Understanding the Highly Sensitive Health Communication Behavior in Social Media from the Perspective of the Risk Perception Attitude Framework and Perceived Interactivity
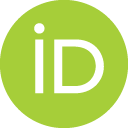
- DOI
- 10.2991/ijcis.d.210223.001How to use a DOI?
- Keywords
- Social media; Perceived interactivity; Risk perception attitude; Perceived risk; Perceived involvement; Reproductive health
- Abstract
Integrating aspects of the risk perception attitude framework and perceived interactivity, this study investigates the impact of the social media interaction environment on users' motivation and behavioral intention to reproductive health communication. A survey was conducted, and structural equation modeling was used to test the research model. The results demonstrate that human–human interaction can improve self-efficacy and reduce perceived risk and has both direct and indirect positive effects on health communication intention. However, human–information interaction has no significant impact on self-efficacy or health communication intention, and it has a positive impact on perceived risk, thus indirectly affecting health communication intention in a negative direction. The results also show that perceived involvement (PI) is a moderating variable. The findings help us to understand how perceived interactivity affects highly sensitive health communication.
- Copyright
- © 2021 The Authors. Published by Atlantis Press B.V.
- Open Access
- This is an open access article distributed under the CC BY-NC 4.0 license (http://creativecommons.org/licenses/by-nc/4.0/).
1. INTRODUCTION
Reproductive health (RH) is a state of complete physical, mental, and social well-being, not merely the absence of disease or infirmity, in all matters relating to the reproductive system and to its functions and processes [1]. Problems of RH, which include diseases of the reproductive system, early pregnancy, abortion, sexually transmitted disease, and assisted reproduction [1], are highly sensitive and even taboo topics in some Eastern countries [2]. In previous times, people could only turn to their parents and closest friends for information and advice on these problems. However, thanks to the widespread availability of social media, such as Facebook and WeChat, people have a new place they can turn when seeking health information [3]. Such sites are often the first choice for adolescents seeking RH information [2]. At the same time, social media has become an important tool for healthcare providers to implement targeted digital communication and interventions for RH [4,5].
Social media is characterized by high levels of interactivity and sociality, which greatly benefit RH communication [6–9]. The foremost topic feature of this assistance is “the widening and accessibility access of health information to a variety of individual groups” [10]. Two-way communication channels support real-time and convenient interaction and dialogue between users [11]. Patients can join online health communities (OHCs) to discuss and exchange information about their conditions, medications, and health experiences [12]. At the same time as obtaining information support, they can also receive emotional and social support, and their self-efficacy can be improved [9,13].
However, the potential for hidden problems and risks brought about by social media in health communication cannot be ignored [14,15]. User-generated content (UGC) [16] has produced extensive marketing content and incorrect information on social media [17], which may have an irreversible effect on information adopter [18]. In the case of Wei Zexi in China, for example, treatment was delayed due to the adoption of incorrect medical information, which ultimately cost lives [19]. Privacy concerns are a key factor in RH communication among social media users, especially when for highly sensitive information [20–22]. In addition, the feedback interaction mechanism of social media can raise concerns among users that their own communications will be misunderstood by others and even lead to discrimination, hostility, or aggression from others; these concerns can create anxiety and tension [4,5,22,23]. Therefore, the impacts of the social media interaction environment on users' intention to RH communication are very complex. The ways in which the interactive environment of social media influences users' intentions to RH communication is the focus of this study.
Most previous studies have explored the positive impact of social media on users' intentions to health communication. Researchers found that the technical empowerment [24,25], perceived interactivity [8,26–28], and social support [9] functions of social media have a positive impact on users' motivations (e.g., self-efficacy, outcome expectation of health self-management, and outcome expectations of social relationships) and health communication intention. Recently, Li et al. [15] and Deng and Li [9] indicated that risk factors should not be ignored. Users are willing to be involved in health communication only when perceived benefits exceed perceived risk [15]. Perceived risk may be an especially key factor for highly sensitive RH topics, influencing people's participation in RH communication [4,5,29]. However, few studies have focused on the influential factors of RH communication, especially on the impact of the social media interaction environment on users' perceived risk and RH communication intentions.
To fill this gap, this study investigates the antecedent factors of the intention to highly sensitive health communication by integrating the perspectives of the risk perception attitude (RPA) framework and perceived interactivity. Social cognition theory (SCT) [30] argues that individual behaviors are jointly influenced by environmental factors and individual factors. Individuals participate in health communication for the purpose of protecting their individual health, as part of self-protection behavior [9]. The RPA framework is a well-established theory that assesses the motivation of self-protection behavior, which holds that perceived risk and self-efficacy are two dimensions that affect individual protection behavior [9,31]. In this study, the terms perceived risk and self-efficacy were taken from the RPA framework as variables of individual motivation.
Among environmental factors, media synchronization theory (MST) [32] holds that media tools can affect the quality and performance of communication. Interactivity is the most prominent feature of social media. Environmental psychology holds that an individual's perceived interactivity in a social media environment is an important variable for his or her intention to perform health communication [11,33]. Therefore, perceived interactivity is adopted as an environmental variable in this study. Hsu et al. argued that perceived interactivity can be divided into two dimensions: human–information interaction and human–human interaction [34].
In addition, illness and individual involvement in illness can also affect health communication behavior. Communication on RH topics has always been a sensitive privacy topic [2,29], especially in Eastern countries. The participants in communication topics regarding RH may be more sensitive to perceived risk than other illness [29]. This paper takes RH as a situational factor to better investigate the antecedents of users' intentions for health communication in this context. At the same time, we used perceived involvement (PI) as a moderating variable to examine the role of perceived interactivity at different levels of involvement in RH issues
In summary, the purpose of this study is to identify the antecedents of RH communication by integrating the perspectives of the RPA framework and perceived interactivity. Accordingly, this study addresses the following four questions:
Do user's self-efficacy and perceived risk affect RH communication?
Does perceived interactivity affect RH communication? Do the two dimensions of perceived interactivity affect RH communication differently?
Does perceived interactivity affect RH communication through the mediation of self-efficacy and perceived risk?
Does PI moderate the relationship between perceived interactivity and user's perceived risk and self-efficacy?
2. LITERATURE REVIEW AND HYPOTHESIS DEVELOPMENT
2.1. RPA Framework
Health communication in social media describes the events of “the general public, patients, and health professionals communicating about health issues using social media platforms” [35], including health information sharing and seeking, which is a health protection behavior [9]. The RPA framework was proposed by Rimal [36], which indicated that individual protection behavior could be explained in two dimensions, namely, perceived risk and self-efficacy [31,37,38]. Perceived risk refers to users' subjective expectation of potential uncertainties or possible losses in health communication with social media [39], which can be divided into perceived health risk and perceived information risk. The former promotes health communication behaviors [31,37,38], while the latter negatively affects health communication behaviors [9]. This paper explores whether the interactive environment of social media brings risks to RH communication, so that perceived risk refers to the latter, including information uncertainty risk, privacy risk, and psychological risk. Users may experience concerns about the irreversible physical effects of adopting incorrect health information [9], hesitation about the disclosure of their private information [21,40,41], and psychological pressure, such as nervousness and anxiety, due to the highly sensitive nature of RH communications [40], all of which negatively affect their intentions to RH communication. Therefore, the following hypothesis is proposed:
H1: Perceived information risk has a negative association with health communication intention.
Self-efficacy is defined as individuals' expectation of their ability to achieve a certain behavior and the expectation of positive results from participating in a particular behavior [31,42]. Many studies have shown that self-efficacy significantly affects users' information behaviors [43–45], including health communication behaviors [9,46]. Therefore, the following hypothesis is proposed:
H2: Information self-efficacy has a positive association with health communication intentions.
2.2. Perceived Interactivity
Perceived interactivity refers to the user's experience and self-reporting of the interactive environment, and it is a psychological perception variable [47], which can be defined as the extent to which users perceive their experiences of interpersonal interactions and the sense that they are in the presence of a social other [8,48].
The perceived interactivity can be divided into two dimensions: human–information interaction and human–human interaction [8,28]. Human–information interaction refers to interactions between people and content, as well as to the degree of control over content people have through social media's functions of inquiry, selection, replying, forwarding, and commenting [28]. It reflects users' perception of the interaction convenience of the social media technology environment and the satisfaction of users' information needs.
By contrast, human–human interaction refers to the interpersonal interaction carried out through the function of information sending and receiving and real-time communication in social media [28], a kind of parasocial interaction in the form of a network [48]. Social media is the tool and locus of interaction, and the subject of interaction is the individual who is understood to be behind the interaction on the other side of social media. In human–human interaction, users perceive the convenience of the interaction environment of social media as well as the response speed, attitude, and emotional and social support of the interaction objects [9], which satisfies users' informational, emotional, and social needs [49].
Perceived interactivity is widely recognized for its influence on user acceptance and continuance intention [11,26,49]. Lin and Chang found that perceived interactivity affects health management efficiency, social relationship efficiency, and health information exchange in social media users [8]. That is, they demonstrated the impact of perceived interactivity on the self-efficacy dimension of the RPA framework [8], although the impact on the perceived risk dimension has not been clearly investigated. However, for highly sensitive RH communications, users are concerned about the content professionalism, privacy leakage, and psychological risk in interactions on social media. Therefore, the perceived risk entailed by the interaction environment of social media cannot be ignored. To fill this knowledge gap, this paper investigates whether perceived interactivity affects health communications through both dimensions of the RPA framework.
2.2.1. Perceived interactivity and perceived information risk
Human–information interaction describes the convenience of processing information content through social media [28]. The impact of human–information interaction on perceived risk may be complex. On the one hand, an efficient human–machine interactive system can allow users to obtain additional information and reduce the risk of information uncertainty [50]. On the other hand, using technology can have various risks [15]. Efficient human–information interaction often comes at the expense of security. For example, relative to the professional-generated health information disseminated by traditional media, the UGC of social media greatly improves the human–information interaction, but it also increases the health information risk of patient adopters because its professionalism and value cannot be guaranteed [51]. Some social media platforms allow the release of medical records, pictures, and videos instead of merely text to improve human–information interaction, but this also increases privacy risks, such as the disclosure of personal biometric information [21,52], especially in the highly sensitive area of RH. Considering the current state of affairs, where users are very dissatisfied with the quality of health information on social media [53], and even up to 50% of users believe that it cannot be trusted [54], this study suggests that high human–information interaction leads to high perceived risk.
H3: human–information interaction is positively correlated with perceived risk.
By contrast, human–human interaction reflects the convenience of interpersonal interactions between social media users [8,28], which can be evaluated along the dimensions of connectivity, responsiveness, and relationship [11]. Panteli and Sockalingam [55] and Chang [49] found that sustained and lasting online interactions with others enable users to build mutual trust, which, in turn, facilitates trusting behaviors, such as the sharing and adoption of health information. Lee et al. [50] considered that online interactivity can reduce the perceived risk of customers. Real-time human–human interaction with doctors, friends, and other patients through social media can help users calm their doubts, identify the authenticity of health information, and receive encouragement and support from others, thus reducing perceived risk [56]. Zhang et al. [53] found that the public is most interested in personalized and interactive communication with clinicians through social media. Therefore, we believe that human–human interaction can reduce perceived risk. Accordingly, we propose the following hypothesis:
H4: human–human interaction has a negative association with perceived information risk.
2.2.2. Perceived interactivity and information self-efficacy
The main purpose of social media users' participation in health communication is to obtain health knowledge or information to improve their competence in dealing with disease and self-efficacy [25,35]. MST [32] holds that the information transmission and processing capabilities of a type of media affect the quality and performance of communications [8,57]. Therefore, human–information interaction, which reflects the ability of social media to spread and process information, will affect people's performance in acquiring health information and knowledge and health self-efficacy [11,49]. Accordingly, we propose the following hypothesis:
H5: human–information interaction has a positive association with information self-efficacy.
When people encounter health problems, they seek relevant health information as well as communicating face-to-face with their relatives, friends, and peers through social media to obtain information and emotional support [13]. They also participate in OHCs through social media, seek out people with similar health problems, and gain social support by exchanging health information, recommending doctors through word-of-mouth, sharing treatment advice, and encouraging and supporting each other [12,58]. Research has shown that social support in relation to health has a positive effect on users' self-efficacy [9,13,57], especially emotional support [9,13]. Many studies have shown that human–human interaction in social media can affect the communication quality and performance, as well as the degree of information support and social support obtained, thus affecting self-efficacy [8,28,49]. The following hypothesis is therefore proposed:
H6: human–human interaction has a positive association with information self-efficacy.
2.2.3. Perceived interactivity and health communication intention
Social media is a social computing tool [59], and its human–information interaction features determine the efficiency and convenience of its information content processing [28]. Chang et al.'s study revealed that human–information interaction affects information communication, emotion and social gratification, and continuance intention [49]. Wei et al. [28] found that human–information interaction can affect people's attitudes toward and perceived stickiness of social media. People tend to use social media for information exchange only when they believe that it can provide useful interaction functions [59]. Lin and Chang [8] revealed that human–information interaction can affect the outcome expectations of health management, thus affecting the intentions to health communication. Therefore, the following hypotheses are proposed:
H7: human–information interaction has a positive association with health communication intentions.
Human–human interaction is a computer-mediated interpersonal interaction [59,60], which emphasizes the bidirectional, responsive, and real-time nature of communication [8,61]. Health communication between doctors and patients or between patients can be conducted through social media for the passing of relevant health knowledge or information, as well as for social and emotional support [49]. Many studies have shown that human–human interaction affects the quality of communication [60], which in turn affects people's communication satisfaction and the intention of the next health communication [8,26,49]. Therefore, we propose the following hypothesis:
H8: human–human interaction has a positive association with health communication intentions.
2.3. Role of PI
PI refers to the degree to which an individual feels any stimulus or situation to be relevant [62,63]. Laurent argued that perceived importance, perceived risk, and perceived value are important dimensions of PI [64]. When people realize that their situation has importance, value, or risk for them, they tend to enter into a state of high involvement.
The degree of users' PI in something is considered to be an important variable influencing attitudes to that thing and information behavior [65]. Depending on the level of involvement, users may be passive or active when they receive RH communication through social media, and they may limit or extend their processing of this communication [64]. Low-involvement users without RH problems may passively receive RH information through social media and consider it useless or even difficult or risky, while high-involvement users may hold the opposite view. In other words, PI may moderate the relationship between social media interactivity and user perception (self-efficacy and perceived information risk). The higher the user's PI in RH, the more likely they are to perceive the benefits of social media interaction and ignore its disadvantages. That is, perceived involvement can enhance the positive impact of the perceived interactivity of social media on self-efficacy, while reducing the negative impact on perceived information risk. Therefore, the following hypotheses are proposed:
H9a: Involvement reduces the positive impact of human–information interaction on information perceived risk.
H9b: Involvement enhances the positive influence of human–information interaction on information self-efficacy.
H9c: Involvement enhances the negative influence of human–human interaction on information perceived risk.
H9d: Involvement enhances the positive influence of human–human interaction on information self-efficacy.
Taking PI as an individual health context variable, this paper discusses how perceived interactivity as a technical environment variable of social media in different health contexts affects perceived information risk and self-efficacy at the level of individual perception, as well as health communication intention at the level of behavior. The research model is given in Figure 1.
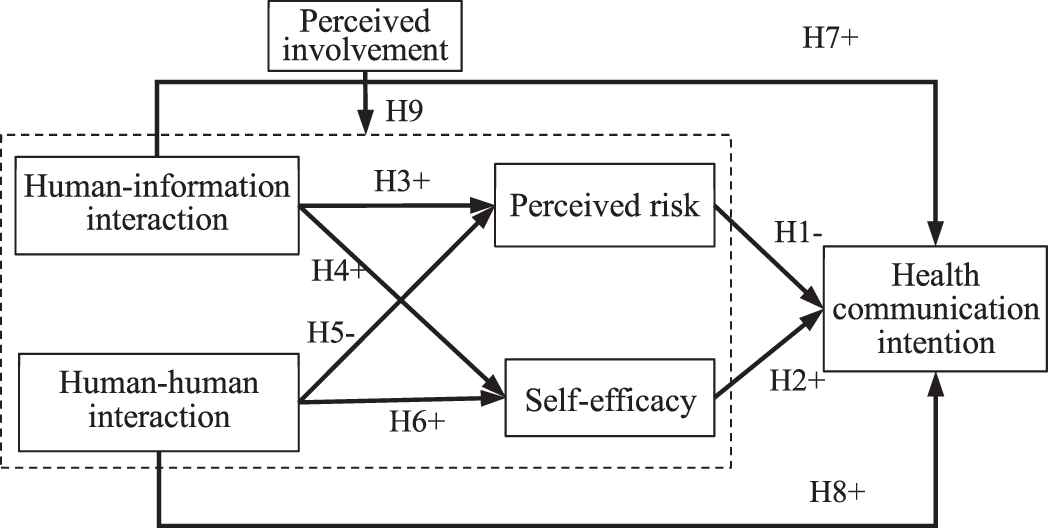
Research model.
3. METHODOLOGIES
3.1. Measurements
A questionnaire survey was conducted to collect individual perceptions of the RH behavior of social media users. To ensure the validity of the results, all of the instruments used to measure the constructs in this study were derived from previous studies and modified according to the actual survey requirements. Moreover, before any formal survey, two small-scale preliminary surveys were conducted, and modifications were made to the measurement items based on the feedback data.
The questionnaire is divided into two parts. The first part collects the basic information on the participants, and the second measures the constructs through the measurement items. A 5-point Likert scale was used to collect quantitative data, in degrees from less to more and from negative to positive, rated from 1 to 5.
Variable | N | Percentage | Variable | N | Percentage |
---|---|---|---|---|---|
Gender | Age | ||||
Male | 88 | 32.5 | <20 | 3 | 1.1 |
Female | 183 | 67.5 | 20–30 | 121 | 44.6 |
Education level | 30–40 | 92 | 33.9 | ||
Middle school or less | 99 | 36.5 | 40–50 | 48 | 17.7 |
Junior college | 40 | 14.8 | >50 | 7 | 2.6 |
University | 114 | 42.1 | Reproductive problems | ||
Postgraduate | 16 | 5.9 | Never | 89 | 32.8 |
Doctorate | 2 | 0.7 | Many years ago | 54 | 19.9 |
Profession | Nearly 3 years | 54 | 19.9 | ||
Doctors and nurses | 54 | 19.9 | Almost 1 year | 44 | 16.2 |
Enterprises, institutions | 49 | 18.1 | Past 6 months | 30 | 11.1 |
Services, business | 33 | 12.2 | Problem severity | ||
Student | 34 | 12.5 | No problem | 87 | 32.1 |
Others | 101 | 37.3 | Slight problem | 61 | 22.5 |
Some trouble | 61 | 22.5 | |||
More trouble | 38 | 14.0 | |||
Serious trouble | 24 | 8.9 |
Sample characteristics (N = 271).
The measurement items for measuring human–information interaction derive from Lin and Chang [8]. The item measuring human–human interaction is adapted from Rimal and Juon [31]. The measurement items for perceived risk were drawn from Liang et al. [66]. Some of the measurement items for information self-efficacy were adopted from Varshney [67], Chen and Hung [68], and others, and some were constructed for this research. Health communication intention uses Lin's measurements [8]. See Appendix for specific construct measurements.
3.2. Data Collecting Process
The data were taken from two sources. First, friends of the research team, including some former patients who have become friends through WeChat, were invited to participate in an online questionnaire through WeChat moments or a WeChat group (138 sources of valid data). Most of these participants had no or minor RH problems at present. Second, the patients of the Nanchang Reproductive Hospital in China, who all had conditions or concerns closely related to RH, were invited to participate in the paper questionnaire survey (133 sources of valid data), distributed to patients with the assistance of doctors.
All surveys were conducted anonymously, and a total of 279 questionnaires were collected. After removing invalid questionnaires with the same exclusion options and missing responses, 271 valid questionnaires were obtained, with an effective rate of 97.1%, which exceeded the minimum sample size of 100–150 required by the structural equation modeling (SEM) technique recommended by Gefen et al. [69].
The online and offline questionnaire items were identical, and the data were homogeneous, so we combined them for analysis. The demographic characteristics of the sample are shown in Table 1. Two-thirds of the participants in the questionnaire were women, twice as many as men, which is in line with the male-to-female ratio of the patients at the Nanchang Reproductive Hospital. According to data on RH experiences and severity, about two-thirds of the samples were from those who had experienced RH problems, so the samples reflected the realities of RH.
4. RESULTS
IBM AMOS 24 and the IBM SPSS Statistics 24 were used for the statistical analyses. The two-step approach suggested by Anderson and Gerbing [70] was used for the data analysis: the measurement model was estimated, and the structural relationships among latent constructs were examined.
4.1. Evaluation of Measurement Model
4.1.1. Reliability and validity analysis
The measurement model was evaluated with reliability and validity analysis. Standardized loading, Cronbach's alpha, combined reliability (CR), and average variance extracted (AVE) of the measurement model are shown in Table 2. Cronbach's alpha was used to verify the reliability, and the value of all indicators for it exceeded 0.7. The convergence validity was tested according to the loadings, AVE and CR. All load factors exceeded 0.5, and only two loadings (0.687, 0.659) did not exceed 0.7. All AVE values exceeded 0.5, and all CR values exceeded 0.7. Therefore, this scale had high convergent validity and combination validity.
Construct | Item | Standard Loadings | Cronbach's Alpha | CR | AVE |
---|---|---|---|---|---|
Human–information interaction (HII) | HII1 | 0.761 | 0.885 | 0.890 | 0.730 |
HII2 | 0.910 | ||||
HII3 | 0.885 | ||||
Human–human interaction (HHI) | HHI1 | 0.777 | 0.879 | 0.881 | 0.650 |
HHI2 | 0.854 | ||||
HHI3 | 0.827 | ||||
HHI4 | 0.764 | ||||
Perceived risk (PR) | PR1 | 0.700 | 0.827 | 0.830 | 0.553 |
PR2 | 0.863 | ||||
PR3 | 0.687 | ||||
PR4 | 0.710 | ||||
Self-efficacy (SE) | SE1 | 0.816 | 0.897 | 0.898 | 0.687 |
SE2 | 0.838 | ||||
SE3 | 0.842 | ||||
SE4 | 0.819 | ||||
Health communication intention (HCI) | HCI1 | 0.771 | 0.835 | 0.839 | 0.567 |
HCI2 | 0.659 | ||||
HCI3 | 0.764 | ||||
HCI4 | 0.809 |
Measurement model statistics.
The discriminant validity was tested by comparing the square root value of AVE with the correlation coefficient between the indicators, as shown in Table 3. It can be seen that all indicators' correlation coefficients were less than the square root of AVE, which indicates that the questionnaire had good discriminant validity. In general, the questionnaire in this paper had high reliability and validity.
Construct | HII | HHI | PR | SE | HCI |
---|---|---|---|---|---|
HII | 0.854 | ||||
HHI | 0.615 | 0.806 | |||
PR | −0.082 | −0.325 | 0.744 | ||
SE | 0.479 | 0.674 | −0.206 | 0.829 | |
HCI | 0.370 | 0.634 | −0.475 | 0.628 | 0.753 |
Construct correlations and square roots of AVE.
4.1.2. Testing the model's goodness of fit
In this study, AMOS 24.0 software was used to test the model's goodness of fit. The indices of the model's goodness of fit are shown in Table 4. Χ2/df is 1.93 and lower than 3. RMSEA is 0.059 lower than 0.08. AGFI is 0.863 and higher than 0.8. CFI, NFI, IFI, and TLI are all greater than 0.9. Thus, all of the fit indices are acceptable based on the suggestions of Hair et al. [71], which indicate that the model fits the data well.
Index | χ2/df | RMSEA | AGFI | CFI | NFI | IFI | TLI |
---|---|---|---|---|---|---|---|
Reference | <3 | <0.08 | >0.08 | >0.9 | >0.9 | >0.9 | >0.9 |
Result | 1.930 | 0.059 | 0.863 | 0.956 | 0.913 | 0.956 | 0.945 |
Test results for model's goodness of fit.
4.2. Structural Model Testing Results
The structural model was evaluated with AMOS 24 to test the hypothetical relationships among constructs. Figure 2 shows the normalized path coefficient and path significance. All hypothesis relationships were significant, with p-values of less than 0.05, except for the correlations between human–information interaction and self-efficacy (H4), and between human–information interaction and health communication intention (H7). That is, H1, H2, H3, H5, H6, and H8 were supported, while H4 and H7 were not.
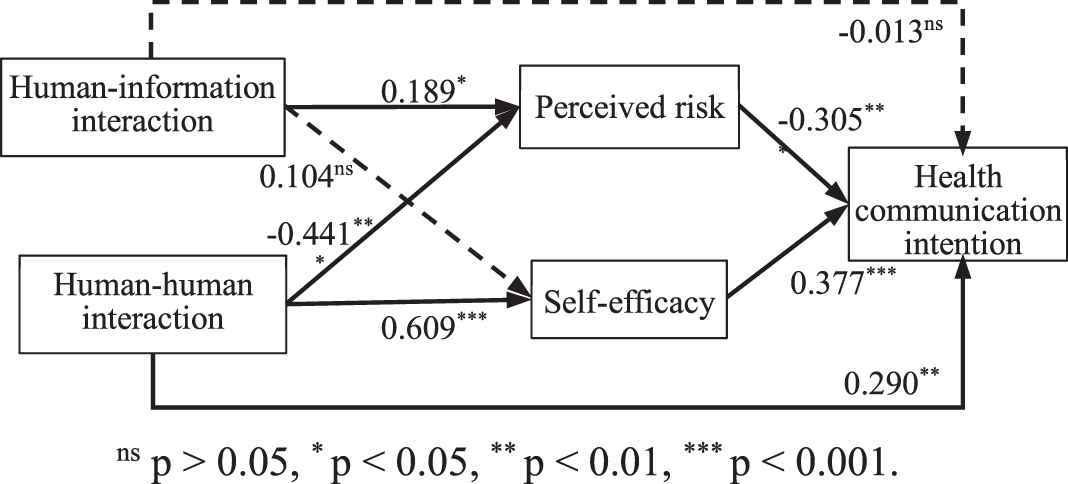
SEM analysis of research model.
Effects | Β | Lower | Upper | p |
---|---|---|---|---|
Direct Effect: HII->HCI | −0.010 | −0.139 | 0.128 | 0.911 |
Indirect Effect: HII->HCI | −0.014 | −0.115 | 0.072 | 0.662 |
HII->PR->HCI | −0.044 | −0.104 | −0.005 | 0.027* |
HII->SE->HCI | 0.030 | −0.022 | 0.104 | 0.212 |
Direct Effect: HI->HCI | 0.251 | 0.053 | 0.451 | 0.011* |
Indirect Effect: HHI->HCI | 0.315 | 0.1877 | 0.491 | 0.001** |
HHI->PR->HCI | 0.116 | 0.057 | 0.210 | 0.000*** |
HHI->SE-HCI | 0.199 | 0.088 | 0.361 | 0.001** |
p < 0.05
p < 0.01
p < 0.001.
Results of the mediating effects.
Independent Variable | PR |
SE |
||
---|---|---|---|---|
Model 1 | Model 2 | Model 1 | Model 2 | |
HII | −0.024 | −0.034 | 0.411*** | 0.418*** |
PI | −0.278*** | −2.267*** | 0.167** | 0.159** |
HII×PI | −0.135* | 0.096 | ||
R2 | 0.080 | 0.098 | 0.221 | 0.230 |
∆R2 | – | 0.018 | – | 0.009 |
∆F | – | 5.355* | – | 3.178 |
HHI | −0.201** | −0.212*** | 0.583*** | 0.582*** |
PI | −0.227*** | −0.222*** | 0.078 | 0.075 |
HHI×PI | −0.116* | 0.049* | ||
R2 | 0.117 | 0.130 | 0.371 | 0.382 |
∆R2 | – | 0.013 | – | 0.010 |
∆F | – | 4.071* | – | 4.411* |
p < 0.05
p < 0.01
p < 0.001.
Moderating effects test results.
4.3. Mediating Effect Testing Results
We further tested the mediating effects of self-efficacy and perceived risk on human–information interaction, human–human interaction and HCI using the bootstrap method in AMOS. The results are demonstrated in Table 5. The results show that human–information interaction affects health communication intentions through the complete mediating of perceived risk
4.4. Moderating Effect Testing Results
In this paper, SPSS 24.0 was used for hierarchical regression analysis to verify the moderating effect of PI on the relationship between perceived interaction and users' psychological perception layer (perceived risk, self-efficacy). The test results are shown in Table 6. They indicate that involvement has a significant negative moderating effect on the relationship between human–information interaction and perceived risk
According to the results in Table 5, involvement has an enhanced moderating effect on the relationship between human–human interaction and perceived information risk
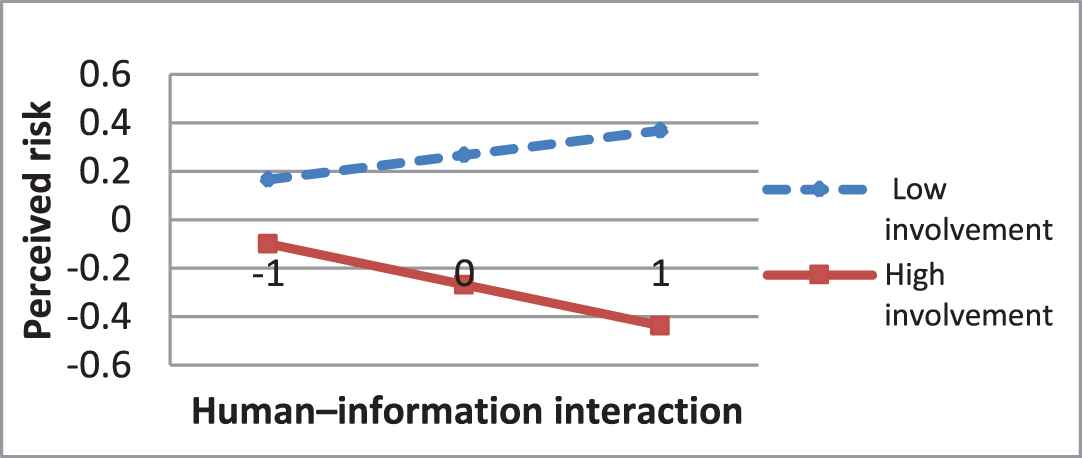
Moderation of involvement on the relationship between HII and PR.
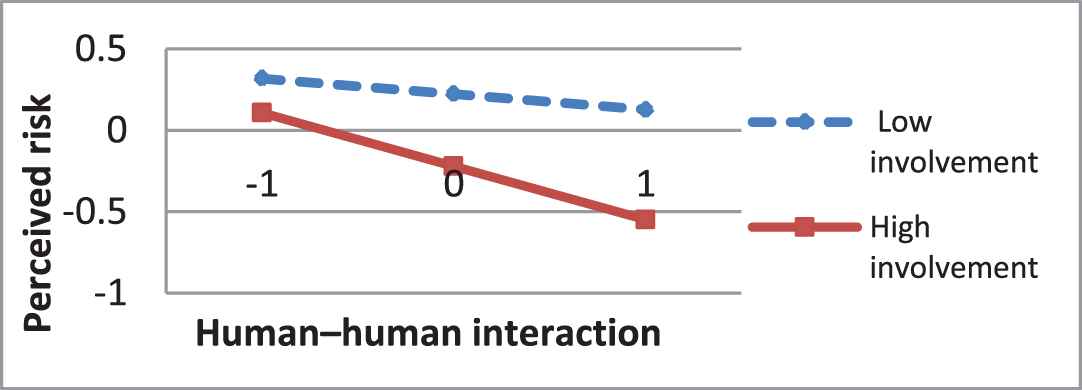
Moderation of involvement on the relationship between HHI and PR.
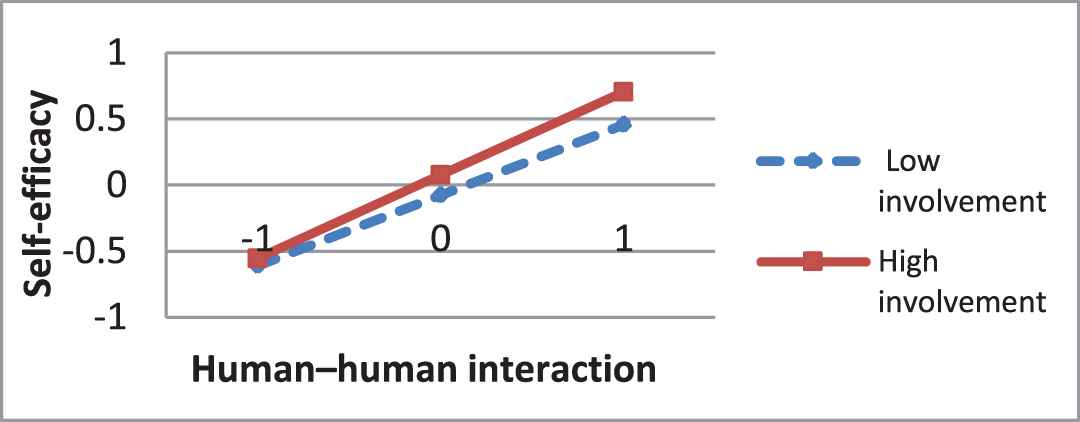
Moderation of involvement on the relationship between HHI and SE.
5. DISCUSSION
5.1. Major Findings
This study promotes the understanding of processes whereby interactive features and RPA frameworks influence communication intentions about highly sensitive RH in social media. Several findings are reported as follows.
First, the two dimensions of the RPA framework, self-efficacy and perceived information risk, both have a significant influence on RH communication, and the effect of self-efficacy is positive while the perceived information risk is negative. These findings are in line with Deng's findings that self-efficacy and perceived risk are predictors for health communication behavior [9], and are similar to Li's view that Chinese social media users engage in health communication only when perceived benefits outweigh perceived risk [8].
Second, human–information interaction has no significant direct effect on intention to RH communication, but it has significant negative mediating effect through perceived risk. These findings are contrary to our expectations and to the results produced by Lin and Chang [8] that more human–information interaction will enhance the outcome expectations of health self-management and promote health communication. The explanation for this may be that in the highly sensitive context of RH, users are very concerned about privacy leakage during human–information interaction through social media, thus weakening the benefits of human–information interaction. However, Lin's research [8] fails to consider the effect of human–information interaction on perceived risk. The results also reveal that more human–information interaction will increase perceived risk and thus weakens RH communication intentions. This finding provides an explanation for the fact that 50% of users in Beijing believed that health information generated by social media users was not reliable [54], as well as the fact that US adults' use of social media for sharing health information declined between 2013 and 2017 [72].
Third, more human–human interaction of social media can significantly reduce users' perceived risk, improve self-efficacy, and directly and indirectly promote communication intentions in RH, which is different from human–information interaction. These findings support Lin's finding that more human–human interaction can improve outcome expectations of health self-management and social relationships, thus promoting health communication [8], but it also revealed that it could also reduce people's perceived risk. Human–human interaction can enhance the relationship between interacting objects to obtain social support. Deng and Liu's [9] study reveals that social support can reduce people's perceived risk and enhance self-efficacy, thus promoting health communication, which provides an explanation for our findings.
Fourth, our mediating testing results indicate that perceived interactivity affects health communication through RPA framework mediation. Specifically, perceived risk completely mediates the impact of human–information interaction on health communication intentions. Perceived risk and self-efficacy jointly partly mediate the influence of human–human interaction on health communication intention, and the indirect effect size is larger than the direct effect. These findings extend Lin's research that perceived interactivity affects health communication only through outcome expectations.
Finally, it is found that the user situational factor (PI) is a moderating variable for the relationship between perceived interactivity and user's psychological perception (perceived risk, self-efficacy). High-involvement users are more likely to perceive the benefits of human–human interaction, including the improvement of self-efficacy and the reduction of perceived risk. Additionally, the influence of human–information interaction on perceived risk also changes from increasing to decreasing. This finding reveals the boundaries of perceived interactivity on health communication.
5.2. Theoretical Implications
The results of this study have implications for theory. First, although past studies have revealed the antecedents for health communication in social media [8,9], few have investigated the antecedents of health communication in social media on highly sensitive topics like RH, which is different from general topics. Therefore, understanding the antecedents of RH communication in social media is important because it can extend our knowledge about how to facilitate digital communication and intervention for HR [4,5] via social media.
Second, although previous studies have found that the two dimensions of RPA (perceived risk and self-efficacy) are important internal motivation factors that affect health communication in social media [9,15], and perceived interactivity is an important external environmental factor [8]. The impact between the two and their combined impact on health communication has not been closely investigated. By integrating perceived interactivity and RPA into the SCT, this study contributes to the current SCT and RPA framework literature by revealing the association between the interactive features of social media, RPA framework, and individual's behavior.
Third, this study addresses the direct, indirect, and boundary effects of different dimensions of perceived interactivity on RH communication in social media, which contributes to a more comprehensive understanding of the impact mechanisms of the social media interaction environment on health communication. The difference of the effects of different dimensions of perceived interactivity on health communication, the mediating effect of perceived risk, and the moderating effect of PI have been found. Those findings, to the best of our knowledge, have not been reported in previous studies. Our study provides a new insight into the influence mechanism of social media interactive environment on individual behavior.
5.3. Practical Implications
From a practical perspective, this study has several implications for reproductive healthcare providers (healthcare personnel and health information service personnel), health APP developers, and administrators of medical institutions and governments. First, the results show that self-efficacy is a key factor for health communication. Therefore, healthcare providers should provide communication services to meet users' information needs and emotional and to improve their outcome expectations. At the same time, they should also communicate in easy-to-understand language to enhance users' self-efficacy.
Second, the results showed that perceived risk is another key factor in health communication. Therefore, reproductive healthcare institutions and government administrators should formulate relevant rules and regulations to restrict reproductive healthcare providers to protect users' privacy and ensure information professionalism, so as to reduce users' perceived information risks. Moreover, the communication tone should be motivational, friendly, encouraging, polite, and respectful, rather than didactic, shameful [4], so as to reduce users' perceived psychological risk.
Third, the results also show that better human–human interaction characteristics of social media may improve users' self-efficacy, reduce perceived risk, and promote RH communication, while human–information interaction has different effects on perceived risk at different levels of involvement. Therefore, reproductive healthcare providers should choose social media platforms with high human–human interaction potential for communication, and health APP designers should improve the human–human interaction of apps to promote RH communication. At the same time, healthcare providers should adopt novel information service strategies to respond to different levels of user involvement. For low-involvement users who are particularly sensitive to risks, a low-interaction approach should be adopted, while for high-involvement users, a high-interaction approach should be adopted, so as to better reduce users' perceived risks and thus promote health communication.
5.4. Limitations
This study had some limitations. First, high-involvement individuals come from patients and their families in only one reproductive hospital. Since there is no maternity department in this reproductive hospital, the vast majority of patients suffer from highly private and painful RH problems such as reproductive system diseases, sexually transmitted diseases or infertility, rather than low-privacy or pleasant RH problems such as pregnancy or childbirth. Second, the illness experience and the degree of health problem risk were selected as the measures for involvement factors in this paper, which did not take into account the perception of people (such as relatives and friends of patients) without relevant experience but with a high degree of concern about the health problem, which may cause the measurement of PI to be inaccurate. Therefore, future research needs to expand the scope of investigation and optimize the scale for PI.
CONFLICTS OF INTEREST
There are no known conflicts of interest.
AUTHORS' CONTRIBUTIONS
Guo constructed the research model, wrote and revised the paper. Liao analyzed the data and wrote the first draft of the paper. Li conducted surveys and collected data. Liu designed and directed the study and provided revision advice.
Funding Statement
This study was jointly funded by the National Natural Science Foundation of China and the Education Department of Jiangxi Province, but it was not involved in this study except for financial support.
ACKNOWLEDGMENTS
This work was supported by the national natural science foundation of China [grant number: 72064029 and 72064027]; and the university humanities and social sciences project of Jiangxi province, China [grant number: JC18116]. This study has been approved by the medical ethics review committee of Nanchang reproductive hospital in China. We would also like to thank those who participated in the survey for their generous time and thoughtful advice.
APPENDIX: MEASUREMENT SCALES
Human–Information Interaction (HII)
HII1: I can easily get health information or other people's experience by using social media
HII2: I can easily share or forward health information by using social media
HII3: I can easily like or comment on health information by using social media
Human–Human Interaction (HHI)
HH1: I can easily exchange ideas with others (friends, doctors, patients) by using social media
HH2: I can easily establish a good relationship with others (strangers, doctors, patients) and become friends by using social media
HH3: I can easily get encouragement, comfort, and support from other people (friends, doctors, patients) by using social media
HH4: I can easily verify the authenticity and usefulness of information from others (relatives, friends, doctors) by using social media
Perceived Risk (PR)
PR1a: Are you worried about the professionalism and authenticity of social media information?
PR1b: Are you worried about the health consequences of adopting social media messages?
PR2: Do you worry about privacy when communicating with friends, family, or doctors on WeChat?
PR3: Do you worry about privacy when you communicate anonymously in virtual communities (e.g., haodoc.com, online patients' groups)?
PR4: Do you feel psychological pressure when you communicate with friends or doctors on WeChat?
Information Self-Efficacy (SE)
SE1: I think social media health information can increase my knowledge and ability of reproductive health
SE2: I believe that communication through social media can solve some of my reproductive health problems or doubts
SE3: I believe that communication through social media can build or maintain good relationships with others (doctors, friends, and patients)
SE4a: I think I can tell the truth and validity of most information by analyzing it or communicating with others
SE4b: I think I have high information literacy and can protect my information security and privacy
Health Communication Intention (HCI)
HCI1: Are you willing to use social media for reproductive health information?
HCI2: Are you willing to share your reproductive health information or knowledge on social media?
HCI3: If you have a reproductive health problem, are you willing to seek out people with the same problem on social media for consultation and communication?
HCI4: If you have a reproductive health problem, are you willing to seek help from friends or doctors on social media?
Perceived Involvement (PI)
PI1: How long ago did you or your family have a reproductive health problem?
PI2: If you or a family member has had a reproductive health problem, how serious is it?
REFERENCES
Cite this article
TY - JOUR AU - Lusheng Guo AU - Lifang Liao AU - Donglan Li AU - Chunnian Liu PY - 2021 DA - 2021/03/01 TI - Understanding the Highly Sensitive Health Communication Behavior in Social Media from the Perspective of the Risk Perception Attitude Framework and Perceived Interactivity JO - International Journal of Computational Intelligence Systems SP - 922 EP - 934 VL - 14 IS - 1 SN - 1875-6883 UR - https://doi.org/10.2991/ijcis.d.210223.001 DO - 10.2991/ijcis.d.210223.001 ID - Guo2021 ER -