Automated Recognition of Hand Grasps Using Electromyography Signal Based on LWT and DTCWT of Wavelet Energy
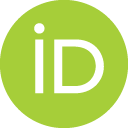
- DOI
- 10.2991/ijcis.d.200724.001How to use a DOI?
- Keywords
- Signal processing; Electromyogram; Discrete wavelet transform; Feature extraction; Pattern recognition; Support vector machine; Deep Learning Neural Network
- Abstract
This paper presents a novel framework that automatically classifies hand grasps using Electromyogram (EMG) signals based on advanced Wavelet Transform (WT). This method is motivated by the observation that there lies a unique correlation between different samples of the signal at various frequency levels obtained by Discrete WT. In the proposed approach, EMG signals captured from the subjects are subjected to denoising using symlet wavelets, followed by Principal Component Analysis (PCA) for dimensionality reduction. Further, the important attributes of the signal are extracted using Lifting Wavelet Transform (LWT) and Dual Tree Complex WT (DTCWT). Multiple classifiers such as Feed Forward Neural Networks (FFNN), Cascaded Feed Forward Neural Networks (CFNN), Support Vector Machine (SVM) and Deep Learning Neural Network (DLNN) are used for classification. The simulation results are compared with various training algorithms and it is observed that DTCWT features combined with CFNN and trained with Gradient Descent with Adaptive Back Propagation (GDABP) algorithm achieved the best performance. The advantages of the proposed method were proved by comparing with the earlier conventional methods, in terms of recognition performance. These experimental results prove that the proposed method gives a potential performance in the recognition of hand grasps using EMG signals. In addition, the proposed method supports clinicians to improve the performance of myoelectric pattern recognition.
- Copyright
- © 2020 The Authors. Published by Atlantis Press B.V.
- Open Access
- This is an open access article distributed under the CC BY-NC 4.0 license (http://creativecommons.org/licenses/by-nc/4.0/).
Download article (PDF)
View full text (HTML)
Cite this article
TY - JOUR AU - A. Haiter Lenin AU - S. Mary Vasanthi AU - T. Jayasree PY - 2020 DA - 2020/07/31 TI - Automated Recognition of Hand Grasps Using Electromyography Signal Based on LWT and DTCWT of Wavelet Energy JO - International Journal of Computational Intelligence Systems SP - 1027 EP - 1035 VL - 13 IS - 1 SN - 1875-6883 UR - https://doi.org/10.2991/ijcis.d.200724.001 DO - 10.2991/ijcis.d.200724.001 ID - Lenin2020 ER -