The Evolving Landscape of Myelodysplastic Syndrome Prognostication
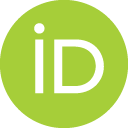
- DOI
- 10.2991/chi.d.200408.001How to use a DOI?
- Keywords
- Acute myeloid leukemia (AML); Myelodysplastic syndromes (MDS); Prognostic model; Machine learning
- Abstract
Myelodysplastic syndromes (MDSs) are potentially devastating monoclonal deviations of hematopoiesis that lead to bone marrow dysplasia and variable cytopenias. Predicting severity of disease progression and likelihood to undergo acute myeloid leukemia transformation is the basis of treatment strategy. Some patients belong to a low-risk cohort best managed with conservative supportive care, whereas others are included in a high-risk cohort that requires decisive therapy with hematopoietic cell transplantation or hypomethylating agent administration. Risk scoring systems for MDS prognostication were traditionally based on karyotype characteristics and clinical factors readily available from chart review, and validation was typically conducted on de novo MDS patients. However, retrospective analysis found a large subset of patients incorrectly risk-stratified. In this review, the most commonly used scoring systems are evaluated, and pitfalls therein are identified. Emerging technologies such as personal genomics and machine learning are then explored for efficacy in MDS risk modeling. Barriers to clinical adoption of artificial intelligence-derived models are discussed, with focus on approaches meant to increase model interpretability and clinical relevance. Finally, a guiding set of recommendations is proposed for best designing an accurate and universally applicable prognostic model for MDS, which is supported by more than 20 years of observation of traditional scoring system performance, as well as modern efforts in creating hybrid genomic-clinical scoring systems.
- Copyright
- © 2020 International Academy for Clinical Hematology. Publishing services by Atlantis Press International B.V.
- Open Access
- This is an open access article distributed under the CC BY-NC 4.0 license (http://creativecommons.org/licenses/by-nc/4.0/).
1. INTRODUCTION
Myelodysplastic syndromes (MDSs) are malignant clonal diseases of hematopoiesis which present with bone marrow (BM) dysplasia and variable cytopenias. The etiology of MDS is usually idiopathic, although environmental causes have been identified (exposure to benzene, radiation, alkylating agents), which may lead to an accumulation of oncogenic mutations in a clonal progenitor cell [1]. The progression and extent of disease is difficult to predict, with a spectrum of outcomes occurring in seemingly similar cohorts of patients, ranging from relatively benign with only occasional transfusions being needed, to rapid progression to acute myeloid leukemia (AML) and death [2–5]. The current standard of care for MDS patients depends upon early risk stratification according to predicted overall survival (OS), given that the choice of intervention is guided by prognosis [6]. In today's treatment of the MDS patient, an initial encounter with a poor prognosis warrants aggressive therapy, possibly including hematopoietic cell transplantation (HCT) or a hypomethylating agent (HMA) [5], whereas patients stratified to a low-risk cohort for disease progression have less-invasive options available, such as erythropoiesis support, serial transfusions, and adjunct therapies [6]. This dichotomy emphasizes the need for accurate prognostication and explains why so much research has historically been dedicated to building prognostic models, both for guiding therapeutic care and for establishing realistic expectations for the patient and physician.
In recent years, the most widely used risk stratification scoring systems in clinical practice and in clinical trial eligibility are the international prognostic scoring system (IPSS) and the revised IPSS (IPSS-R) [3,7–10]. These systems attempt to predict a patient's general prognosis using clinical features, such as blood count, BM blast percentage, and cytogenetic characteristics. Numerous other scoring systems have been reported and more still currently under development [11–13]. Here, we detail the landscape of MDS prognostication and offer insight into the evolution of the field.
2. CURRENT CLINICAL MDS PROGNOSTICATION
The IPSS, developed in 1997, provided clinicians with useful risk stratification by assessing BM blast percentage, karyotype categorization for deletions and abnormalities, and cytopenias defined as hemoglobin <100 g/L, absolute neutrophil count (ANC) <1,000/µL, and platelets < 100,000/mm3. It ranked patients into four cohorts of risk: low, intermediate-1, intermediate-2, and high. A revision of the IPSS was released in 2012, which improved risk prediction by redefining cutoffs for BM blast percentages and cytopenias, adding numerous additional cytogenetic features, and establishing five risk cohorts instead of four: very low, low, intermediate, high, and very high. The IPSS-R is now the most widely used prognostic mechanism for MDS, but several other scoring systems are available, the most notable being the Global MD Anderson Prognostic Scoring System (MDAPSS) and the WHO Prognostic Scoring System (WPSS). All these systems attempt to combine clinical features into a simplified model that predicts disease outcomes.
The features used in determining risk scores can be thematically divided into a patient-related group and a disease-related group. Patient-related factors are those that describe the patient's health apart from MDS-modified attributes, such as demographics, comorbidities, performance status, and other scoring system assignments. Disease-related factors encompass markers of MDS pathology, such as BM blast percentage, presence of anemia and karyotype characteristics, but also include emerging technologies, such as personal genomics. Despite the validated efficacy of IPSS-R, inherent limitations to the model's design prevent it from being universally applicable, including being built without mutational analysis apart from general cytogenetic findings. This lack of genetic insight, along with shortcomings regarding universal applicability and flexibility, argues for the creation of a new model taking into account the lessons learned from years of IPSS-R usage, such as how to accommodate treatment failure, cases of MDS due to therapy or medication, intermediate-risk group assignment, and accuracy in risk stratification.
2.1. Predicting Outcomes in Nonstandard Patients
The IPSS was developed using a cohort of 816 MDS patients seen at clinic onset and prior to treatment with HMA or HCT. This means that the initial feature selection and statistical validation were contingent upon the specific population being considered. Later research validated this rigid scoring system's ability to risk stratify patients in various disease states, but it has not been found to predict outcomes after disease modifying treatment failure. The importance of scoring system flexibility is well demonstrated by considering a patient risk stratified into the low-risk cohort based on IPSS-R, resulting in an expected survival of 5.7 years. After trialing HMA therapy and ultimately failing to respond, and assuming the pre- and post-HMA clinical presentation is generally similar, the patient would be risk stratified into the same low-risk group and have the same expected survival of 5.7 years, despite empiric evidence indicating that the average mortality is about 4–6 months from failed HMA treatment [14–16]. Similarly, attempts have been made to extrapolate the IPSS and IPSS-R algorithms to stratify patients with therapy-related or secondary MDS, but again were not found to be predictive, likely owing to the homogeneity of the population used to build the scoring system and simplicity of features utilized [17–19].
2.2. Struggling with Intermediate-Risk Groups
The original IPSS assigned many patients to the intermediate-1 and intermediate-2 groups, which were redefined and simplified to a single intermediate group with the release of IPSS-R. The intermediate group is difficult to interpret and may be inflated, due to a lack of predictive features in the scoring system, which would otherwise drive a patient's classification to low versus high risk. This is exemplified by intermediate-risk patients with unusual ferritin, LDH, EXH2, or TP53 characteristics, all of which correlate with high-risk status but are missing from IPSS-R [13]. Reducing the number of patients assigned to intermediate-risk status may improve outcomes and greatly facilitate clinical trial enrolment, which frequently seeks either high- or low-risk patients only.
2.3. Risk Stratification Accuracy
The purpose of prognostic modeling early in the clinical course of MDS is to guide management and improve OS. While any model will be imperfect, the popularity of IPSS and IPSS-R allows for real-world evaluation. The IPSS often underestimates OS, with a difference between predicted mean and observed mean OS being −23.3 months for the low-risk patient cohort, and −11.1 months for the high-risk patient cohort. Conversely, the IPSS-R frequently overestimates OS, with a mean difference of 70.6 months in the low-risk group and 6.7 months in the high-risk group.
3. RECENT ADVANCEMENTS IN MODEL CREATION
The strength of a risk stratifying model comes from the predictive features it is built upon. Although the available patient-factors today are much the same as when IPSS was first published, disease-factors have been multiplying, as a result of next generation DNA sequencing and mutation discovery, culminating in a nearly overwhelming source of patient data to interpret. These mutations require careful consideration to determine prognostic usefulness, as feature interaction can easily exaggerate or mask a mutation's ability to modify patient outcomes [20]. Now that genome mutability is readily interpretable on a research basis and, likely soon, on a clinical basis, the utility of DNA mutations in MDS prognostication needs to be interrogated.
3.1. Including Mutation Features
An abundance of mutations has been described as implicated in MDS. Many such mutations simply correlate with the presence of disease and offer only questionable prognostic value, while others significantly modulate OS and are strong candidates for future modeling, such as TP53, RUNX1, and more [11–13,20–25]. Prior to the mutational analysis advent, most models differed primarily by patient-factor cutoffs or arrangement of stepwise classification algorithms based on roughly the same available features. Leveraging this influx of new prognostic indicators has the potential to accelerate MDS modeling, but the complexity of this genomic data brings new challenges. The interdependence of features at different times during disease progression or in conjunction with certain other feature states dramatically complicates identification of truly prognostic mutations. This phenomenon has been demonstrated by numerous studies, including a survey of 104 genes among 944 MDS patients in which 26 mutations were initially found to alter OS, but after correcting for confounding features, only ASXL1, KRAS, PRPF8, RUNX1, and SF3B1 remained significant [11]. Similarly, 12 mutations were found to independently decrease OS in a study of 3,392 patients, but correction for confounding IPSS-R stratification negated the prognostic ability of all but 4 mutations [26]. Another example found CBL, NRAS, and TP53 to correlate with decreased OS, but the effect was magnified in patients categorized as having a complex karyotype (CK), with TP53-mutated individuals with normal karyotypes enjoying a 5-year post-procedure survival of 73% [27]. Genotypes can also inform therapy choices, with a study of 1,514 HCT patients demonstrating molecular subgroups that strongly correlated with outcomes [28]. Untangling these mutations is burdensome, but having new features with strong OS correlation from which predictive models can be built more than justifies the endeavor.
3.2. Assessing Mutational Heterogeneity
An additional consideration for this modern class of prognostic features is variability within a particular mutated gene. The majority of experimental models now attempting to incorporate mutational data do so in a binary present/absent manner. This allows for ease of downstream analysis and reduces the sample size needed to reach significance, but disregards a wealth of detail that may determine a mutation's actual effect on OS. The type of mutation (silent, missense, nonsense, etc.) and variant allele frequency (VAF) can be more informative than knowing if a gene contains a mutation, since the functional effect of a wildtype gene with a silent mutation is different from that of a gene with s a nonsense mutation. Cataloguing mutational attributes with this specificity, and moreover, collecting enough patients with each intra-mutational variation to allow statistical analysis pose new challenges. However, similarly to the decision to use mutational features in MDS prognostication, the promise of improved model accuracy warrants the effort. Again, consider TP53, known to be detrimental to OS. A patient with TP53 and a VAF of 50% or greater has a predicted median survival of 3.4 months but, it the VAF is of less than 25%, the predicted median survival will be 12.4 months, which is 3.6 times longer. Similarly, the VAF of mutations associated with poor prognosis in HCT patients before and after treatment correlates with risk of disease progression 30 days after transplant [29]. The breadth of intra-mutational heterogeneity is heavily prognostic and allows more personalized results.
3.3. Combining Traditional and Mutational Models
Despite these challenges, mutational data is proving to be instrumental in improving upon established scoring systems. A model comprised solely of mutational data has achieved similar accuracy to IPSS-R and, more tellingly, combining some of those mutations with traditional clinical features has yielded prognostic abilities that surpassed IPSS-R [11]. Another study used IPSS-R score combined with age (which is not directly assessed by IPSS-R) and mutational data from EZH2, SF3B1, and TP53, and succeeded in surpassing the accuracy of IPSS-R alone when applied to the same cohort of 508 patients. Similarly, adding age and these same mutational features to the other major classifying schemes uniformly resulted in increased accuracy, and in the case of IPSS-R, reassignment of 26% of patients in the low-risk cohort to the high-risk cohort.
4. FUTURE CONSIDERATIONS—TOWARD PERSONAL PROGNOSTICATION
Next generation DNA sequencing has identified a significant number of mutations implicated in MDS prognosis, and the breadth of MDS features available for analysis, both patient-related and disease-related, has now quickly surpassed the limitations of traditional scoring systems. The unwieldy interactions within this multitude of features argues for novel approaches when designing the latest iteration of prognostic models. The path forward is best informed by critical analysis of current prognostic models, and by identifying guiding mechanisms that likely need to be considered to allow for a new generation of prognostic accuracy.
4.1. Handling Complexity
As the pool of features associated with MDS outcomes grows, the task of choosing which features to include in scoring system creation based on expert opinion becomes unsurmountable, particularly when considering that such features have dramatic and unpredictable interactions with other feature states. For example, possessing a mutated TP53 gene is associated with worse outcomes in many malignancies, MDS included. This may be inferred by expert opinion and would therefore likely be considered for model creation. However, the presence of a CK highly modulates the effect of a TP53 mutation, decreasing survival from 73% of patients alive at 60 months with TP53-only phenotype to less than 20% alive within 2 years if TP53 is in combination with CK [27]. Such interactions are unreliably predicted in best-case scenarios with known genes, and completely unknown for the majority of new genomic features that are now under scrutiny. Because the next generation of prognostic scoring systems will need to incorporate large-scale genomic data, as well as all the previously described patient and disease factors, unsupervised computational grouping and relevance determination will be required to account for unknown or inexplicable feature interactions, given the complexity of the system. Machine learning, an application of artificial intelligence, is particularly suited for this task. Machine learning-powered MDS prognostication is already surpassing traditional models such as the IPSS-R [30,31] (Table 1). Based on the breadth of support and development of machine learning algorithms throughout the scientific community, the continued improvement of this computational method and its ability to refine MDS OS prediction is assured.
Scoring System | Model Features | c-index |
---|---|---|
International Prognostic Scoring System (IPSS) | Karyotype categories [3], simple BM blasts % Cytopenias |
0.65 |
Revised International Prognostic Scoring System (IPSS-R) | Karyotype categories [5], complex BM blasts % Hgb Platelets ANC |
0.67 |
MD Anderson Cancer Center (MDACC) | Karyotype categories [2], simple BM blasts % Hgb Platelets WBC Age Performance status Prior transfusion |
0.65 |
World Health Organization-Based Prognostic Scoring System (WPSS) | Karyotype categories [3], simple WHO category Transfusion requirement |
0.65 |
Nazha et al. Geno-clinical model [13] | Cytogenetic categories of IPSS-R [5], complex BM blasts % Hgb Platelets WBC Age WHO category Secondary vs de novo MDS TP53, RUNX1, ANC, STAG2, SRSF2, NPM1, PHF6, IDH1, EZH2, and SF3B1 |
0.71 |
A summary of model features utilized in five MDS prognostic scoring systems, and the resulting c-index of each.
4.2. Universal Applicability
As previously described, most major MDS scoring systems were originally designed using data from de novo patients and later extrapolated to patients with secondary MDS, treatment failure, and other disease states, with variable success. To create a prognostic algorithm that can model OS when implemented at various times throughout the disease course, the initial training dataset should include features collected from patients from each of these disease phases. Beginning with a multitude of patient presentations will allow unsupervised training to adjust the model to accommodate each permutation of MDS-associated illness, such as de novo patients, secondary or therapy-related MDS, those who have failed HMA or HCT therapy, etc. Because modeling with machine learning improves its accuracy with additional data points, accumulating a large and diverse pool of MDS patients is needed to produce clinically relevant predictions.
4.3. Clinical Interpretability
After the total pool of MDS features is evaluated by machine learning and a best-fit model is produced, iterative feature reduction is necessary to distill a frequently overwhelming number of quasi-significant features to the least number possible, while preserving the predictive power of the model. This can be accomplished by determining the relative weight of each feature and culling the least-weighted features followed by repeat modeling. Reducing the feature burden after model creation disposes of those variables least likely to contribute to OS, and simplifies future data acquisition via a reduction in features necessary for a patient to be included in the total pool of MDS data (allowing for primary data growth and subsequent model refinement). Importantly, it also allows for a more clinically relevant end model. An advantage of traditional scoring systems is the degree of interpretability inherent to each system's design: the features assessed were mostly obtained by expert opinion or known disease correlates, such as BM blast percentage or degree of cytopenias. In addition to being statistically validated, traditional scoring systems benefit from simple design with few components, all of which put clinicians at ease because each prediction can be questioned, if deemed necessary. The “black box” nature of machine learning models remove this failsafe and instill distrust, regardless of statistical methodology. To overcome this barrier and allow for clinician adoption of a model, feature explanation/weighting analysis should be made available for any given prediction, which is a programmatic feat within the scope of modern machine learning today. These explanatory tools can take the best available model, already bereft of minimally contributing features due to iterative feature reduction, and produce patient-specific outcome predictions that are fully annotated with descriptions and relative weights to ensure maximum interpretability.
5. CONCLUSIONS
Using prognostic scoring systems to inform clinical expectations and treatment paths has long been essential to MDS management, with the most commonly used systems today dating back to 1997. Over the past two decades, retrospective observation has identified numerous areas of potential prognostic improvement, including dynamicity to accommodate various stages of MDS (both at presentation and during or after treatment), relevance for secondary or therapy-related MDS, and more concise delineation of high- and low-risk patients rather than using suboptimal intermediate groups. Over the same time period, the advent of next generation sequencing has potentiated an influx of novel prognostic factors that are now starting to appear in clinically relevant scoring systems. Similarly, emerging machine learning strategies can now replace expert opinion and informed guessing when first deciding which factors to evaluate for statistical relevance, leading to more accurate scoring models. MDS is best treated based on decisive and accurate risk stratification for aggressive progression while early in the disease course. By assessing new genetic markers along with traditional clinical factors via machine learning, a new standard of prognostication may be possible.
6. PRACTICAL POINTS
Individualized prognosis at the time of AML diagnosis has significant influence over treatment decisions and patient expectations.
The most commonly used prognostic scoring system in the clinic today is the revised IPSS, which relies on standard patient-related and disease-related variables, including cytogenetics, and also includes a small subset of gene mutations.
Recent data suggest that greater prognostic accuracy can be achieved through the inclusion of additional gene mutations and the consideration of complex, difficult to predict variable interactions.
Contemporary genetic and clinical prognostic models have now been found to outperform the revised IPSS, but are still experimental and not yet widely adopted.
7. RESEARCH AGENDA
As genetic and clinical prognostic models devised using machine learning overtake current clinical standards, several considerations pertaining to research involving artificial intelligence need to be addressed.
These models are able to determine meaningful associations amidst the complexity of MDS feature interactions, but improving each model through the optimization of different machine learning algorithms, training parameters, and feature reduction is still underway.
More patient data are needed to generate better-fit models with wider applicability, such as for re-prognostication after treatment failure or for individuals with secondary or therapy-related MDS.
Additional avenues of model interpretability are needed to improve clinical relevance and to assuage the practitioners' fear of the “black box.”
CONFLICT OF INTEREST
Authors disclose no conflict of interest of this work.
AUTHORS' CONTRIBUTIONS
Both authors contributed equally to the conceptualization and execution of this review.
ACKNOWLEDGMENTS
We appreciate the academic support of the Department of Internal Medicine, the Taussig Cancer Center, and Cleveland Clinic at large for enabling this review.
REFERENCES
Cite this article
TY - JOUR AU - Jacob Shreve AU - Aziz Nazha PY - 2020 DA - 2020/04/19 TI - The Evolving Landscape of Myelodysplastic Syndrome Prognostication JO - Clinical Hematology International SP - 43 EP - 48 VL - 2 IS - 2 SN - 2590-0048 UR - https://doi.org/10.2991/chi.d.200408.001 DO - 10.2991/chi.d.200408.001 ID - Shreve2020 ER -