Whale Optimization for Wavelet-Based Unsupervised Medical Image Segmentation: Application to CT and MR Images
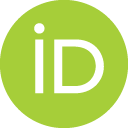
- DOI
- 10.2991/ijcis.d.200625.001How to use a DOI?
- Keywords
- Medical segmentation; Unsupervised machine learning; Wavelet transform; Texture features; Clustering; K-means; Fuzzy K-means; Particle swarm optimization; Genetic algorithm; Whale optimization; CT and MRI
- Abstract
Image segmentation plays crucial role in medical image analysis and forms the basis for clinical diagnosis and patient's treatment planning. But the large variation in organ shapes, inhomogeneous intensities, poor contrast, organic nature of textures and complex boundaries in medical images makes segmentation process adverse and challenging. Further, the absence of annotated ground-truth dataset in medical field limits the advantages of the trending deep learning techniques causing several setbacks. Though numerous unsupervised methods are reported in literature to combat the challenges in medical domain, achieving better segmentation quality still remains as an open issue. This work aim to address this issue integrating the strength of multiresolution analysis and the meta-heuristic optimization techniques for unsupervised medical image segmentation. The proposed approach employs undecimated wavelet frames to extract translation invariant texture and gray information at different orientations to effectively characterize the textures in medical images. Next, the approach introduces the latest meta-heuristic whale optimization algorithm (WOA), the global optimizer to enhance the performance of unsupervised clustering algorithm with optimized cluster centers to cluster the extracted wavelet texture features. Moreover, the study contributes to fill the gap in literature investigating for the first time different intelligence algorithms such as fuzzy, genetic algorithm (GA) and particle swarm optimization (PSO) for unsupervised medical image segmentation to demonstrate the efficacy of the proposed approach. Evaluation was performed on medical CT and MR images based on feature similarity (FSIM), dice (DC) and feature of merits (FOMs). Experimental results demonstrates the supremacy of the proposed approach over other intelligence algorithms. Finally, statistical study with ANOVA analysis was carried out to confirm the significance of the proposed approach in determining the optimal solution and displaying promising segmentation results toward diagnostic support for radiologist.
- Copyright
- © 2020 The Authors. Published by Atlantis Press SARL.
- Open Access
- This is an open access article distributed under the CC BY-NC 4.0 license (http://creativecommons.org/licenses/by-nc/4.0/).
Download article (PDF)
View full text (HTML)
Cite this article
TY - JOUR AU - Thavavel Vaiyapuri AU - Haya Alaskar PY - 2020 DA - 2020/07/01 TI - Whale Optimization for Wavelet-Based Unsupervised Medical Image Segmentation: Application to CT and MR Images JO - International Journal of Computational Intelligence Systems SP - 941 EP - 953 VL - 13 IS - 1 SN - 1875-6883 UR - https://doi.org/10.2991/ijcis.d.200625.001 DO - 10.2991/ijcis.d.200625.001 ID - Vaiyapuri2020 ER -