Woodland Labeling in Chenzhou, China, via Deep Learning Approach
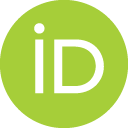
- DOI
- 10.2991/ijcis.d.200910.001How to use a DOI?
- Keywords
- Woodland labeling; Convolutional network; Deep learning; Dense fully convolutional network (DFCN)
- Abstract
In order to complete the task of the woodland census in Chenzhou, China, this paper carries out a remote sensing survey on the terrain of this area to produce a data set, and used deep learning methods to label the woodland. There are two main improvements in our paper: Firstly, this paper comparatively analyzes the semantic segmentation effects of different deep learning models on remote sensing image datasets in Chenzhou. Secondly, this paper proposed a dense fully convolutional network (DFCN) which combines dense network with FCN model and achieves good semantic segmentation effect. DFCN method is used to label the woodland in Gaofen-2 (GF-2) remote sensing images in Chenzhou. Under the same experimental conditions, the labeling results are compared with the original FCN, SegNet, dilated convolutional network, and so on. In these experiments, the global pixel accuracy of DFCN is 91.5%, and the prediction accuracy of the “woodland” class is 93%, both of them perform better than that of the other methods. In other indicators, our method also has better performance. Using the method of this paper, we have completed the land feature labeling of Chenzhou area and provided it to customers.
- Copyright
- © 2020 The Authors. Published by Atlantis Press B.V.
- Open Access
- This is an open access article distributed under the CC BY-NC 4.0 license (http://creativecommons.org/licenses/by-nc/4.0/).
Download article (PDF)
View full text (HTML)
Cite this article
TY - JOUR AU - Wei Wang AU - Yujing Yang AU - Ji Li AU - Yongle Hu AU - Yanhong Luo AU - Xin Wang PY - 2020 DA - 2020/09/16 TI - Woodland Labeling in Chenzhou, China, via Deep Learning Approach JO - International Journal of Computational Intelligence Systems SP - 1393 EP - 1403 VL - 13 IS - 1 SN - 1875-6883 UR - https://doi.org/10.2991/ijcis.d.200910.001 DO - 10.2991/ijcis.d.200910.001 ID - Wang2020 ER -