-6883 UR - https://doi.org/10.2991/ijcis.d.210304.002 DO - 10.2991/ijcis.d.210304.002 ID - Vu2021 ER -