Tree-Based Contrast Subspace Mining for Categorical Data
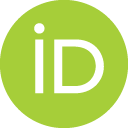
- DOI
- 10.2991/ijcis.d.201020.001How to use a DOI?
- Keywords
- Mining contrast subspace; Contrast subspace; Categorical data; Feature selection; Data mining
- Abstract
Mining contrast subspace has emerged to find subspaces where a particular queried object is most similar to the target class against the non-target class in a two-class data set. It is important to discover those subspaces, which are known as contrast subspaces, in many real-life applications. Tree-Based Contrast Subspace Miner (TB-CSMiner) method has been recently introduced to mine contrast subspaces of queried objects specifically for numerical data set. This method employs tree-based scoring function to estimate the likelihood contrast score of subspaces with respect to the given queried object. However, it limits the use of TB-CSMiner on categorical values that are frequently encountered in real-world data sets. In this paper, the TB-CSMiner method is extended by formulating the tree-based likelihood contrast scoring function for mining contrast subspace in categorical data set. The extended method uses features values of queried object to gather target samples having similar characteristics into the same group and separate non-target samples having different characteristics from this queried object in different group. Given a contrast subspace of the target samples, the queried object should fall in a group having target samples more than the non-target samples. Several experiments have been conducted on eight real world categorical data sets to evaluate the effectiveness of the proposed extended TB-CSMiner method by performing classification tasks in a two-class classification problem with categorical input variables. The obtained results demonstrated that the extended method can improve the performance accuracy of most classification tasks. Thus, the proposed extended tree-based method is also shown to have the ability to discover contrast subspaces of the given queried object in categorical data.
- Copyright
- © 2020 The Authors. Published by Atlantis Press B.V.
- Open Access
- This is an open access article distributed under the CC BY-NC 4.0 license (http://creativecommons.org/licenses/by-nc/4.0/).
Download article (PDF)
View full text (HTML)
Cite this article
TY - JOUR AU - Florence Sia AU - Rayner Alfred AU - Yuto Lim PY - 2020 DA - 2020/10/29 TI - Tree-Based Contrast Subspace Mining for Categorical Data JO - International Journal of Computational Intelligence Systems SP - 1714 EP - 1722 VL - 13 IS - 1 SN - 1875-6883 UR - https://doi.org/10.2991/ijcis.d.201020.001 DO - 10.2991/ijcis.d.201020.001 ID - Sia2020 ER -