Dictionary Learning Approach to Monitoring of Wind Turbine Drivetrain Bearings
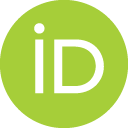
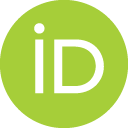
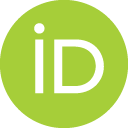
- DOI
- 10.2991/ijcis.d.201105.001How to use a DOI?
- Keywords
- Wind turbine; Condition monitoring; Dictionary learning; Feature extraction; Bearings
- Abstract
Condition monitoring is central to the efficient operation of wind farms due to the challenging operating conditions, rapid technology development, and a large number of aging wind turbines. In particular, predictive maintenance planning requires the early detection of faults with few false positives. Achieving this type of detection is a challenging problem due to the complex and weak signatures of some faults, particularly the faults that occur on the gearbox bearings of a turbine drivetrain. The results of former studies addressing condition-monitoring tasks using dictionary learning indicate that unsupervised feature learning is useful for diagnosis and anomaly detection purposes. However, these studies are based on small sets of labeled data from test rigs operating under controlled conditions that focus on classification tasks, which are useful for quantitative method comparisons but gives little insight into how useful these approaches are in practice or how can be used by existing condition-monitoring systems. Here, we investigate an unsupervised dictionary learning method for condition monitoring using vibration data recorded over 46 months under typical industrial operations. Thus, we contribute real-world industrial vibration data that are made publicly available and novel test results. In this study, dictionaries are learned from gearbox vibrations in six different turbines, and the dictionaries are subsequently propagated over a few years of monitoring data when faults are known to occur. We perform the experiment using two different sparse coding algorithms to investigate if the algorithm selected affects the features of abnormal conditions. We propose a dictionary distance metric derived from the dictionary learning process as a condition indicator and find the time periods of abnormal dictionary adaptation starting six months before a drivetrain bearing replacement and one year before the resulting gearbox replacement. In addition, we investigate the distance between dictionaries learned from geographically close turbines of the same type under healthy conditions. We find that the features learned are similar and that a dictionary learned from one turbine can be useful for monitoring a similar turbine.
- Copyright
- © 2021 The Authors. Published by Atlantis Press B.V.
- Open Access
- This is an open access article distributed under the CC BY-NC 4.0 license (http://creativecommons.org/licenses/by-nc/4.0/).
Download article (PDF)
View full text (HTML)
Cite this article
TY - JOUR AU - Sergio Martin-del-Campo AU - Fredrik Sandin AU - Daniel Strömbergsson PY - 2020 DA - 2020/11/12 TI - Dictionary Learning Approach to Monitoring of Wind Turbine Drivetrain Bearings JO - International Journal of Computational Intelligence Systems SP - 106 EP - 121 VL - 14 IS - 1 SN - 1875-6883 UR - https://doi.org/10.2991/ijcis.d.201105.001 DO - 10.2991/ijcis.d.201105.001 ID - Martin-del-Campo2020 ER -