A Neural Network for Moore–Penrose Inverse of Time-Varying Complex-Valued Matrices
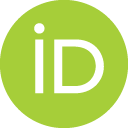
- DOI
- 10.2991/ijcis.d.200527.001How to use a DOI?
- Keywords
- Zhang neural network; Moore–Penrose inverse; Finite-time convergence; Noise suppression
- Abstract
The Moore–Penrose inverse of a matrix plays a very important role in practical applications. In general, it is not easy to immediately solve the Moore–Penrose inverse of a matrix, especially for solving the Moore–Penrose inverse of a complex-valued matrix in time-varying situations. To solve this problem conveniently, in this paper, a novel Zhang neural network (ZNN) with time-varying parameter that accelerates convergence is proposed, which can solve Moore–Penrose inverse of a matrix over complex field in real time. Analysis results show that the state solutions of the proposed model can achieve super convergence in finite time with weighted sign-bi-power activation function (WSBP) and the upper bound of the convergence time is calculated. A related noise-tolerance model which possesses finite-time convergence property is proved to be more efficient in noise suppression. At last, numerical simulation illustrates the performance of the proposed model as well.
- Copyright
- © 2020 The Authors. Published by Atlantis Press SARL.
- Open Access
- This is an open access article distributed under the CC BY-NC 4.0 license (http://creativecommons.org/licenses/by-nc/4.0/).
Download article (PDF)
View full text (HTML)
Cite this article
TY - JOUR AU - Yiyuan Chai AU - Haojin Li AU - Defeng Qiao AU - Sitian Qin AU - Jiqiang Feng PY - 2020 DA - 2020/06/17 TI - A Neural Network for Moore–Penrose Inverse of Time-Varying Complex-Valued Matrices JO - International Journal of Computational Intelligence Systems SP - 663 EP - 671 VL - 13 IS - 1 SN - 1875-6883 UR - https://doi.org/10.2991/ijcis.d.200527.001 DO - 10.2991/ijcis.d.200527.001 ID - Chai2020 ER -